Deep Reinforcement Learning-based scheduling for optimizing system load and response time in edge and fog computing environments
FUTURE GENERATION COMPUTER SYSTEMS-THE INTERNATIONAL JOURNAL OF ESCIENCE(2024)
摘要
Edge/fog computing, as a distributed computing paradigm, satisfies the low-latency requirements of ever-increasing number of IoT applications and has become the mainstream computing paradigm behind IoT applications. However, because large number of IoT applications require execution on the edge/fog resources, the servers may be overloaded. Hence, it may disrupt the edge/fog servers and also negatively affect IoT applications' response time. Moreover, many IoT applications are composed of dependent components incurring extra constraints for their execution. Besides, edge/fog computing environments and IoT applications are inherently dynamic and stochastic. Thus, efficient and adaptive scheduling of IoT applications in heterogeneous edge/fog computing environments is of paramount importance. However, limited computational resources on edge/fog servers imposes an extra burden for applying optimal but computationally demanding techniques. To overcome these challenges, we propose a Deep Reinforcement Learning-based IoT application Scheduling algorithm, called DRLIS to adaptively and efficiently optimize the response time of heterogeneous IoT applications and balance the load of the edge/fog servers. We implemented DRLIS as a practical scheduler in the FogBus2 function-as-a-service framework for creating an edge-fog-cloud integrated serverless computing environment. Results obtained from extensive experiments show that DRLIS significantly reduces the execution cost of IoT applications by up to 55%, 37%, and 50% in terms of load balancing, response time, and weighted cost, respectively, compared with metaheuristic algorithms and other reinforcement learning techniques.
更多查看译文
关键词
Edge computing,Fog computing,Machine learning,Deep reinforcement learning,Internet of Things
AI 理解论文
溯源树
样例
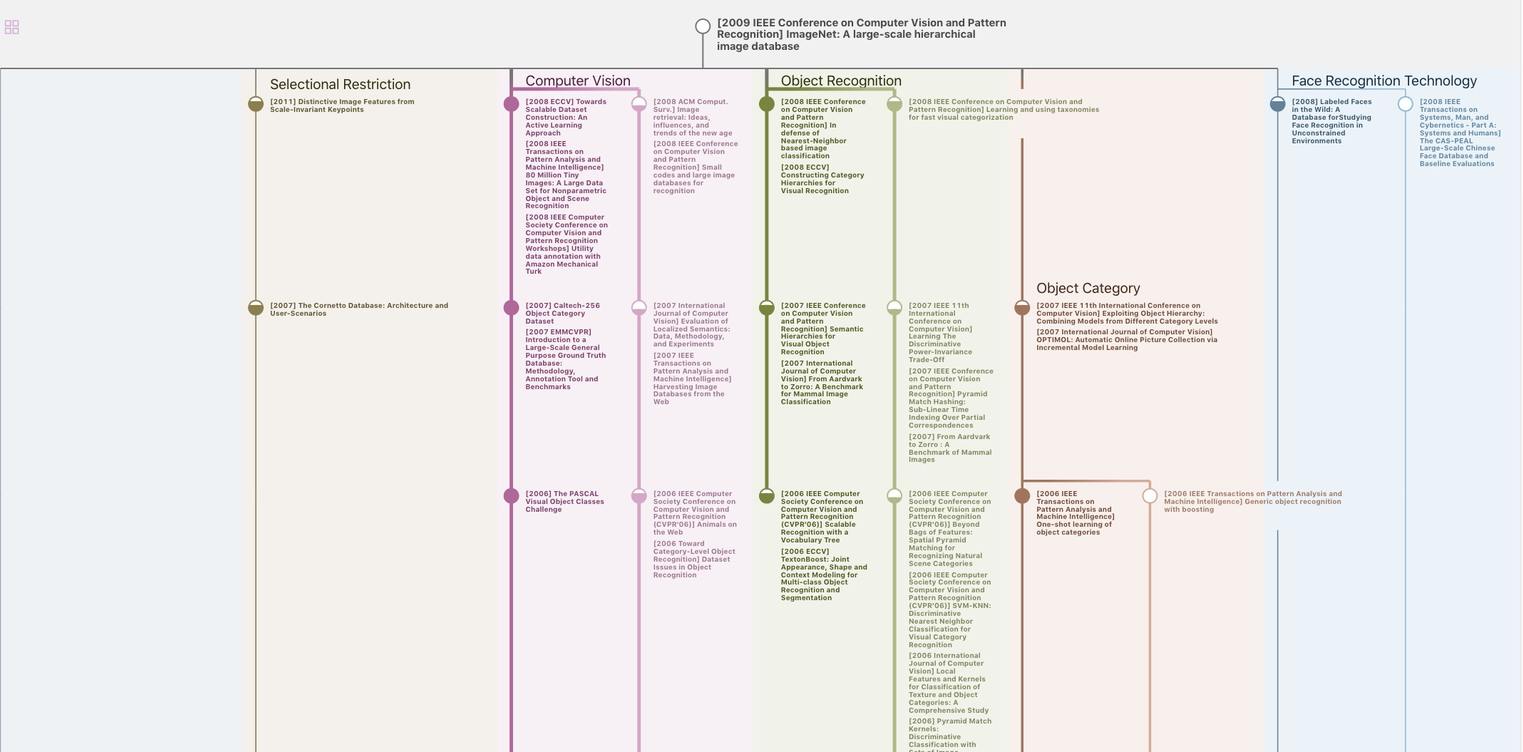
生成溯源树,研究论文发展脉络
Chat Paper
正在生成论文摘要