Real-World Implementation of Reinforcement Learning Based Energy Coordination for a Cluster of Households
PROCEEDINGS OF THE 10TH ACM INTERNATIONAL CONFERENCE ON SYSTEMS FOR ENERGY-EFFICIENT BUILDINGS, CITIES, AND TRANSPORTATION, BUILDSYS 2023(2023)
摘要
Given its substantial contribution of 40% to global power consumption, the built environment has received increasing attention to serve as a source of flexibility to assist the modern power grid. In that respect, previous research mainly focused on energy management of individual buildings. In contrast, in this paper, we focus on aggregated control of a set of residential buildings, to provide grid supporting services, that eventually should include ancillary services. In particular, we present a real-life pilot study that studies the effectiveness of reinforcement-learning (RL) in coordinating the power consumption of 8 residential buildings to jointly track a target power signal. Our RL approach relies solely on observed data from individual households and does not require any explicit building models or simulators, making it practical to implement and easy to scale. We show the feasibility of our proposed RL-based coordination strategy in a real-world setting. In a 4-week case study, we demonstrate a hierarchical control system, relying on an RL-based ranking system to select which households to activate flex assets from, and a real-time PI control-based power dispatch mechanism to control the selected assets. Our results demonstrate satisfactory power tracking, and the effectiveness of the RL-based ranks which are learnt in a purely data-driven manner.
更多查看译文
关键词
Demand Response,Reinforcement Learning,Building Cluster,Coordination,Advantage function
AI 理解论文
溯源树
样例
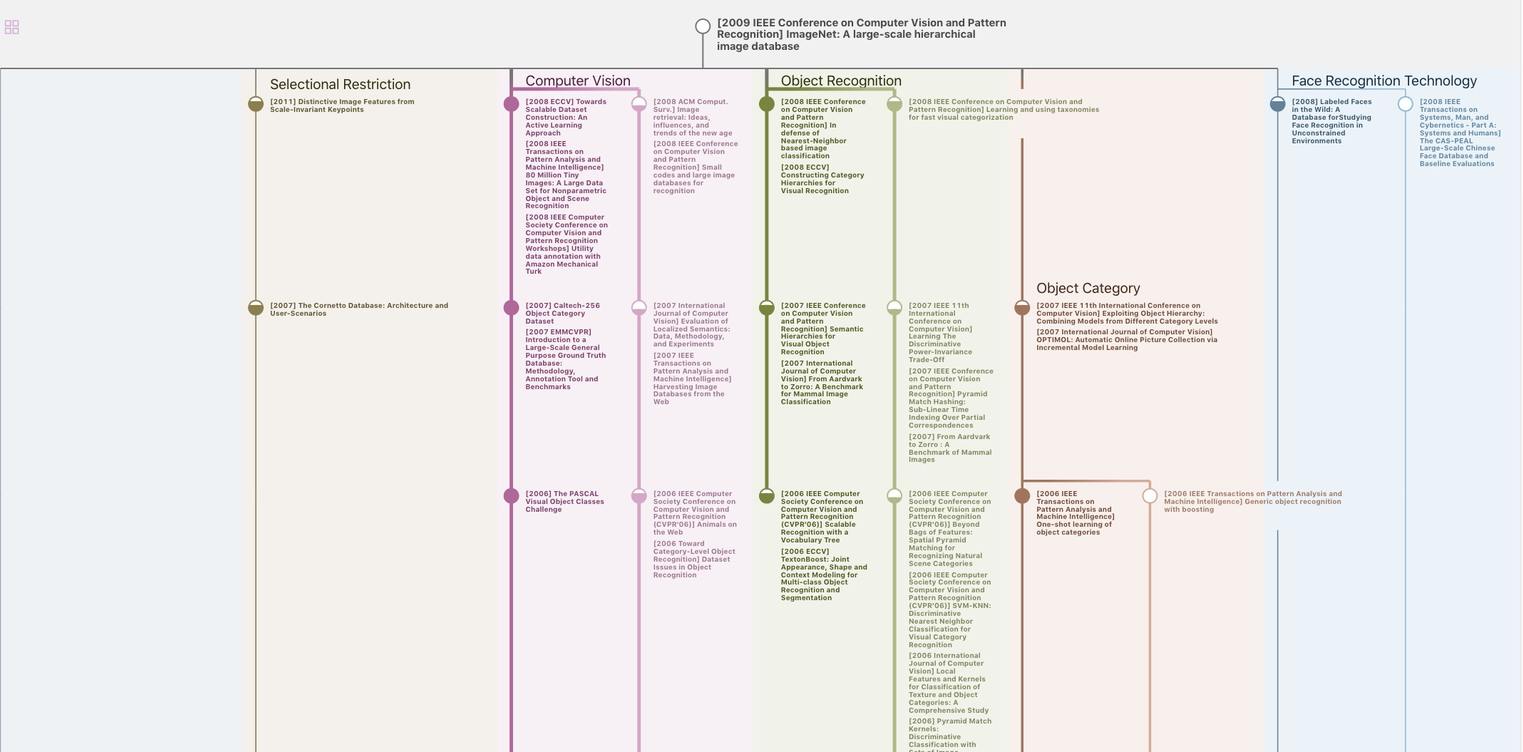
生成溯源树,研究论文发展脉络
Chat Paper
正在生成论文摘要