Machine Learning Based Rain Gauge Using Acoustic Data
2023 IEEE AFRICON(2023)
Abstract
Weather stations provide data used in planning and forecasts such as water management, precision agriculture, and flood prediction. According to the World Meteorological Organization, Africa has about one-eighth of the required number of weather stations. This is attributed to the high set-up costs, lack of skilled staff, and the fragile nature of instruments in conventional weather stations. Also, conventional weather stations have moving parts that make them prone to damage and cause both measurement and reading errors. This makes it difficult to get accurate real-time weather data measurements with good location specificity, resulting in inaccurate estimates. Recent research on using acoustic data for rainfall estimation through machine learning has been on classifying rainfall amounts into intensity bands rather than specifying the exact rainfall intensities. This research employs a CNN regression model to estimate rainfall intensities from MFCCs extracted from audio recordings collected in Nigeria. The study results show that the MAPE and MSE of the trained CNN model were 35.20% and 0.66 respectively. The experiment demonstrates that this CNN architecture performs better than a baseline Support Vector Regression (SVR) model with 152.55% MAPE and 1.73 MSE. The model was further pruned, reducing its size from 863kB to 300kb, making it suitable for deployment on low-cost microcontrollers with low memory. This makes it possible for large-scale deployments in low-resource settings, such as Africa.
MoreTranslated text
Key words
Rainfall,Weather Stations,Convolutional Neural Networks (CNN),Mel Frequency Cepstral Coefficients (MFCCs)
AI Read Science
Must-Reading Tree
Example
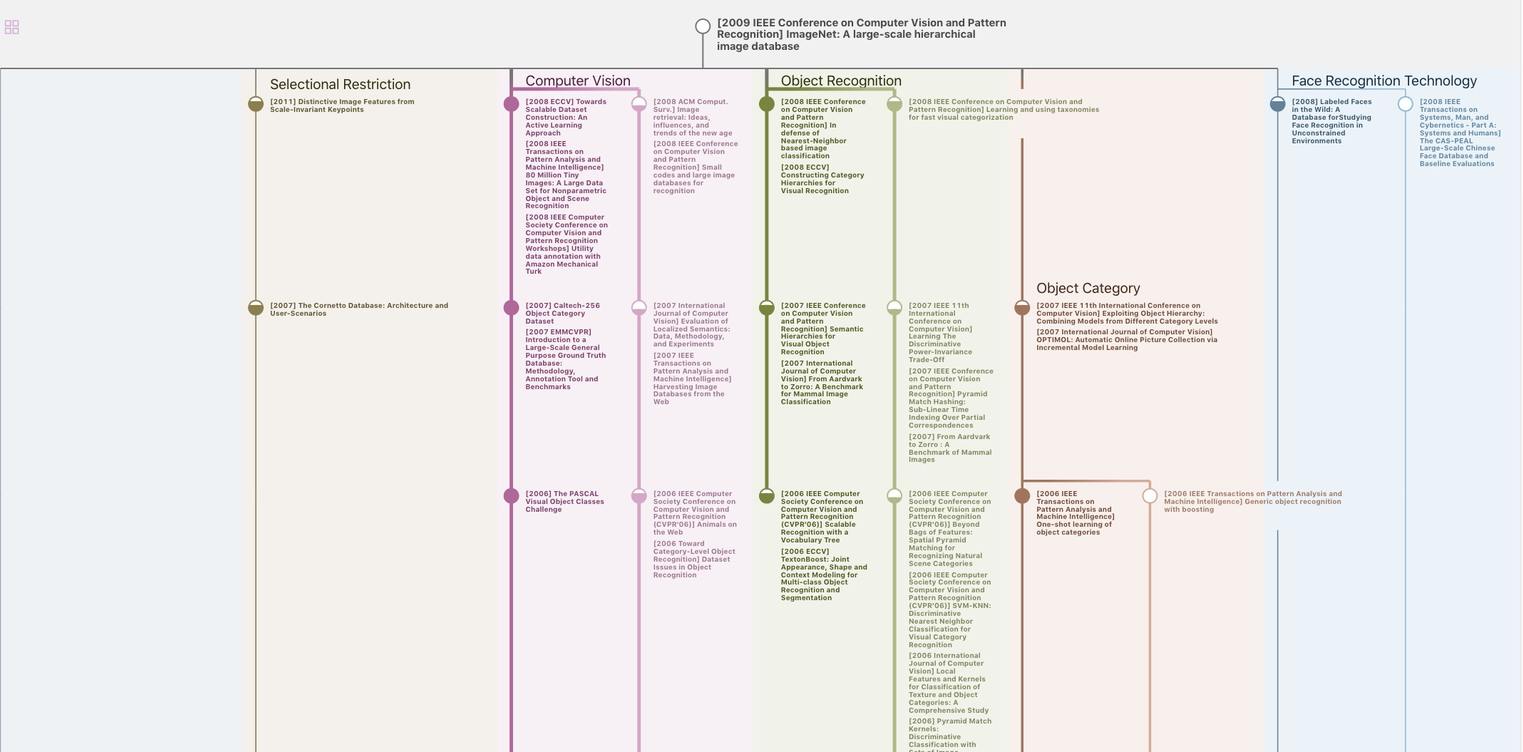
Generate MRT to find the research sequence of this paper
Chat Paper
Summary is being generated by the instructions you defined