Selective Kernel Fusion Complex-Valued CNN for Modulation Recognition
2023 IEEE 34TH ANNUAL INTERNATIONAL SYMPOSIUM ON PERSONAL, INDOOR AND MOBILE RADIO COMMUNICATIONS, PIMRC(2023)
Abstract
Automatic modulation recognition (AMR) plays an essential role in intelligent communication networks monitoring, management, and optimization. Recently, it has been shown that deep learning-based methods perform well in AMR. However, most existing methods are based on real-valued networks, e.g., convolution neural network (CNN), which are not specifically designed for AMR. Thus, the recognition performance is limited. In this paper, we propose a selective kernel fusion complex-valued convolution neural network (SKF-CCNN) for the fulfillment of the AMR task. The proposed method uses parallel complex-valued convolution for raw in-phase/quadrature (I/Q) sequence together with real-valued convolution for amplitude. The complex-valued features and amplitude features are then fused by a selective kernel block to combine all sorts of information. Lastly, a ResNeXt block and two convolution layers are employed to extract further information from the fused feature map for the task of the final classification. Experiments on the benchmark dataset show that the proposed method outperforms the existing complex-valued methods, especially at high SNRs.
MoreTranslated text
Key words
Automatic modulation recognition,complex-valued convolution neural network,deep learning,selective kernel network
AI Read Science
Must-Reading Tree
Example
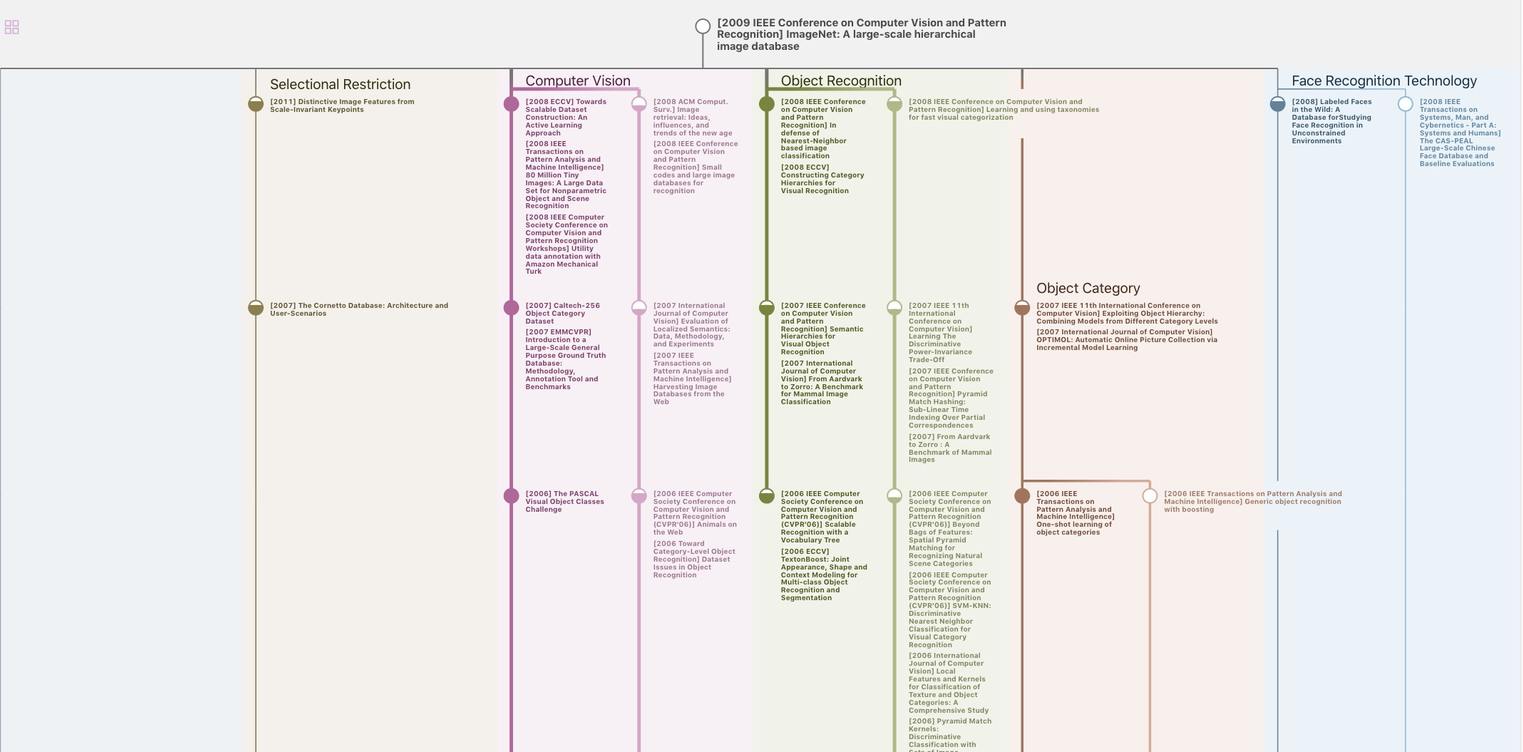
Generate MRT to find the research sequence of this paper
Chat Paper
Summary is being generated by the instructions you defined