Transferable wind power probabilistic forecasting based on multi-domain adversarial networks
ENERGY(2023)
摘要
Due to the limited availability of historical data, forecasting the wind power of newly-built wind farms poses a significant challenge. Transfer learning methods offer a potential solution by transferring knowledge from the source domain to the target domain, thereby reducing the data requirements for wind power forecasting. However, the difference in dataset distribution between the source and target domains causes domain shift. To address this issue, we propose a multi-domain adversarial network (MDAN). MDAN uses multi-domain datasets for adversarial learning, which maps numerical weather prediction (NWP) data to an adaptive latent space, thereby reducing domain shift. Additionally, we propose a data fusion-based incremental learning method to mitigate catastrophic forgetting. Through comprehensive case studies, MDAN provides accurate short-term wind power probabilistic forecasts in zero-shot learning. The incremental learning method enhances forecast accuracy in few-shot learning. Moreover, visualization analysis using t-stochastic neighbor embedding (t-SNE) shows that MDAN successfully reduces the domain shift between the source and target domains.
更多查看译文
关键词
Transfer learning,Wind power,Probabilistic forecasting,Domain adaption,Incremental learning
AI 理解论文
溯源树
样例
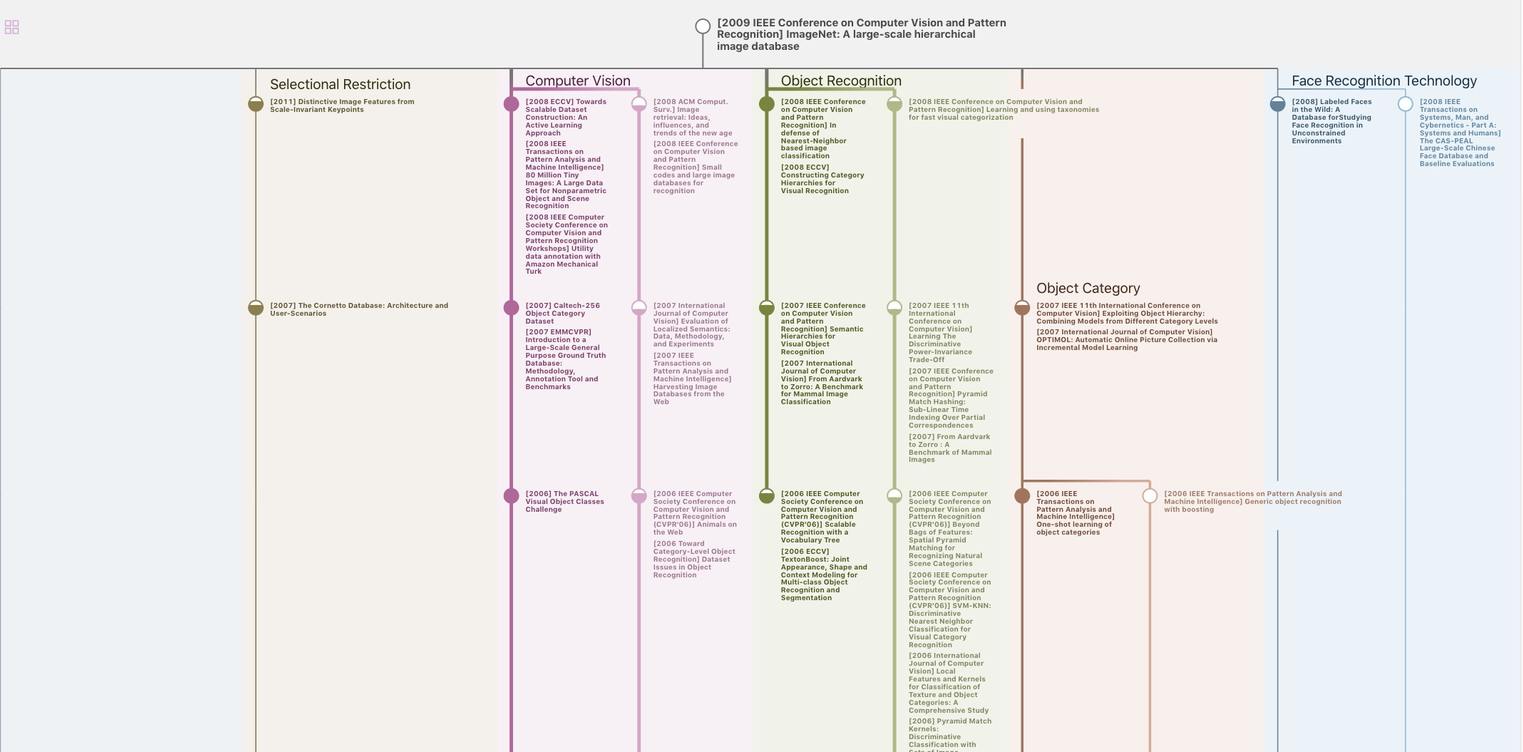
生成溯源树,研究论文发展脉络
Chat Paper
正在生成论文摘要