Beyond U: Making Diffusion Models Faster & Lighter
CoRR(2023)
摘要
Diffusion models are a family of generative models that yield record-breaking performance in tasks such as image synthesis, video generation, and molecule design. Despite their capabilities, their efficiency, especially in the reverse denoising process, remains a challenge due to slow convergence rates and high computational costs. In this work, we introduce an approach that leverages continuous dynamical systems to design a novel denoising network for diffusion models that is more parameter-efficient, exhibits faster convergence, and demonstrates increased noise robustness. Experimenting with denoising probabilistic diffusion models, our framework operates with approximately a quarter of the parameters and 30% of the Floating Point Operations (FLOPs) compared to standard U-Nets in Denoising Diffusion Probabilistic Models (DDPMs). Furthermore, our model is up to 70% faster in inference than the baseline models when measured in equal conditions while converging to better quality solutions.
更多查看译文
关键词
diffusion models faster,diffusion models
AI 理解论文
溯源树
样例
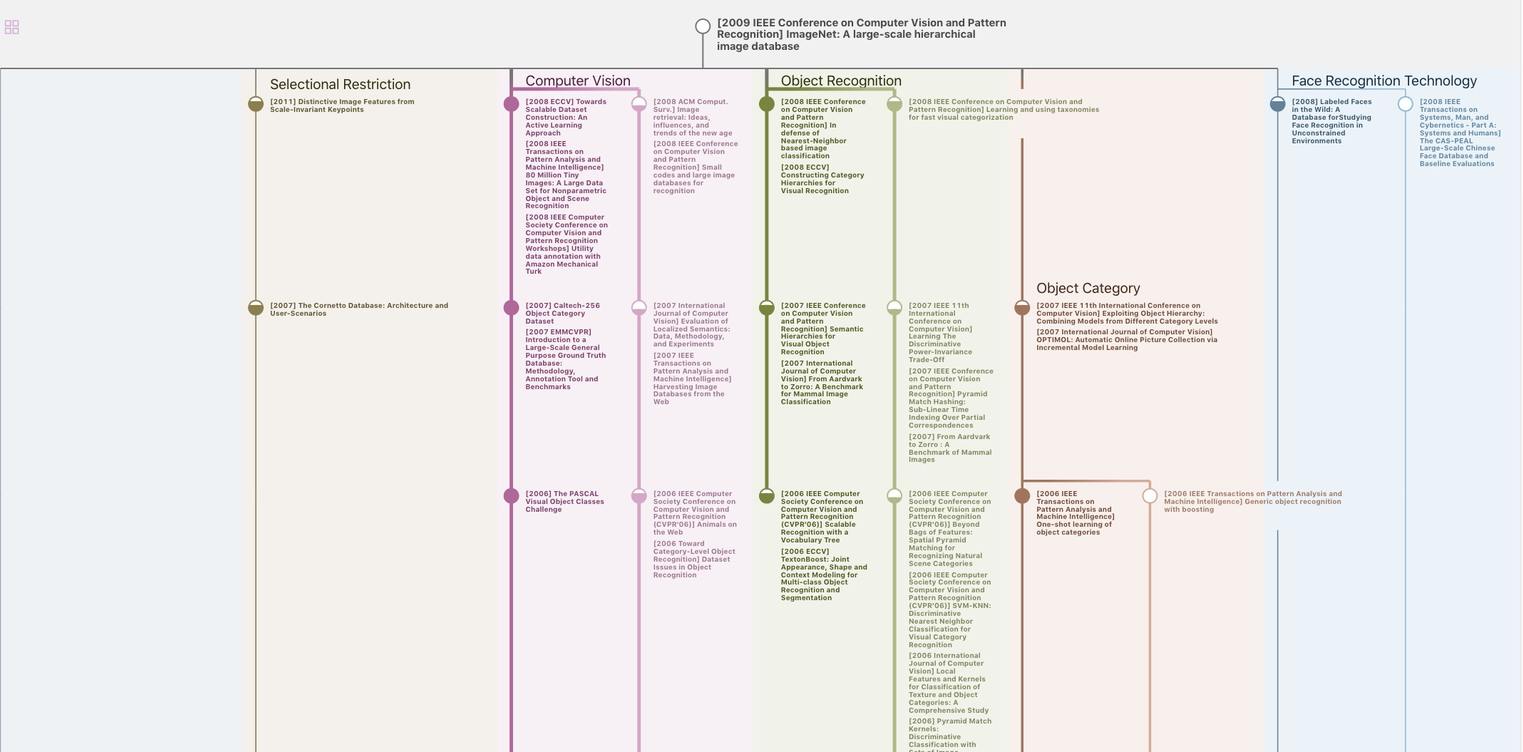
生成溯源树,研究论文发展脉络
Chat Paper
正在生成论文摘要