FlowgateUNet: Dental CT image segmentation network based on FlowFormer and gated attention
Signal, Image and Video Processing(2023)
摘要
Segmentation of cone-beam computed tomography (CBCT) images plays an important role in clinical treatment as well as teaching. Traditional manual segmentation of dental CBCT images requires tools such as mimics and is time-consuming. With the development of deep learning, the U-shaped network, represented by UNet, has shown good results. Due to the significant improvement brought by various applications of Transformers to image tasks, more and more models try to combine attention mechanism with traditional convolutional neural networks. To further improve the performance of dental CBCT segmentation, this paper proposes an improved FlowgateUNet segmentation network, which uses the FlowFormer instead of Transformer in the encoder to achieve attention computation with nearly linear complexity. It also uses the feature map containing global information as the gating signal in the skip connections to further extract relevant features and fuses the results from multiple decoders as the output. Compared to TransUnet, the proposed FlowgateUnet model improved the Dice similarity coefficient (DSC) by 1% on the dental CBCT image dataset, by 0.7% on the dental microCT dataset, and by 2% on the Synapse dataset.
更多查看译文
关键词
Medical image segmentation,Dental CBCT,Semantic segmentation,Attention mechanism
AI 理解论文
溯源树
样例
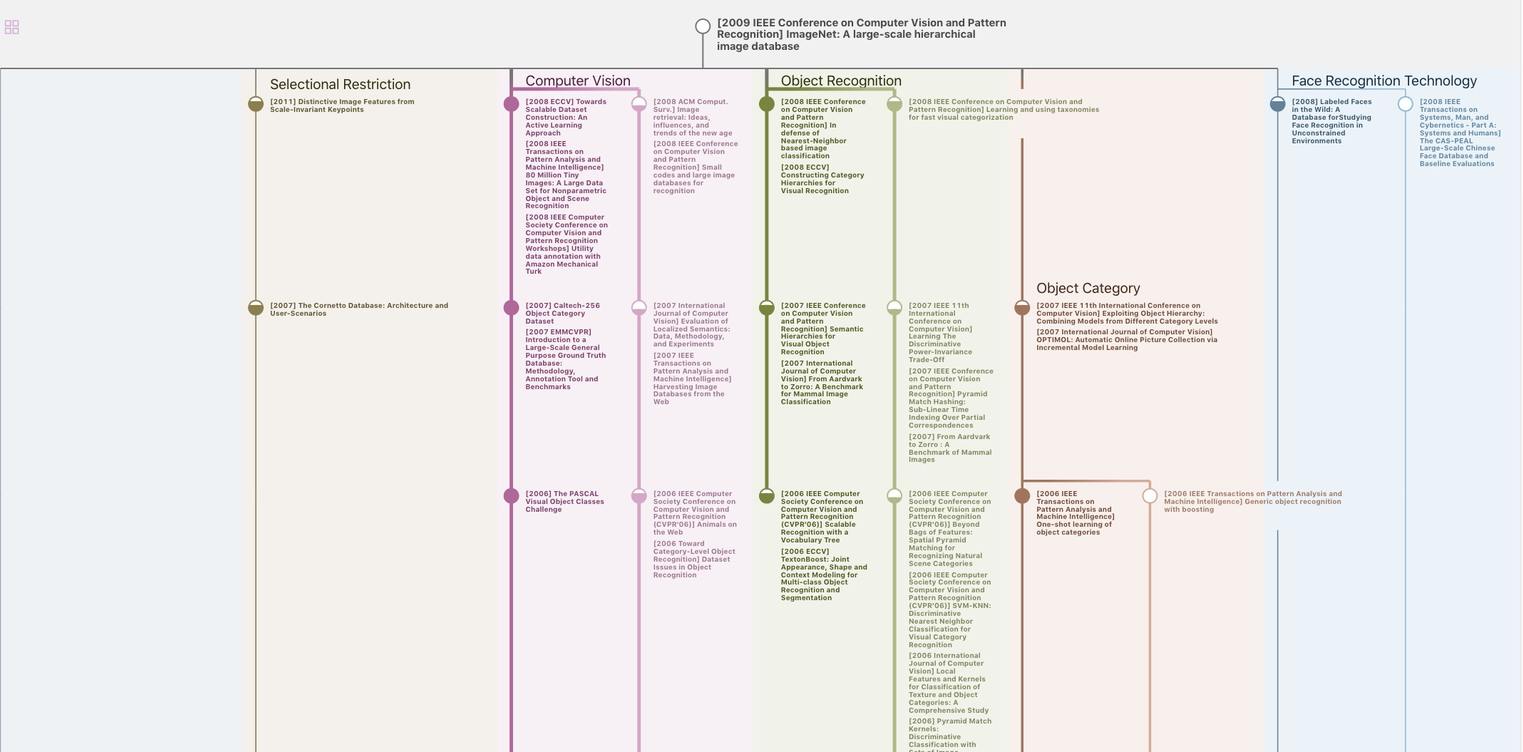
生成溯源树,研究论文发展脉络
Chat Paper
正在生成论文摘要