A multi-attention and depthwise separable convolution network for medical image segmentation
NEUROCOMPUTING(2024)
摘要
Automatic medical image segmentation method is highly needed to help experts in lesion segmentation. The deep learning technology emerging has profoundly driven the development of medical image segmentation. While U-Net and attention mechanisms are widely utilized in this field, the application of attention, albeit successful in natural scene image segmentation, tends to inflate the number of model parameters and neglects the potential for feature fusion between different convolutional layers. In response to these challenges, we present the Multi-Attention and Depthwise Separable Convolution U-Net (MDSU-Net), designed to enhance feature extraction. The multi-attention aspect of our framework integrates dual attention and attention gates, adeptly capturing rich contextual details and seamlessly fusing features across diverse convolutional layers. Additionally, our encoder integrates a depthwise separable convolution layer, streamlining the model's complexity without sacrificing its efficacy, ensuring versatility across various segmentation tasks. The results demonstrate that our method outperforms state-of-the-art across three diverse medical image datasets.
更多查看译文
关键词
U-Net,Dual attention,Attention gate,Depthwise separable convolution,Medical image segmentation
AI 理解论文
溯源树
样例
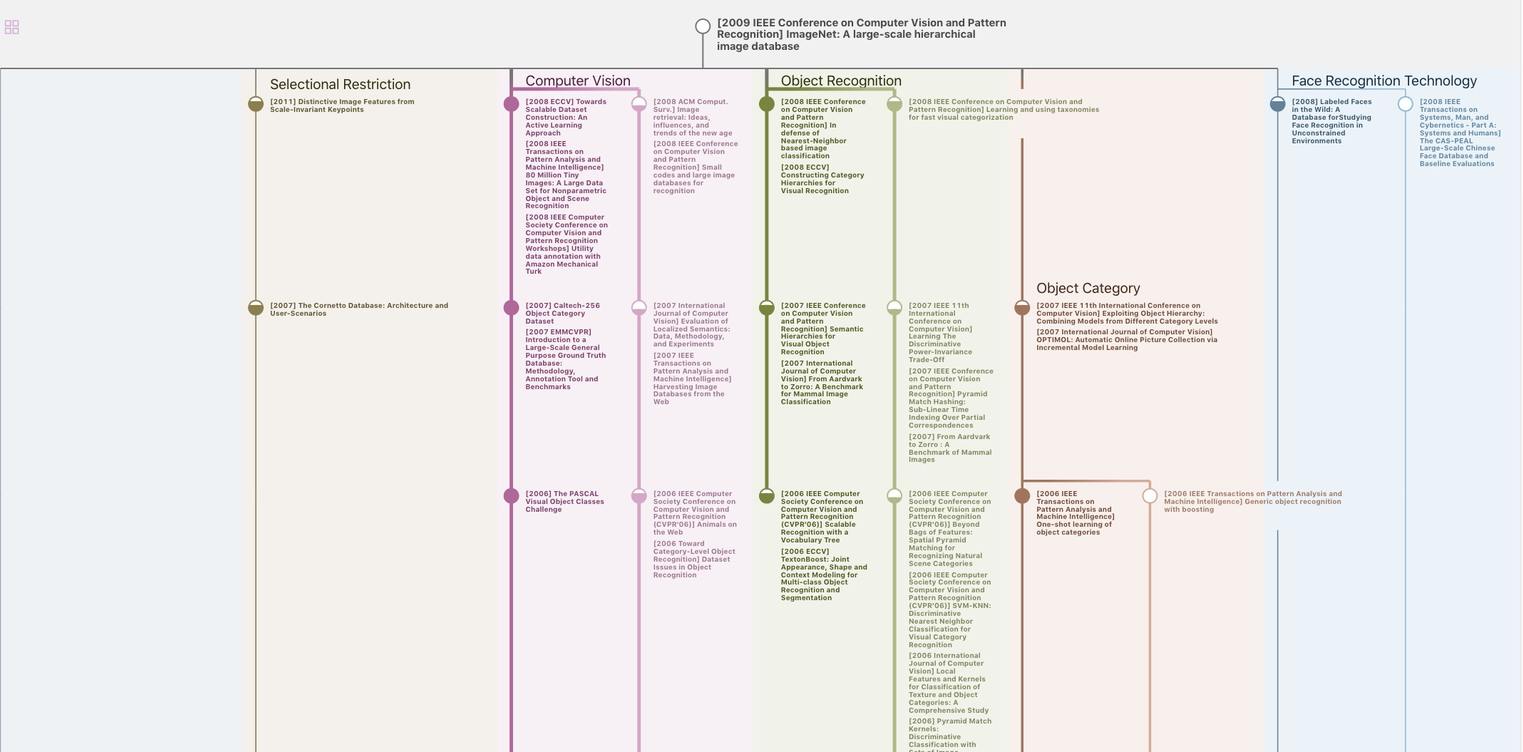
生成溯源树,研究论文发展脉络
Chat Paper
正在生成论文摘要