Raising the ClaSS of Streaming Time Series Segmentation
CoRR(2023)
摘要
Ubiquitous sensors today emit high frequency streams of numerical measurements that reflect properties of human, animal, industrial, commercial, and natural processes. Shifts in such processes, e.g. caused by external events or internal state changes, manifest as changes in the recorded signals. The task of streaming time series segmentation (STSS) is to partition the stream into consecutive variable-sized segments that correspond to states of the observed processes or entities. The partition operation itself must in performance be able to cope with the input frequency of the signals. We introduce ClaSS, a novel, efficient, and highly accurate algorithm for STSS. ClaSS assesses the homogeneity of potential partitions using self-supervised time series classification and applies statistical tests to detect significant change points (CPs). In our experimental evaluation using two large benchmarks and six real-world data archives, we found ClaSS to be significantly more precise than eight state-of-the-art competitors. Its space and time complexity is independent of segment sizes and linear only in the sliding window size. We also provide ClaSS as a window operator with an average throughput of 538 data points per second for the Apache Flink streaming engine.
更多查看译文
关键词
segmentation,time series
AI 理解论文
溯源树
样例
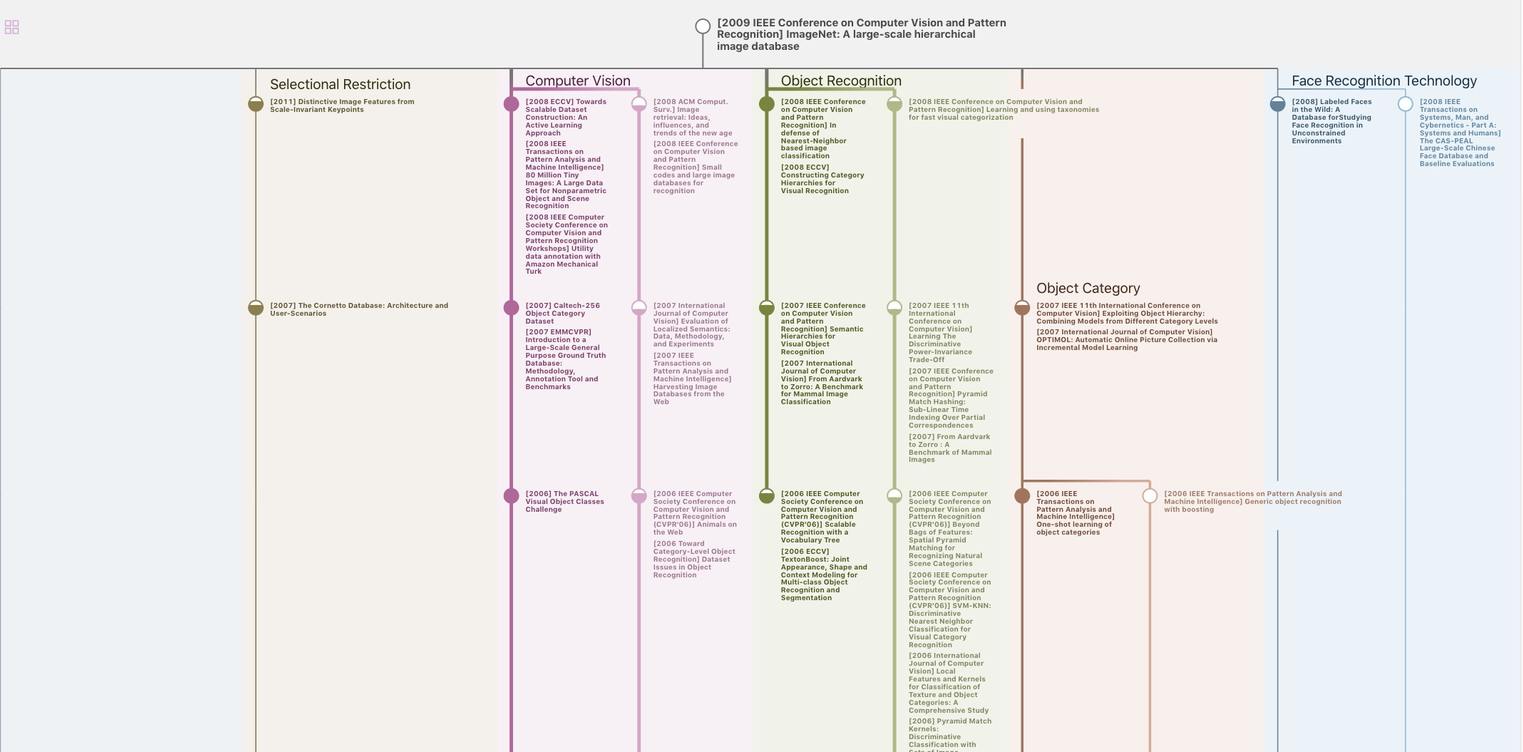
生成溯源树,研究论文发展脉络
Chat Paper
正在生成论文摘要