Attention Alignment and Flexible Positional Embeddings Improve Transformer Length Extrapolation.
CoRR(2023)
摘要
An ideal length-extrapolatable Transformer language model can handle sequences longer than the training length without any long sequence fine-tuning. Such long-context utilization capability highly relies on a flexible positional embedding design. Upon investigating the flexibility of existing large pre-trained Transformer language models, we find that the T5 family deserves a closer look, as its positional embeddings capture rich and flexible attention patterns. However, T5 suffers from the dispersed attention issue: the longer the input sequence, the flatter the attention distribution. To alleviate the issue, we propose two attention alignment strategies via temperature scaling. Our findings improve the long-context utilization capability of T5 on language modeling, retrieval, and multi-document question answering without any fine-tuning, suggesting that a flexible positional embedding design and attention alignment go a long way toward Transformer length extrapolation.\footnote{\url{https://github.com/chijames/Attention-Alignment-Transformer-Length-Extrapolation}}
更多查看译文
AI 理解论文
溯源树
样例
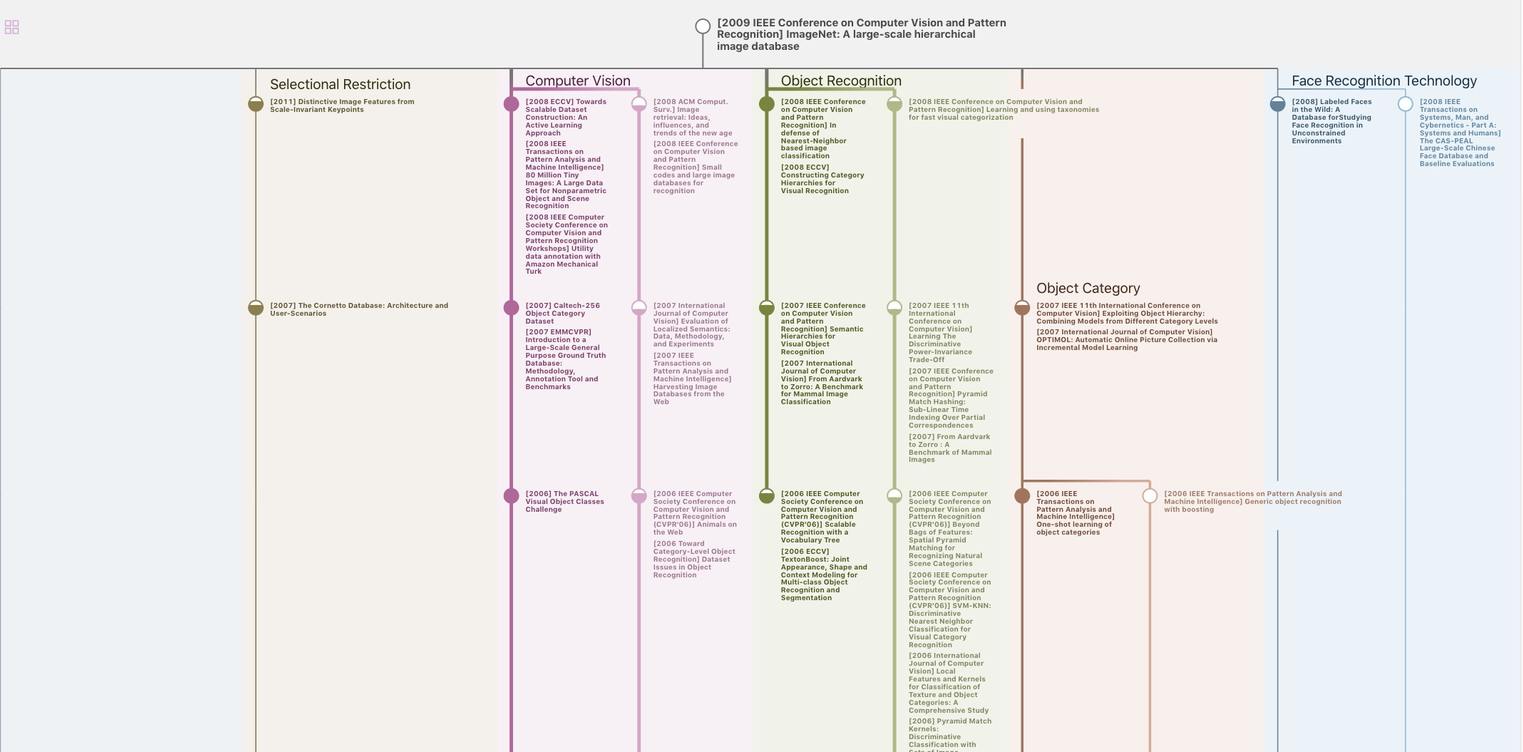
生成溯源树,研究论文发展脉络
Chat Paper
正在生成论文摘要