ConVERTS: Contrastively Learning Structurally InVariant Netlist Representations
2023 ACM/IEEE 5th Workshop on Machine Learning for CAD (MLCAD)(2023)
摘要
Graph neural network (GNN)-based representations of hardware designs are used in electronic design automation (EDA) tasks like logic synthesis, verification, and hardware security. While promising, state-of-the-art methods are supervised and require target labels and/or need different behavioral register transfer level (RTL) codes of the same function as training data to generalize. We propose ConVERTS, a self-supervised netlist contrastive learning method that generalizes well using one-shot RTL of a design. We demonstrate the effectiveness of ConVERTS on two use-cases: (1) netlist classification, and (2) Recovering functionality of obfuscated designs.
更多查看译文
AI 理解论文
溯源树
样例
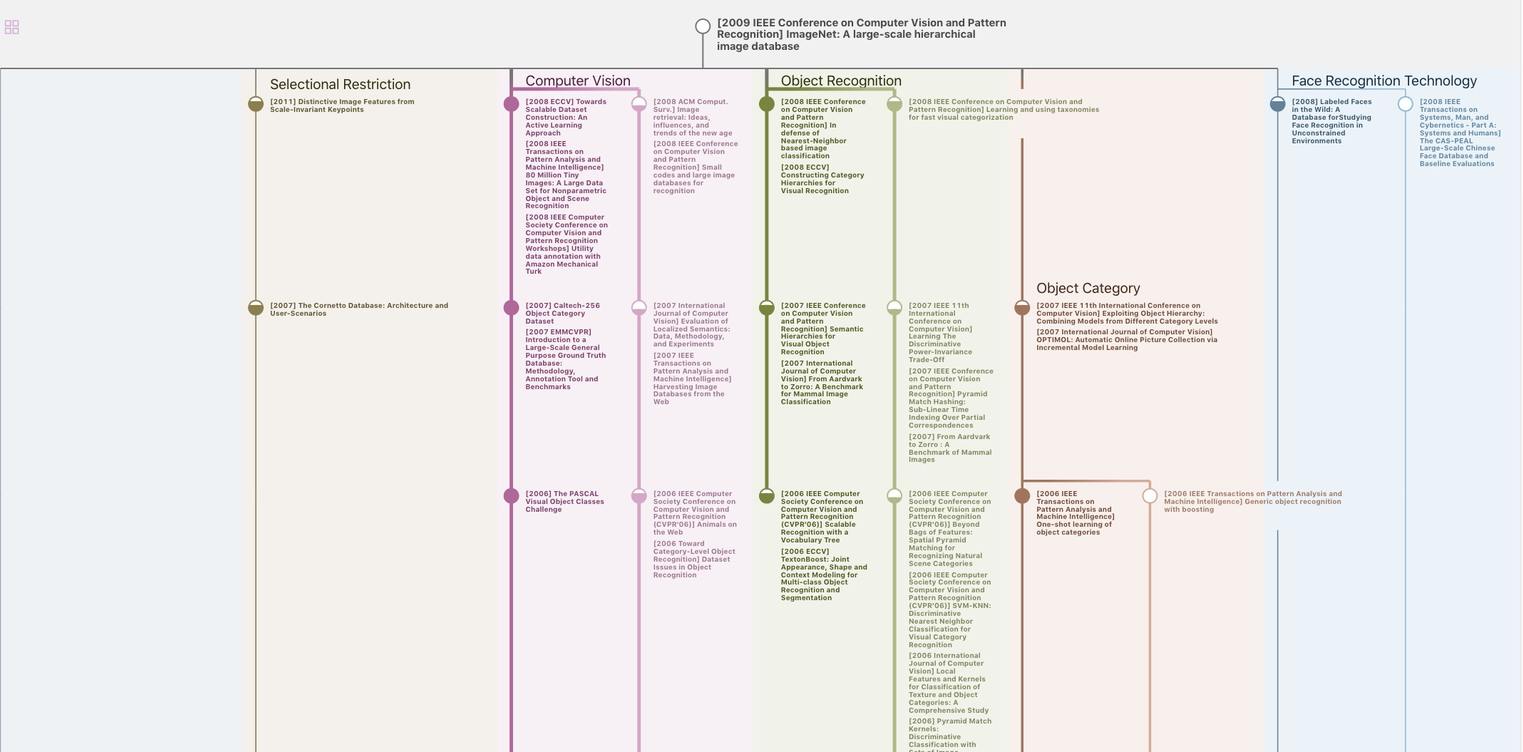
生成溯源树,研究论文发展脉络
Chat Paper
正在生成论文摘要