Dealing with missing data in laboratory test results used as a baseline covariate: results of multi-hospital cohort studies utilizing a database system contributing to MID-NET ® in Japan
BMC medical informatics and decision making(2023)
摘要
Background To evaluate missing data methods applied to laboratory test results used for confounding adjustment, utilizing data from 10 MID-NET ® -collaborative hospitals. Methods Using two scenarios, five methods dealing with missing laboratory test results were applied, including three missing data methods (single regression imputation (SRI), multiple imputation (MI), and inverse probability weighted (IPW) method). We compared the point estimates of adjusted hazard ratios (aHRs) and 95% confidence intervals (CIs) between the five methods. Hospital variability in missing data was considered using the hospital-specific approach and overall approach. Confounding adjustment methods were propensity score (PS) weighting, PS matching, and regression adjustment. Results In Scenario 1, the risk of diabetes due to second-generation antipsychotics was compared with that due to first-generation antipsychotics. The aHR adjusted by PS weighting using SRI, MI, and IPW by the hospital-specific-approach was 0.61 [95%CI, 0.39–0.96], 0.63 [95%CI, 0.42–0.93], and 0.76 [95%CI, 0.46–1.25], respectively. In Scenario 2, the risk of liver injuries due to rosuvastatin was compared with that due to atorvastatin. Although PS matching largely contributed to differences in aHRs between methods, PS weighting provided no substantial difference in point estimates of aHRs between SRI and MI, similar to Scenario 1. The results of SRI and MI in both scenarios showed no considerable changes, even upon changing the approaches considering hospital variations. Conclusions SRI and MI provide similar point estimates of aHR. Two approaches considering hospital variations did not markedly affect the results. Adjustment by PS matching should be used carefully.
更多查看译文
关键词
missing data,baseline covariate,laboratory test results,cohort,multi-hospital,mid-net
AI 理解论文
溯源树
样例
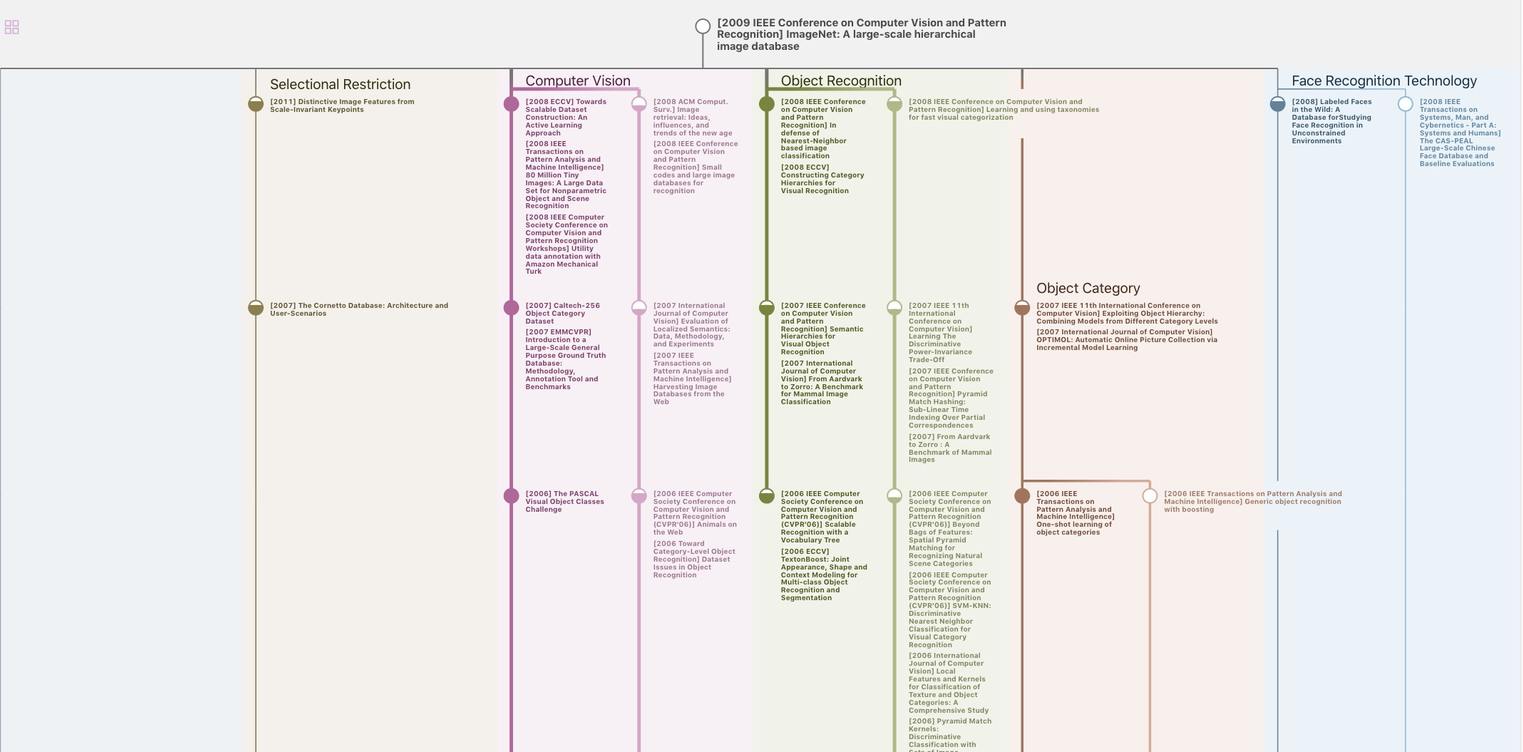
生成溯源树,研究论文发展脉络
Chat Paper
正在生成论文摘要