Development of a physics-based method for calibration of low-cost particulate matter sensors and comparison with machine learning models
JOURNAL OF AEROSOL SCIENCE(2024)
摘要
Low cost particulate matter sensors are receiving significant attention as they can be used in large number for spatial and temporal measurement of PM mass and number concentration. However, the data reliability is questionable as these sensors are affected by numerous parameters such as temperature, relative humidity, and hygroscopicity of particles. To ensure accurate and reliable measurement of particulate matter concentrations, performance evaluation and calibration of LCS co-located with reference instrument at site is essential. In this study, the performance of the lowcost sensor (APT Maxima) was evaluated with the reference instrument SASS (Speciation Air Sampling System) sampler for developing suitable calibration factor that include impact of the meteorological parameter such as relative humidity and temperature, and hygroscopicity. In this study, we have demonstrated a systematic physics-based method for calibration of LCS based on kappa-Ko center dot hler theory and Mie theory. For comparison with statistical models, linear regression and machine learning algorithm were also applied. In physics-based model, calculated total light scattered intensity shows good linearity with reference PM2.5 measurements. Physics based model performed better for both the sites as compared to MLR, kNN, RF, and GB ML algorithms with R2, RMSE, and MAE values of 0.72,18.21, and 13.36 for Bhopal site, and 0.91, 7.84, and 5.76 for Kashmir site, respectively. Study indicates that physics-based approach for LCS calibration is suitable and can be transferable to different sites.
更多查看译文
关键词
Low-cost sensor,Particulate matter,Physics-based model,Sensor calibration
AI 理解论文
溯源树
样例
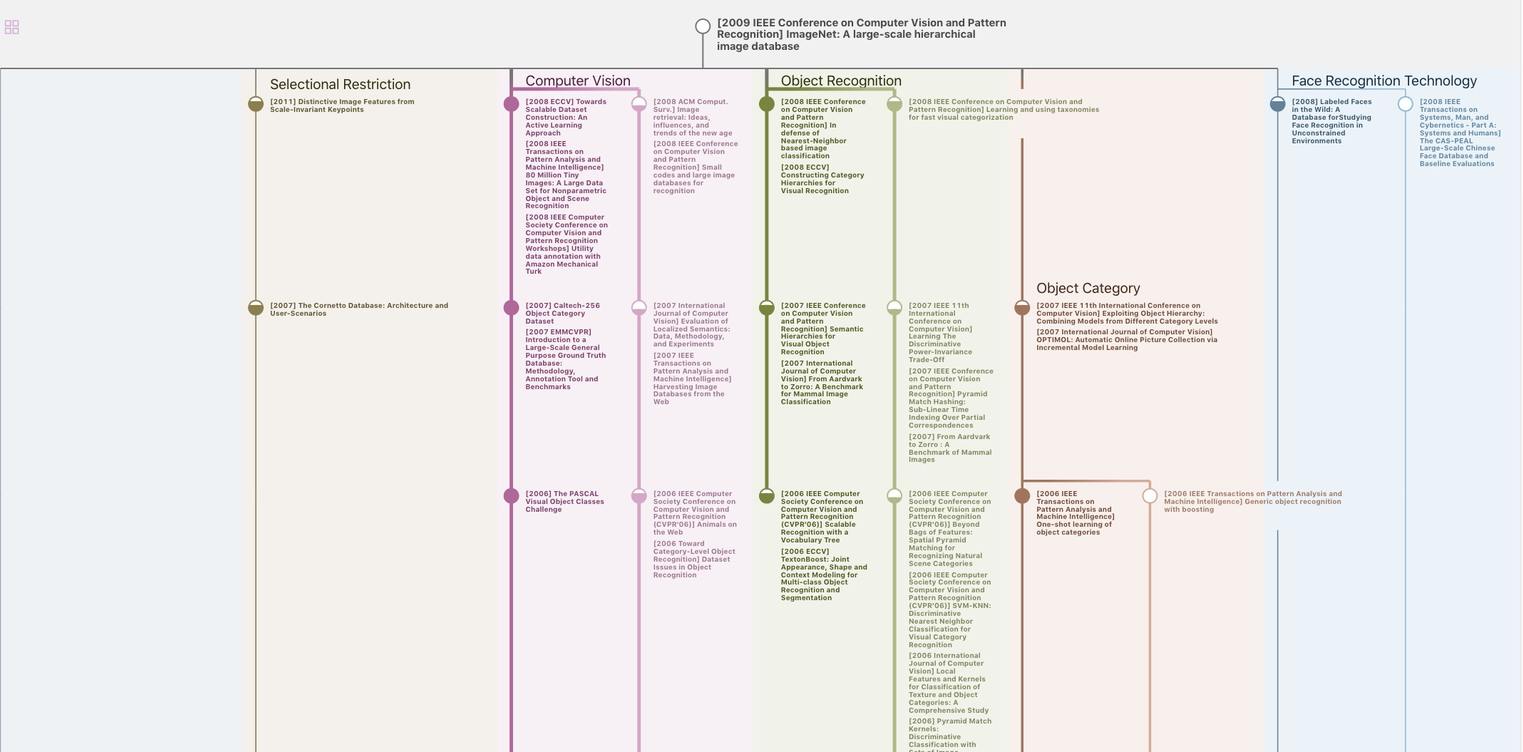
生成溯源树,研究论文发展脉络
Chat Paper
正在生成论文摘要