Topology Recoverability Prediction for Ad-Hoc Robot Networks: A Data-Driven Fault-Tolerant Approach
IEEE TRANSACTIONS ON SIGNAL AND INFORMATION PROCESSING OVER NETWORKS(2023)
摘要
Faults occurring in ad-hoc robot networks may fatally perturb their topologies leading to disconnection of subsets of those networks. Optimal topology synthesis is generally resource-intensive and time-consuming to be done in real time for large ad-hoc robot networks. One should only perform topology re-computations if the probability of topology recoverability after the occurrence of any fault surpasses that of its irrecoverability. We formulate this problem as a binary classification problem. Then, we develop a two-pathway data-driven model based on Bayesian Gaussian mixture models that predicts the solution to a typical problem by two different pre-fault and post-fault prediction pathways. The results, obtained by the integration of the predictions of those pathways, clearly indicate the success of our model in solving the topology (ir)recoverability prediction problem compared to the best of current strategies found in the literature.
更多查看译文
关键词
Ad-hoc robot networks,Bayesian Gaussin mixture model,fault-tolerant prediction,mobile robotics,topology prediction
AI 理解论文
溯源树
样例
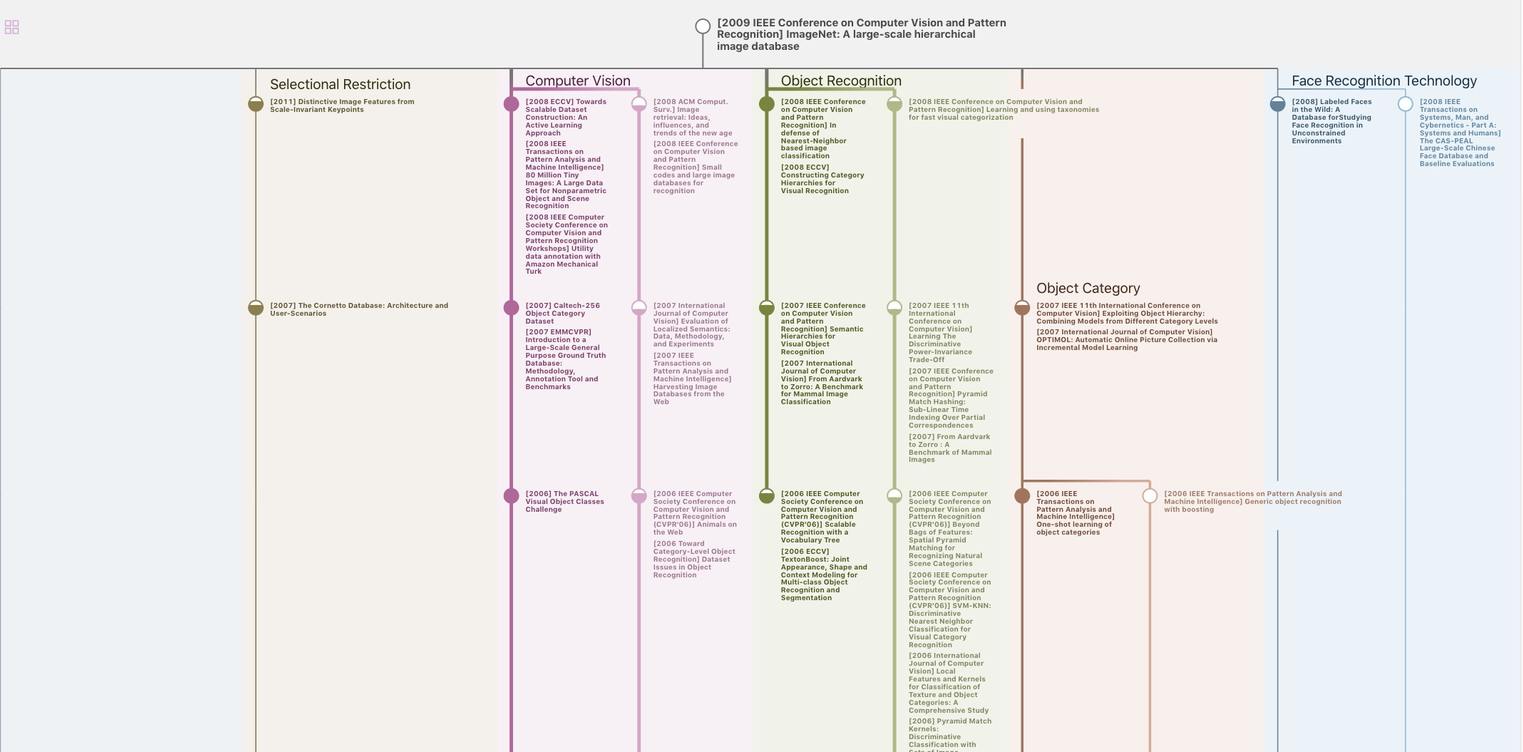
生成溯源树,研究论文发展脉络
Chat Paper
正在生成论文摘要