Gradient-free online learning of subgrid-scale dynamics with neural emulators
CoRR(2023)
摘要
In this paper, we propose a generic algorithm to train machine learning-based subgrid parametrizations online, i.e., with $\textit{a posteriori}$ loss functions for non-differentiable numerical solvers. The proposed approach leverage neural emulators to train an approximation of the reduced state-space solver, which is then used to allows gradient propagation through temporal integration steps. The algorithm is able to recover most of the benefit of online strategies without having to compute the gradient of the original solver. It is demonstrated that training the neural emulator and parametrization components separately with respective loss quantities is necessary in order to minimize the propagation of some approximation bias.
更多查看译文
关键词
dynamics,neural
AI 理解论文
溯源树
样例
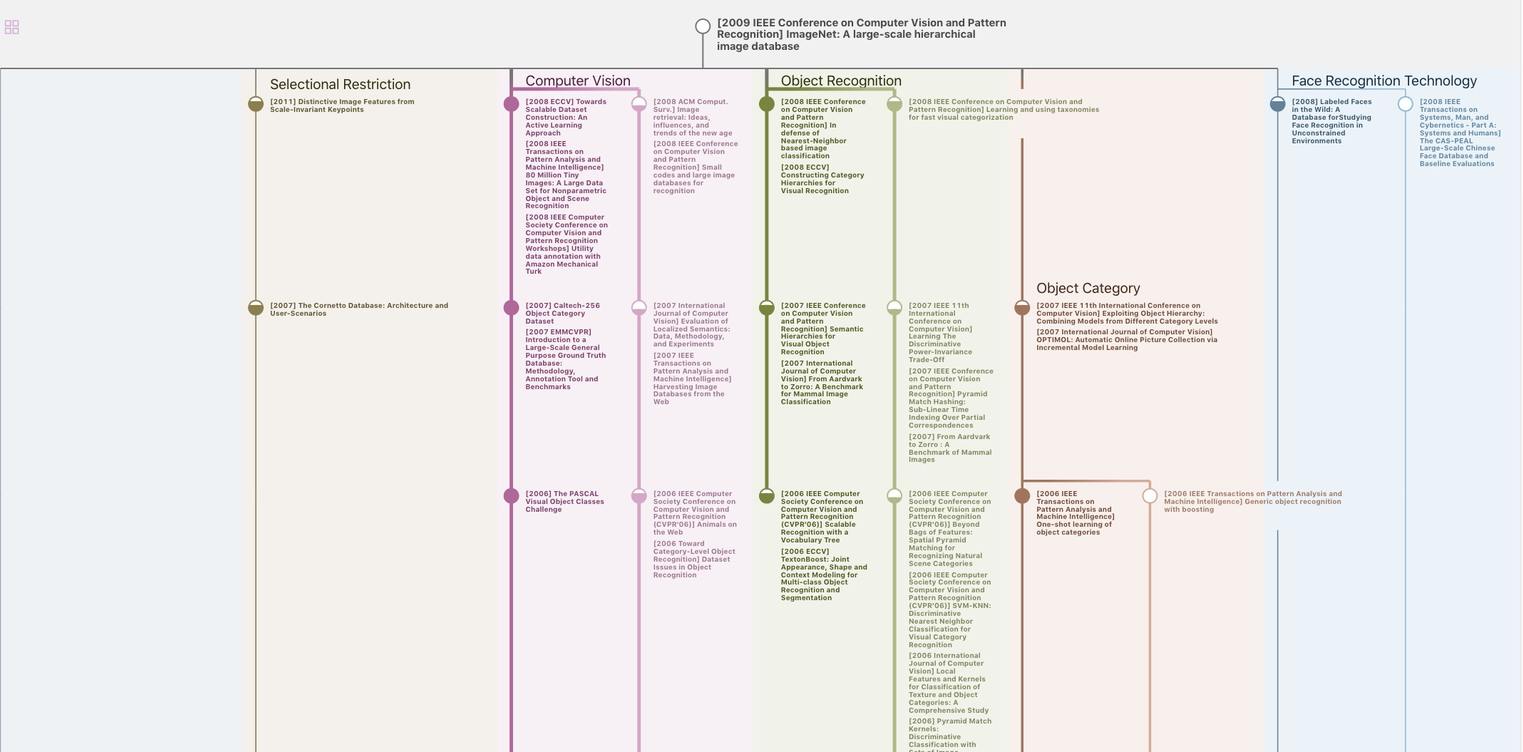
生成溯源树,研究论文发展脉络
Chat Paper
正在生成论文摘要