NP-SBFL: Bridging the Gap Between Spectrum-Based Fault Localization and Faulty Neural Pathways Diagnosis
CoRR(2023)
摘要
Deep learning has revolutionized various real-world applications, but the
quality of Deep Neural Networks (DNNs) remains a concern. DNNs are complex and
have millions of parameters, making it difficult to determine their
contributions to fulfilling a task. Moreover, the behavior of a DNN is highly
influenced by the data used during training, making it challenging to collect
enough data to exercise all potential DNN behavior under all possible
scenarios. This paper proposes a novel NP-SBFL method that adapts
spectrum-based fault localization (SBFL) to locate faulty neural pathways. Our
method identifies critical neurons using the layer-wise relevance propagation
(LRP) technique and determines which critical neurons are faulty. We propose a
multi-stage gradient ascent (MGA), an extension of gradient ascent, to
effectively activate a sequence of neurons one at a time while maintaining the
activation of previous neurons. We evaluated the effectiveness of our method on
two commonly used datasets, MNIST and CIFAR-10, two baselines DeepFault and
NP-SBFL-GA, and three suspicious neuron measures, Tarantula, Ochiai, and
Barinel. The empirical results showed that NP-SBFL-MGA is statistically more
effective than the baselines at identifying suspicious paths and synthesizing
adversarial inputs. Particularly, Tarantula on NP-SBFL-MGA had the highest
fault detection rate at 96.75%, surpassing DeepFault on Ochiai (89.90%) and
NP-SBFL-GA on Ochiai (60.61%). Our approach also yielded comparable results to
the baselines in synthesizing naturalness inputs, and we found a positive
correlation between the coverage of critical paths and the number of failed
tests in DNN fault localization.
更多查看译文
AI 理解论文
溯源树
样例
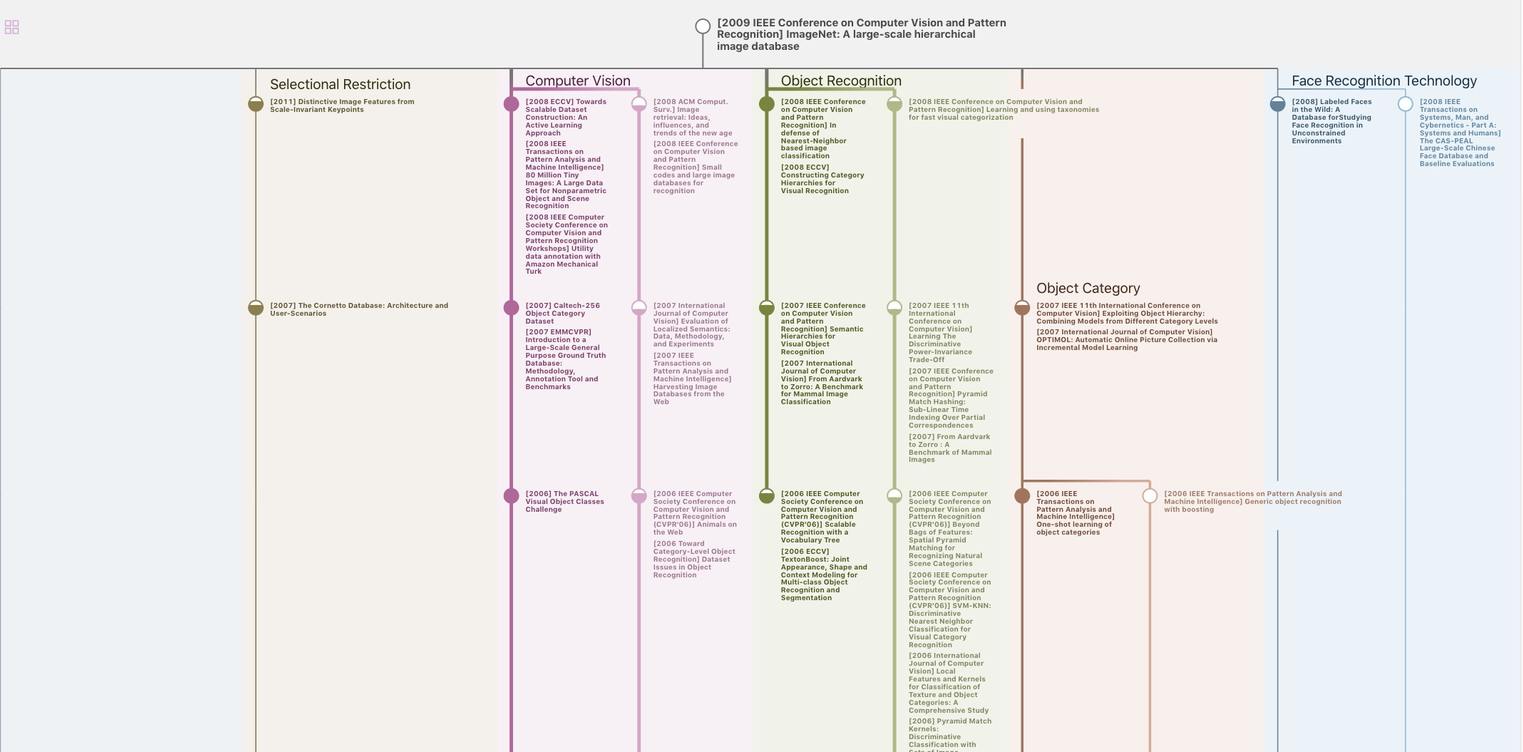
生成溯源树,研究论文发展脉络
Chat Paper
正在生成论文摘要