A physics-informed GAN framework based on model-free data-driven computational mechanics
COMPUTER METHODS IN APPLIED MECHANICS AND ENGINEERING(2024)
摘要
Model -free data -driven computational mechanics, first proposed by Kirchdoerfer and Ortiz, replace phenomenological models with numerical simulations based on sample datasets in strain-stress space. In this study, we integrate this paradigm within physics -informed generative adversarial networks (GANs). We enhance the conventional physics -informed neural network framework by implementing the principles of data -driven computational mechanics into GANs. Specifically, the generator is informed by physical constraints, while the discriminator utilizes the closest strain-stress data to discern the authenticity of the generator's output. This combined approach presents a new formalism to harness data -driven mechanics and deep learning to simulate and predict mechanical behaviors.
更多查看译文
关键词
Model-free data-driven,Generative adversarial networks,Data-driven computing,Physics-informed neural networks
AI 理解论文
溯源树
样例
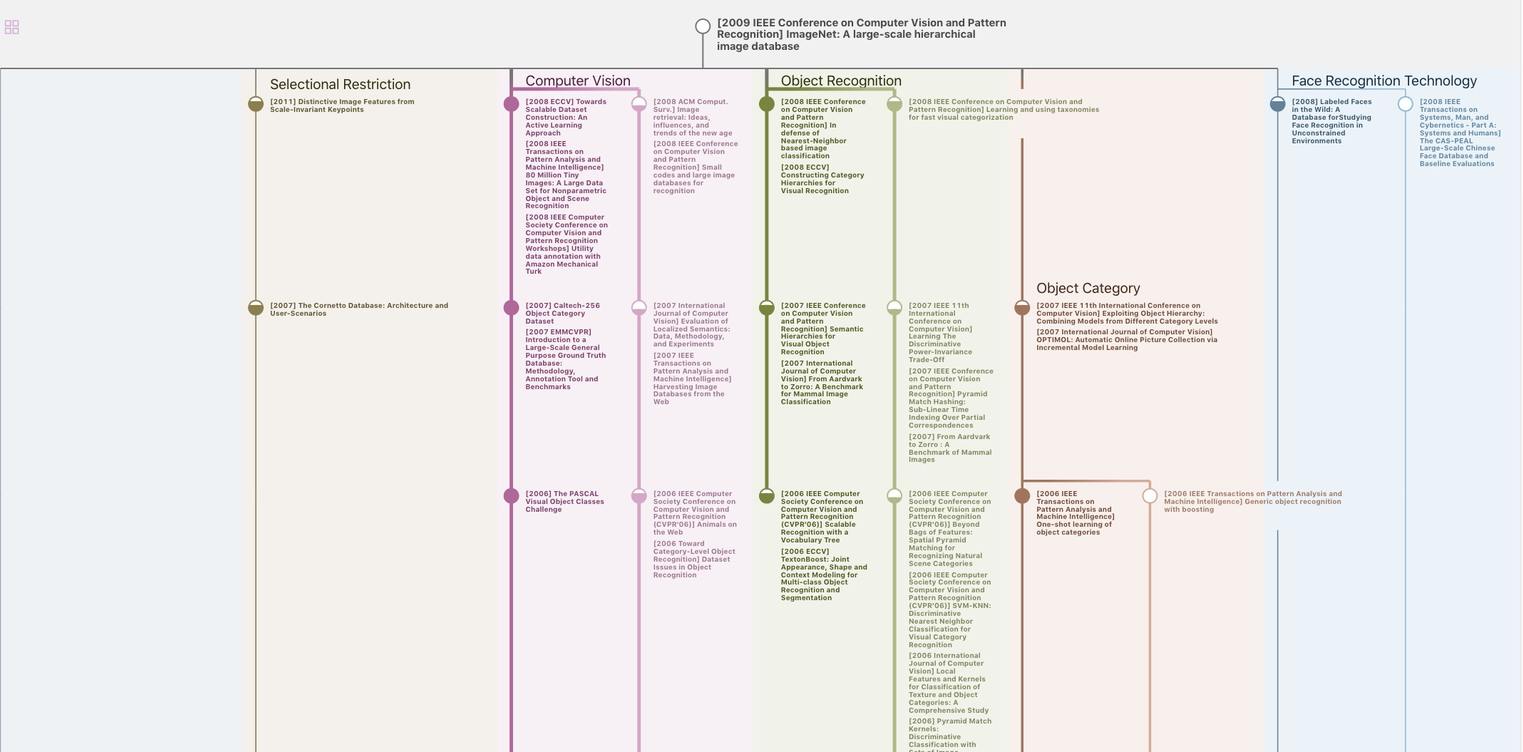
生成溯源树,研究论文发展脉络
Chat Paper
正在生成论文摘要