A novel continual reinforcement learning-based expert system for self-optimization of soft real-time systems
EXPERT SYSTEMS WITH APPLICATIONS(2024)
Abstract
Virtual globes are soft real-time systems, which stream multi-resolution data sets and render world-scale landscapes in real-time. Such systems require an adaptation mechanism to fulfill user and quality of experience (QoE) requirements. Configuration and tuning of parameters to optimize system performance is challenging due to run-time uncertainties. In this work, we propose a continual reinforcement learning-based expert system for self-optimization of a soft real-time system to meet user and quality objectives. The proposed system is capable of learning continuously by utilizing the previously learned knowledge to fulfill adaptation requirements. The proposed managing system interacts with a managed system and evolves a knowledge base of adaptation rules for run-time optimization of the managed system to meet user and quality objectives. We devise a learning classifier system for the evolution, storage, and transfer of adaptation rules using a code fragments-based rich encoding scheme. We transform user goals, system states, and execution environment conditions into observable states. We validated the proposed system for the same and cross-domain knowledge transfer and reuse scenarios for different user goals and execution environments. The results of the proposed system are compared with baseline and state-of-the-art methods where the proposed system outperformed the methods in terms of adaptation accuracy.
MoreTranslated text
Key words
Self-adaptive systems,Continual learning,Autonomic computing,Reinforcement learning,Learning classifier systems
AI Read Science
Must-Reading Tree
Example
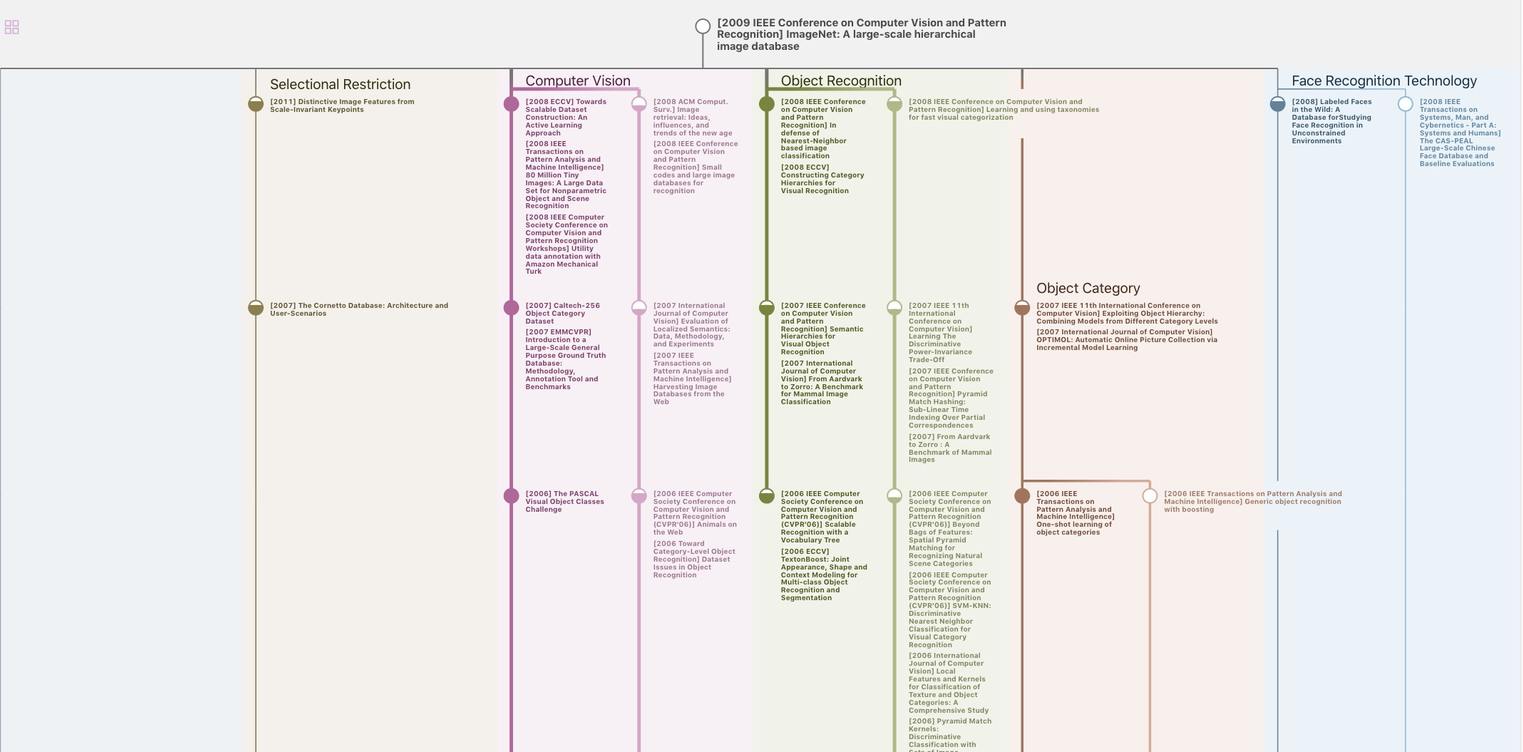
Generate MRT to find the research sequence of this paper
Chat Paper
Summary is being generated by the instructions you defined