Canonical Voice Conversion and Dual-Channel Processing for Improved Voice Privacy of Speech Recognition Data
2023 31st European Signal Processing Conference (EUSIPCO)(2023)
摘要
This paper addresses the need for enhancing the privacy of test data in a deployed automatic speech recognition (ASR) system so that what was said cannot be linked to who said it, a process we describe as acoustic de-identification. Existing techniques can be used to modify voice characteristics to make the speaker identity unrecognizable, but normally at the expense of ASR performance. We present a novel approach for improving ASR performance on acoustically de-identified voice data. Our method exploits a dual-channel input to a self-attention channel combinator front-end to an end-to-end ASR system, and data augmentation, where some amount of original speech data is used in model training. The voice data is de-identified by a zero-shot voice style transfer system to the voice of a registered, canonical speaker. We show that the proposed approach achieves a significant improvement in privacy as demonstrated by a 10x increase in the EER of an automatic speaker verification system, while also improving the ASR accuracy as demonstrated by a 18.3% reduction in WER relative to a single channel model baseline model when tested on acoustically de-identified speech.
更多查看译文
AI 理解论文
溯源树
样例
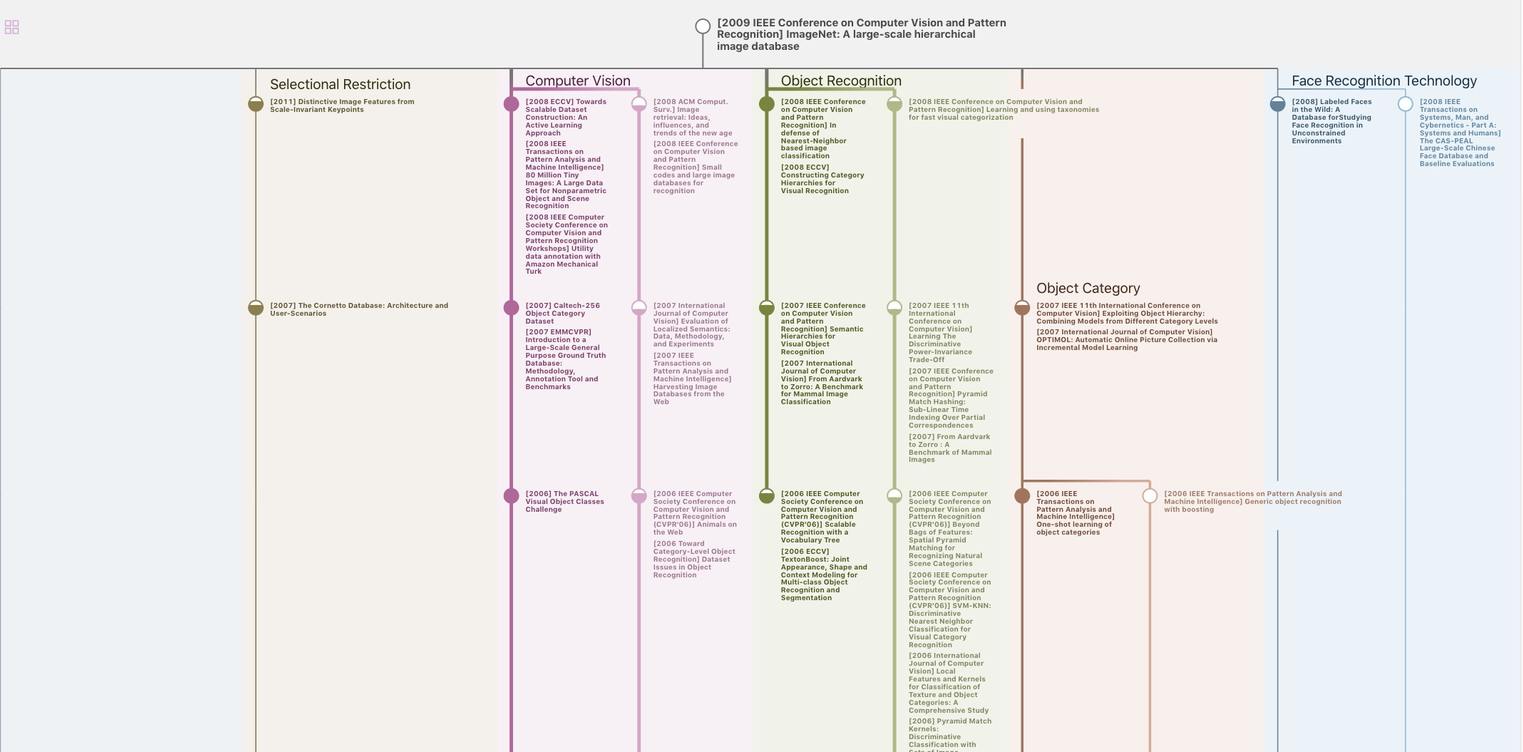
生成溯源树,研究论文发展脉络
Chat Paper
正在生成论文摘要