Echo-evolution data generation for quantum error mitigation via neural networks
arXiv (Cornell University)(2023)
摘要
Neural networks provide a prospective tool for error mitigation in quantum simulation of physical systems. However, we need both noisy and noise-free data to train neural networks to mitigate errors in quantum computing results. Here, we propose a physics-motivated method to generate training data for quantum error mitigation via neural networks, which does not require classical simulation and target circuit simplification. In particular, we propose to use the echo evolution of a quantum system to collect noisy and noise-free data for training a neural network. Under this method, the initial state evolves forward and backward in time, returning to the initial state at the end of evolution. When run on the noisy quantum processor, the resulting state will be influenced by with quantum noise accumulated during evolution. Having a vector of observable values of the initial (noise-free) state and the resulting (noisy) state allows us to compose training data for a neural network. We demonstrate that a feed-forward fully connected neural network trained on echo-evolution-generated data can correct results of forward-in-time evolution. Our findings can enhance the application of neural networks to error mitigation in quantum computing.
更多查看译文
关键词
quantum error mitigation,neural
AI 理解论文
溯源树
样例
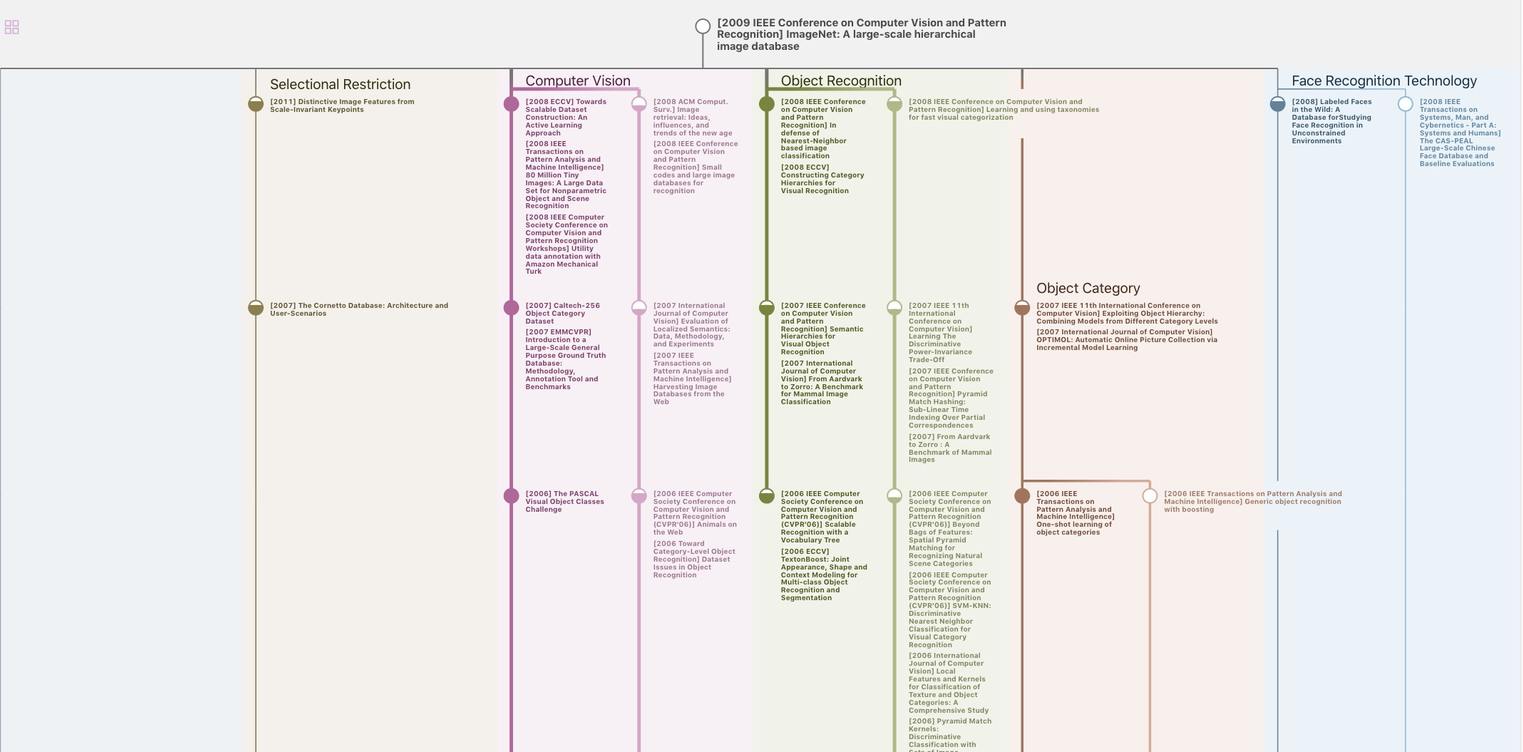
生成溯源树,研究论文发展脉络
Chat Paper
正在生成论文摘要