Multi-Cue Guided Semi-Supervised Learning Toward Target Speaker Separation in Real Environments
IEEE-ACM TRANSACTIONS ON AUDIO SPEECH AND LANGUAGE PROCESSING(2024)
摘要
To solve the cocktail party problem in real multi-talker environments, this article proposed a multi-cue guided semi-supervised target speaker separation method (MuSS). Our MuSS integrates three target speaker-related cues, including spatial, visual, and voiceprint cues. Under the guidance of the cues, the target speaker is separated into a predefined output channel, and the interfering sources are separated into other output channels with the optimal permutation. Both synthetic mixtures and real mixtures are utilized for semi-supervised training. Specifically, for synthetic mixtures, the separated target source and other separated interfering sources are trained to reconstruct the ground-truth references, while for real mixtures, the mixture of two real mixtures is fed into our separation model, and the separated sources are remixed to reconstruct the two real mixtures. Besides, in order to facilitate finetuning and evaluating the estimated source on real mixtures, we introduce a real multi-modal speech separation dataset, RealMuSS, which is collected in real-world scenarios and is comprised of more than one hundred hours of multi-talker mixtures with high-quality pseudo references of the target speakers. Experimental results show that the pseudo references effectively improve the finetuning efficiency and enable the model to successfully learn and evaluate estimating speech on real mixtures, and various cue-driven separation models are greatly improved in signal-to-noise ratio and speech recognition accuracy under our semi-supervised learning framework.
更多查看译文
关键词
Cocktail party problem,target speaker separation,multi-cue guided separation,semi-supervised learning
AI 理解论文
溯源树
样例
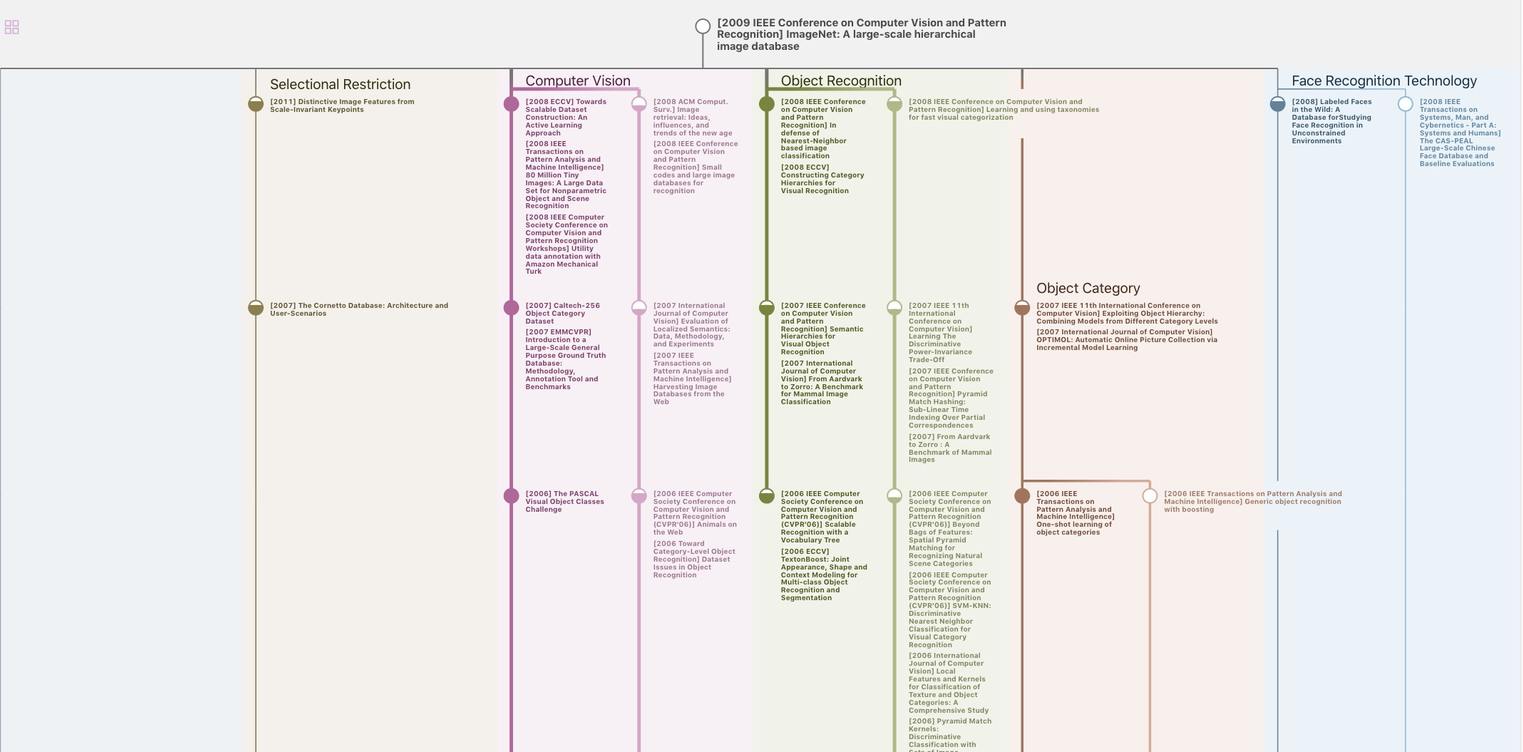
生成溯源树,研究论文发展脉络
Chat Paper
正在生成论文摘要