Ensemble Learning to Identify Depression Indicators for Korean Farmers
IEEE Access(2023)
摘要
Understanding the factors contributing to depression in farmers is crucial for ensuring their well-being and productivity. To address this issue, our study delves into depression factors among farmers, employing advanced tree-based machine learning (ML) algorithms, specifically focusing on the Category Boosting (CatB) algorithm. Applying the Patient Health Questionnaire-9 (PHQ-9) criteria, 2,446 individuals among 14,810 repondents were classified into depression including mild symptoms. In the classification, CatB achieved an impressive 79.7% accuracy and 81.4% F1 score compared to the other tree-based ensemble models (Random Forest - RF, Extra Trees - ET, and XGBoost - XGB). RF showed the highest sensitivity at 90.0% and the 81.3% F1 score followed by CatB. For the feature importances, the Gini impurity was predominantly used to assess in the RF and ET models. Through the analysis of feature importances, ‘Health’, ‘Sleep time’, ‘Busyness’, ‘Income’, and ‘Frequency of wearing protective gear’ were identified as significant features. These results highlighted the significance of treatment strategies for individuals at high risk. and developing treatment strategies for high-risk individuals in the agricultural sector. Empowering healthcare providers by giving them access to this tool can lead to more effective interventions, potentially reducing the burden of depression and enhancing farmers’ productivity.
更多查看译文
关键词
Ensemble learning,depression,feature importance,agricultural,risk factor,decision tree,PHQ-9
AI 理解论文
溯源树
样例
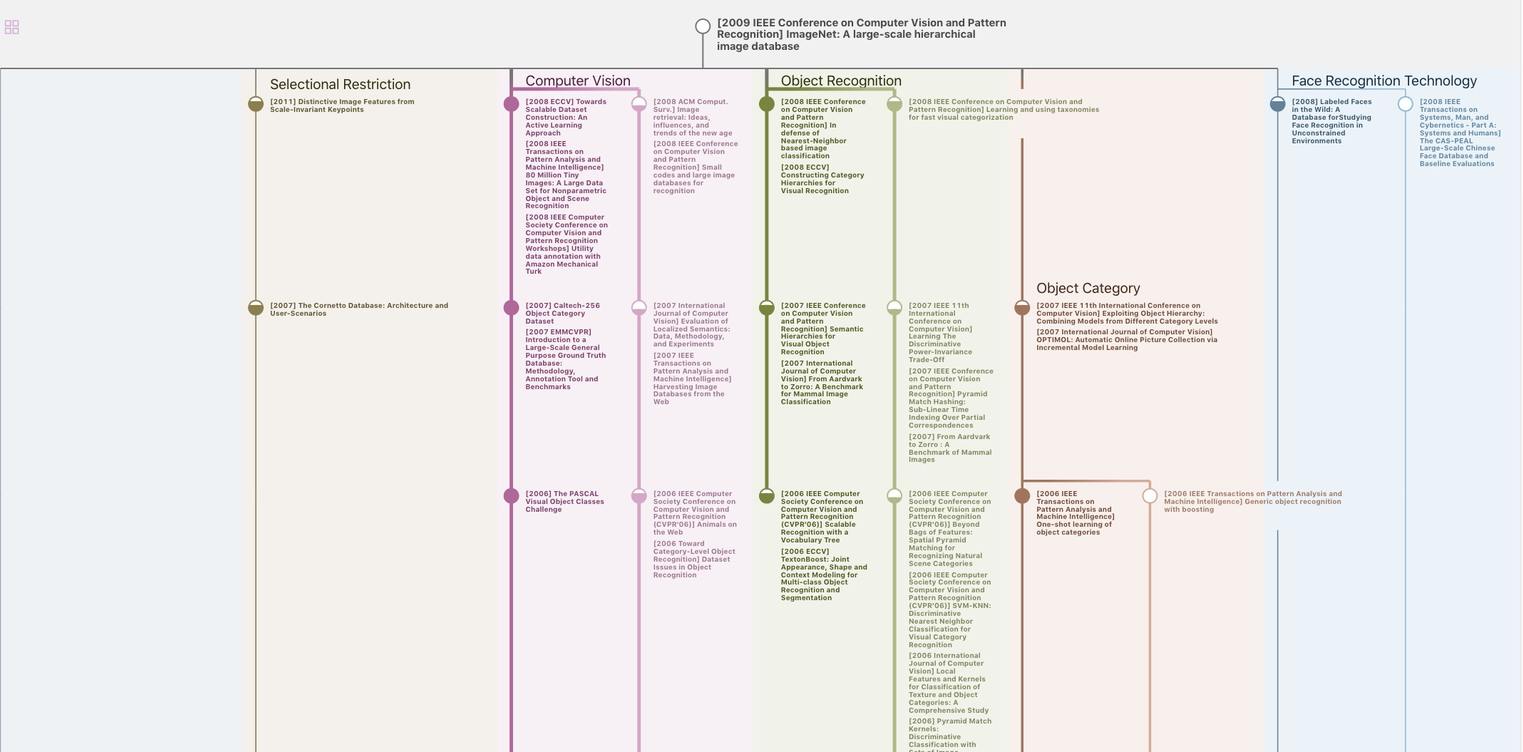
生成溯源树,研究论文发展脉络
Chat Paper
正在生成论文摘要