The 2023 MLCAD FPGA Macro Placement Benchmark Design Suite and Contest Results
2023 ACM/IEEE 5th Workshop on Machine Learning for CAD (MLCAD)(2023)
摘要
The 2023 IEEE/ACM MLCAD Workshop program included the inaugural MLCAD Contest, a multi-month research and development competition intended to focus attention on pressing challenges at the nexus of electronic design automation (EDA) and machine learning. The 2023 contest aimed to spur research into machine learning-driven solutions that can supplant state-of-the-art algorithms for FPGA macro placement. Several factors make this problem more challenging than its ASIC counterpart. These include: (i) discrete and SITE-typed columnated nature of the FPGA device layout; (ii) cascaded macros or carry chains that can greatly impact routability and timing closure because of their high pin count and net connectivities, (iii) prevalence of resource-bound multi-clock domains; (iv) larger number of macros (typically 100s–1000s) than in ASICs; and (v) fragmentation of placement across multiple dies. Two benchmark suites containing 140 public and 198 hidden designs with varying complexities (based on Rent exponent, LUT/FF/BRAM/DSP utilization, carry chains, cascaded macro shapes and number of clock domains) were created for this competition. 19 teams globally participated in the contest. We share the results from the 8 finalists, 6 of which had competitive solutions. Our goal was for the contest to draw international participation and catalyze industry-academic collaborations, leading to contributions in premier conferences and journals. Several contestants used electrostatic-based frameworks (e.g., [13], DreamPlaceFPGA [29] and OpenPARF [24]) for global placement solution evaluations, alongside core macro placement algorithms based on classical optimization approaches (e.g., min-cost flow, bipartite graph matching, and simulated annealing). One team used an RL-parameterized simulated annealing algorithm for their macro placement solution. The contributed solutions are insightful, and we remain optimistic about the contest’s potential lasting influence for developing better algorithms for FPGA macro placement.
更多查看译文
关键词
CAD Contest,FPGA,macro placement,physical design,electronic design automation,computer-aided design,integrated circuits,MLCAD
AI 理解论文
溯源树
样例
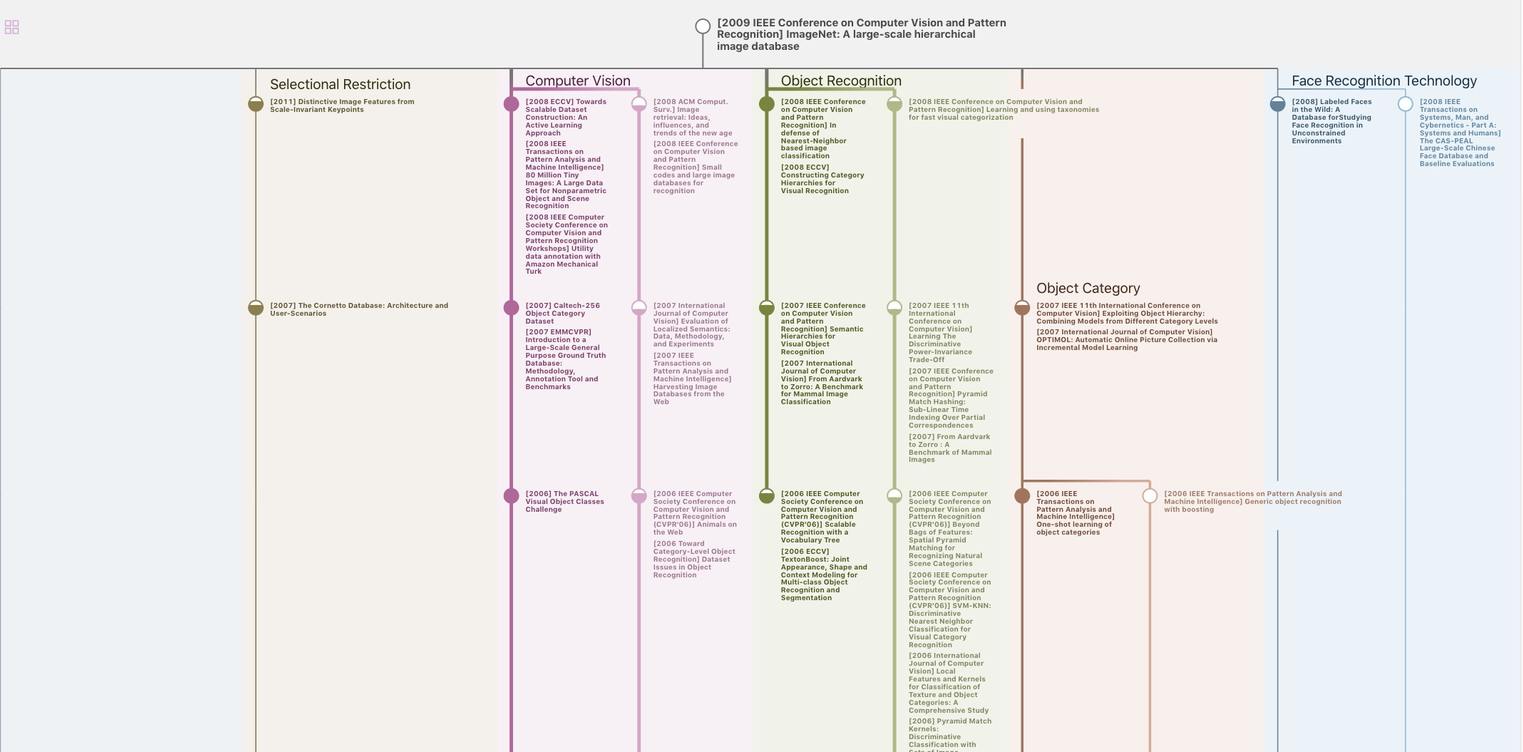
生成溯源树,研究论文发展脉络
Chat Paper
正在生成论文摘要