A new LASSO-BiLSTM-based ensemble learning approach for exchange rate forecasting
ENGINEERING APPLICATIONS OF ARTIFICIAL INTELLIGENCE(2024)
Abstract
Foreign exchange rate affects many countries' economic status and development. Therefore, it is essential to find the factors affecting the exchange rate price and make reasonable predictions. This paper proposes the novel LASSO-BiLSTM-based ensemble learning method by integrating least absolute shrinkage and selection operator (LASSO) and bidirectional long short-term memory (LSTM) to predict the USD/CNY exchange rate. First, 29 variables are selected to reflect economic activities on market and macroeconomic levels. Then, LASSO-BiLSTMbased ensemble learning approach is adopted with two steps: 1) LASSO is used to select six highly correlated variables with the exchange rate to reduce noises. 2) BiLSTM is employed to forecast the exchange rate with the six chosen variables. Last, to test the effectiveness of BiLSTM, comparisons with four deep learning algorithms, which are extreme learning machine (ELM), kernel extreme learning machine (KELM), long short-term memory (LSTM), and support vector regression (SVR), are conducted. The result shows that LASSO-BiLSTM outperforms the other models in 1-step forecast (MAE: 0.051, RMSE: 0.072, MDA: 0.777). The same conclusion applies to 3steps and 6-steps forecasts. Overall, the proposed LASSO-BiLSTM-based ensemble learning method demonstrates high potential in time series forecasting.
MoreTranslated text
Key words
Exchange rate forecasting,Macroeconomic factors,Market-based variables,Time series forecasting
AI Read Science
Must-Reading Tree
Example
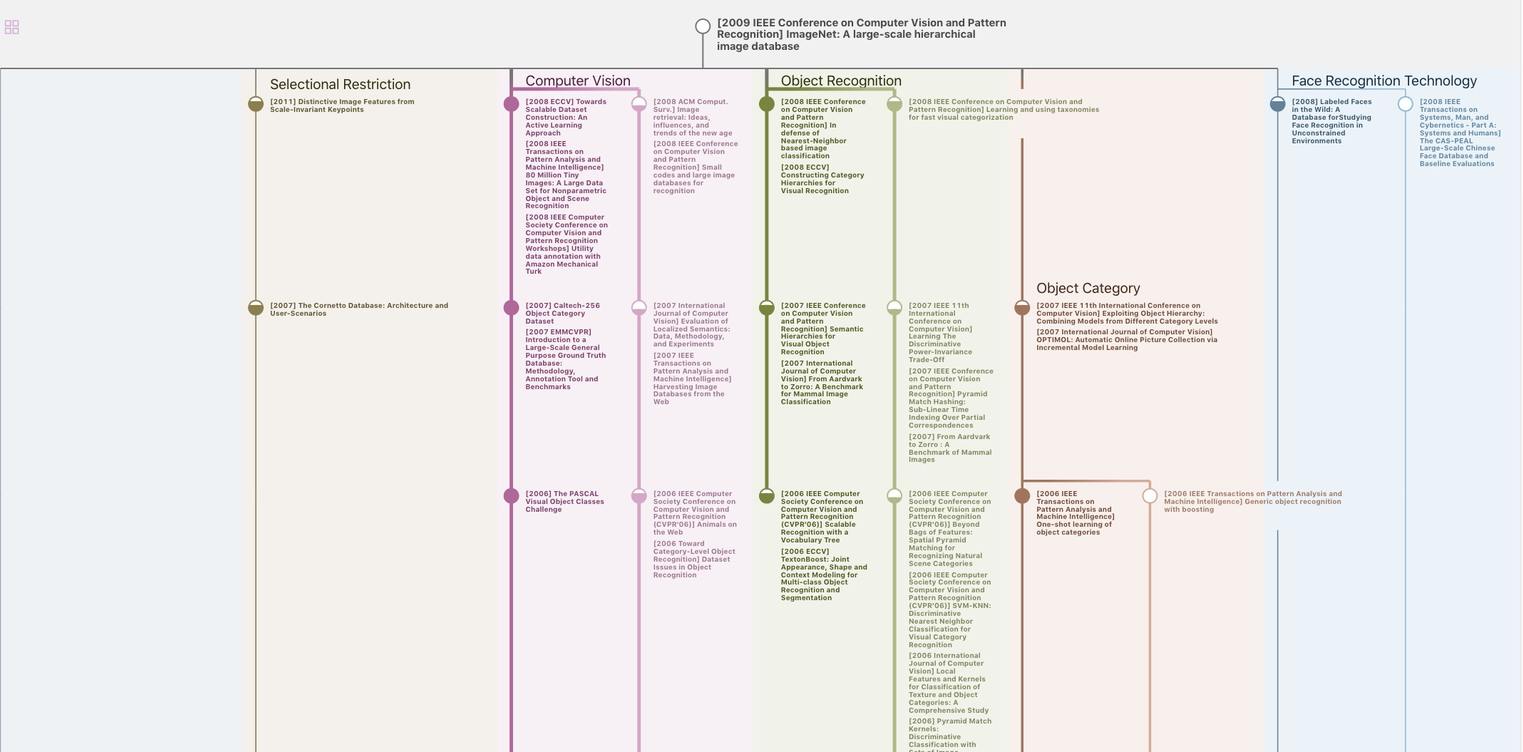
Generate MRT to find the research sequence of this paper
Chat Paper
Summary is being generated by the instructions you defined