Data-Driven Non-Intrusive Speech Intelligibility Prediction Using Speech Presence Probability
IEEE-ACM TRANSACTIONS ON AUDIO SPEECH AND LANGUAGE PROCESSING(2024)
摘要
Time consuming Speech Intelligibility (SI) listening tests with human subjects can be replaced by algorithmic SI predictors. In recent years, data-driven SI predictors have been showing promising results. A major limiting factor in the advancement of data-driven SI prediction is that there is a scarcity of SI listening test data available to train the data-driven methods. In this article we propose a data-driven SI predictor that does not require access to an underlying noise-free reference signal, i.e., non-intrusive, and which does not require listening test data for training. Instead, the proposed method exploits a hypothesized link between SI and Speech Presence Probability (SPP). We show that a neural network can be trained on easily obtainable speech in additive noise data to estimate SPP, and that a simple post-processing stage can be applied in order to map the estimated SPP to SI predictions with high accuracy. The proposed method is evaluated and compared to other state-of-the art non-intrusive SI predictors, and achieves the highest performance even in the presence of processed noisy speech, which the SPP estimator has not been trained on.
更多查看译文
关键词
Speech intelligibility prediction,speech presence probability
AI 理解论文
溯源树
样例
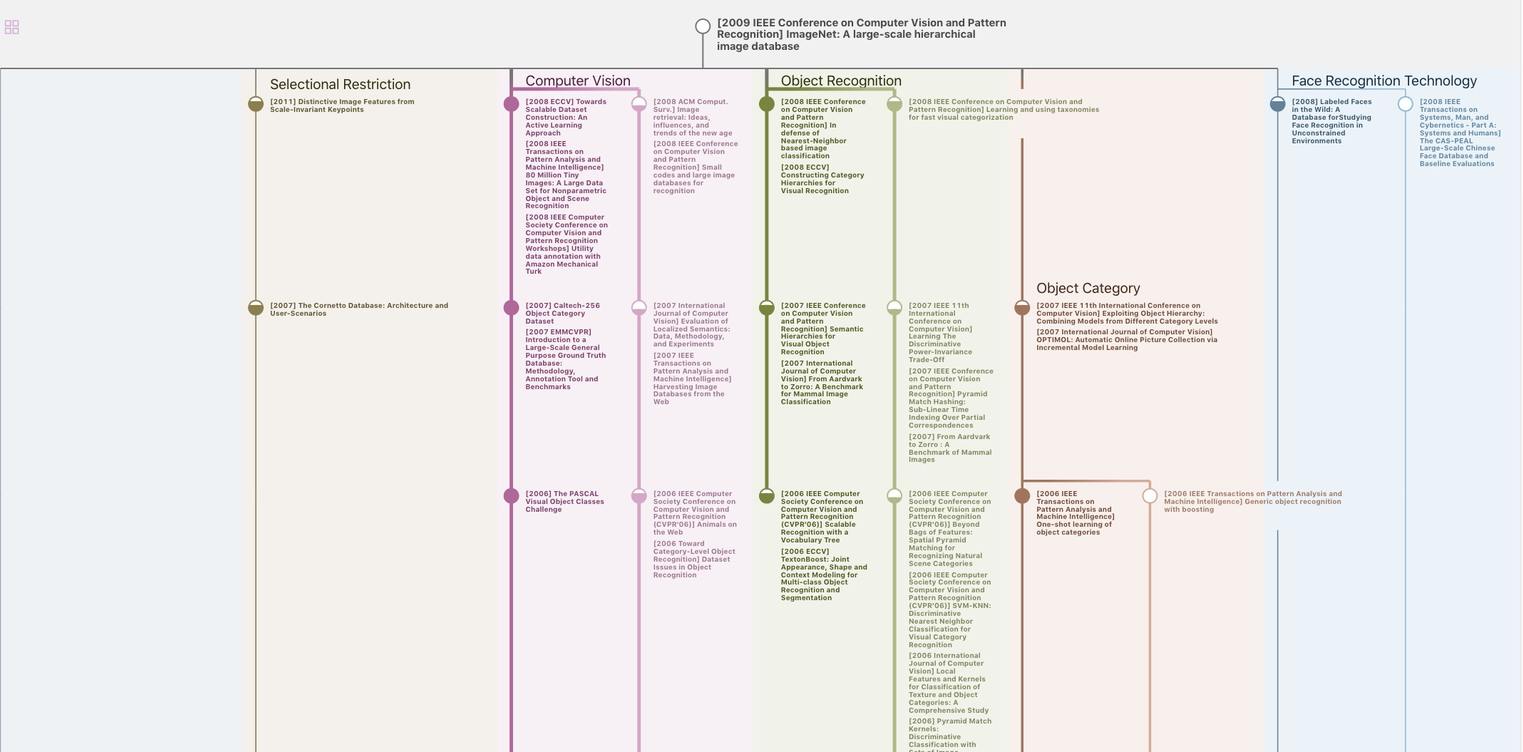
生成溯源树,研究论文发展脉络
Chat Paper
正在生成论文摘要