Intra-Modal Proxy Learning for Zero-Shot Visual Categorization with CLIP
NeurIPS(2023)
摘要
Vision-language pre-training methods, e.g., CLIP, demonstrate an impressive
zero-shot performance on visual categorizations with the class proxy from the
text embedding of the class name. However, the modality gap between the text
and vision space can result in a sub-optimal performance. We theoretically show
that the gap cannot be reduced sufficiently by minimizing the contrastive loss
in CLIP and the optimal proxy for vision tasks may reside only in the vision
space. Therefore, given unlabeled target vision data, we propose to learn the
vision proxy directly with the help from the text proxy for zero-shot transfer.
Moreover, according to our theoretical analysis, strategies are developed to
further refine the pseudo label obtained by the text proxy to facilitate the
intra-modal proxy learning (InMaP) for vision. Experiments on extensive
downstream tasks confirm the effectiveness and efficiency of our proposal.
Concretely, InMaP can obtain the vision proxy within one minute on a single GPU
while improving the zero-shot accuracy from $77.02\%$ to $80.21\%$ on ImageNet
with ViT-L/14@336 pre-trained by CLIP. Code is available at
\url{https://github.com/idstcv/InMaP}.
更多查看译文
关键词
categorization,learning,intra-modal,zero-shot
AI 理解论文
溯源树
样例
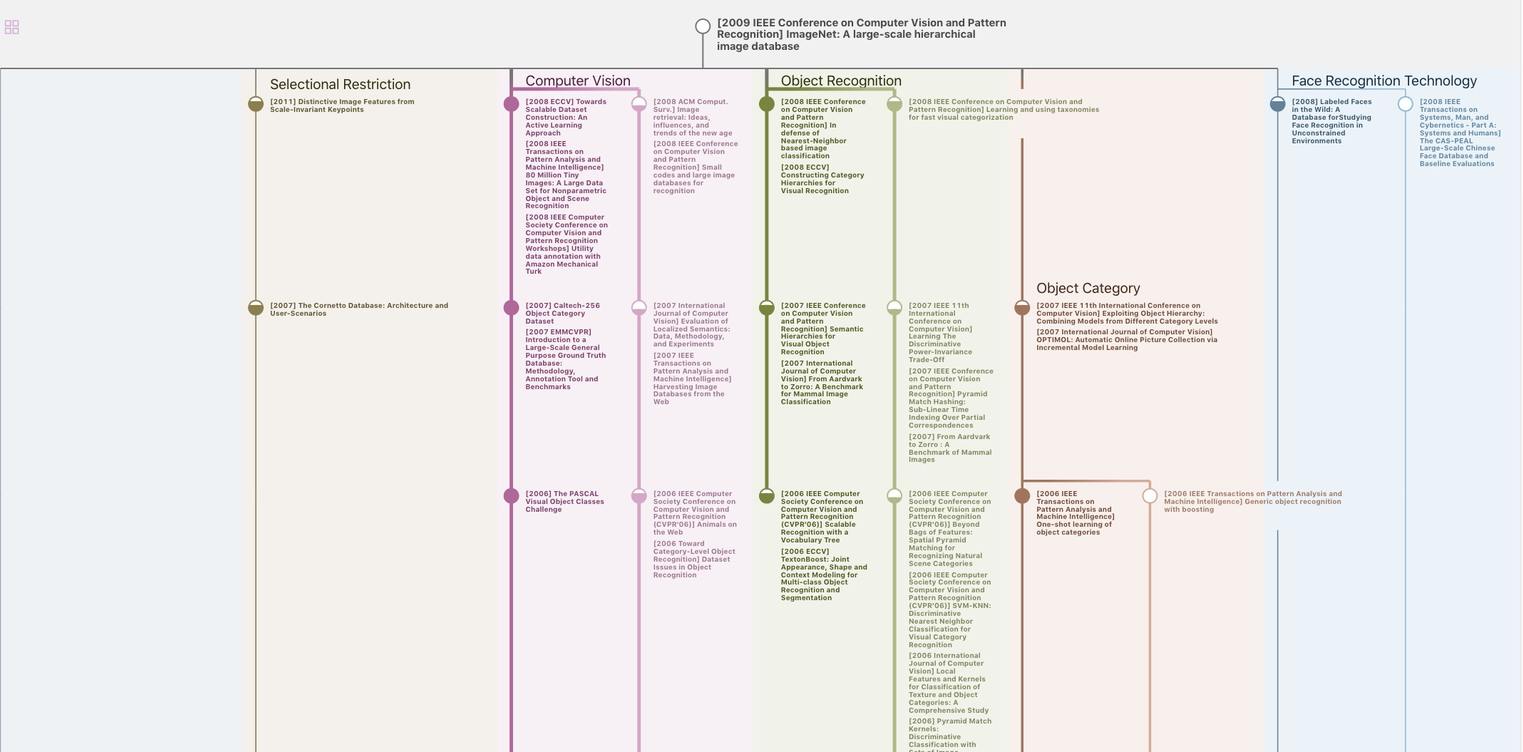
生成溯源树,研究论文发展脉络
Chat Paper
正在生成论文摘要