Multiscale Feature Attribution for Outliers
CoRR(2023)
摘要
Machine learning techniques can automatically identify outliers in massive datasets, much faster and more reproducible than human inspection ever could. But finding such outliers immediately leads to the question: which features render this input anomalous? We propose a new feature attribution method, Inverse Multiscale Occlusion, that is specifically designed for outliers, for which we have little knowledge of the type of features we want to identify and expect that the model performance is questionable because anomalous test data likely exceed the limits of the training data. We demonstrate our method on outliers detected in galaxy spectra from the Dark Energy Survey Instrument and find its results to be much more interpretable than alternative attribution approaches.
更多查看译文
关键词
multiscale,feature
AI 理解论文
溯源树
样例
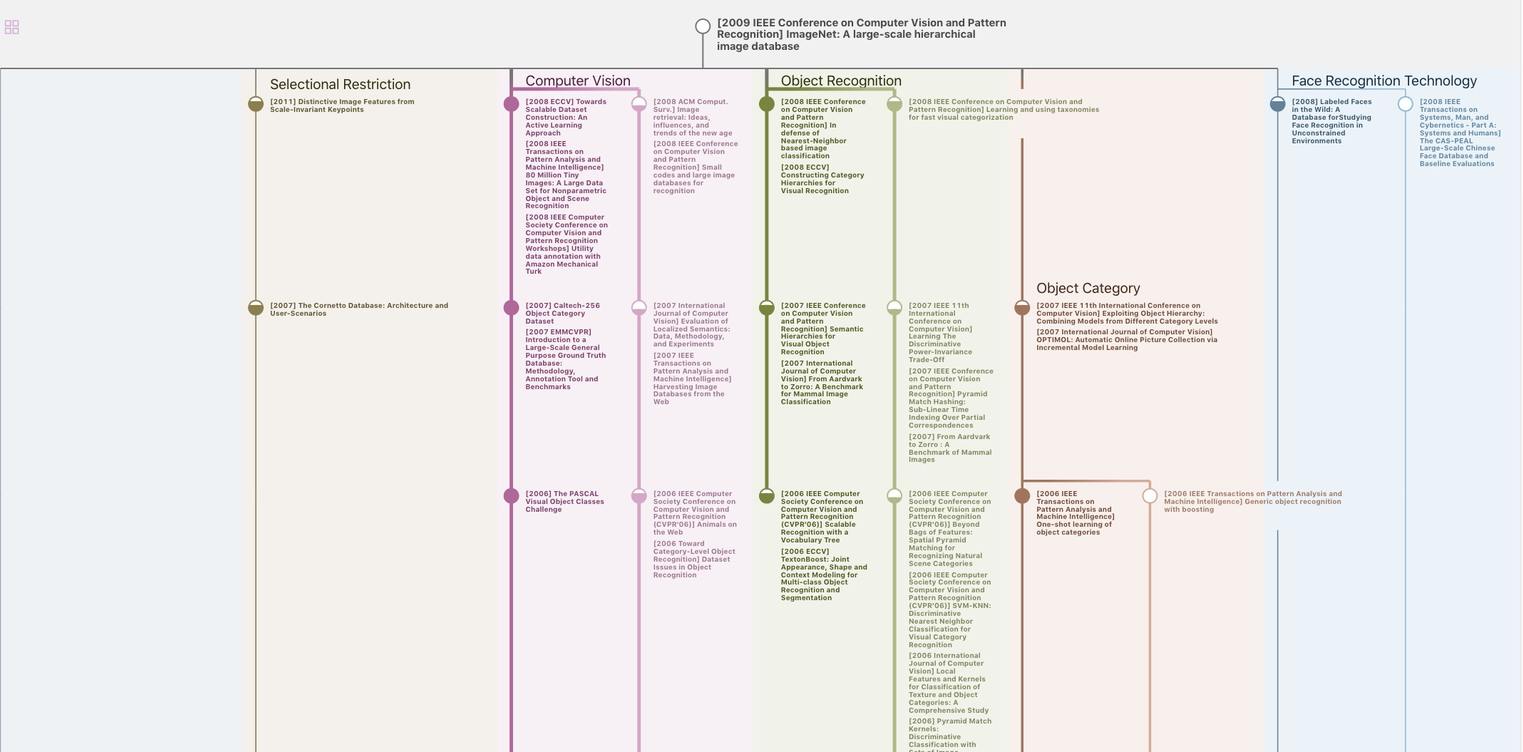
生成溯源树,研究论文发展脉络
Chat Paper
正在生成论文摘要