New Asymptotic Limit Theory and Inference for Monotone Regression
arXiv (Cornell University)(2023)
摘要
Nonparametric regression problems with qualitative constraints such as monotonicity or convexity are ubiquitous in applications. For example, in predicting the yield of a factory in terms of the number of labor hours, the monotonicity of the conditional mean function is a natural constraint. One can estimate a monotone conditional mean function using nonparametric least squares estimation, which involves no tuning parameters. Several interesting properties of the isotonic LSE are known including its rate of convergence, adaptivity properties, and pointwise asymptotic distribution. However, we believe that the full richness of the asymptotic limit theory has not been explored in the literature which we do in this paper. Moreover, the inference problem is not fully settled. In this paper, we present some new results for monotone regression including an extension of existing results to triangular arrays, and provide asymptotically valid confidence intervals that are uniformly valid over a large class of distributions.
更多查看译文
关键词
limit,inference,theory
AI 理解论文
溯源树
样例
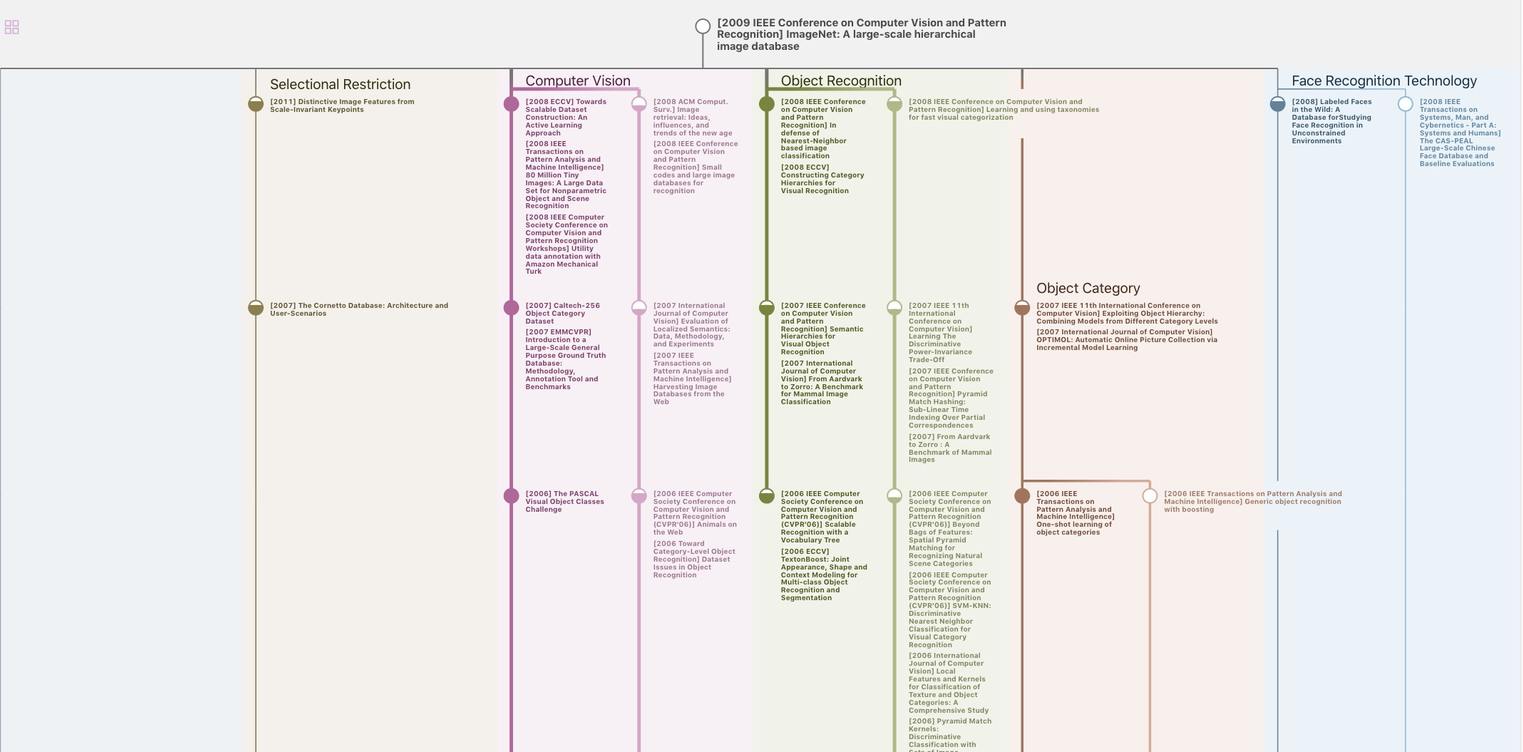
生成溯源树,研究论文发展脉络
Chat Paper
正在生成论文摘要