Network level enrichment provides a framework for biological interpretation of machine learning results
bioRxiv (Cold Spring Harbor Laboratory)(2023)
摘要
Machine learning algorithms are increasingly used to identify brain connectivity biomarkers linked to behavior and clinical outcomes. However, non-standard methodological choices in neuroimaging datasets, especially those with families or twins, have prevented robust machine learning applications. Additionally, prioritizing prediction accuracy over biological interpretability has made it challenging to understand the biological processes behind psychopathology. In this study, we employed a linear support vector regression model to study the relationship between resting-state functional connectivity networks and chronological age using data from the Human Connectome Project. We examined the effect of shared variance from twins and siblings by using cross-validation, either randomly assigning or keeping family members together. We also compared models with and without a Pearson feature filter and utilized a network enrichment approach to identify predictive brain networks. Results indicated that not accounting for shared family variance inflated prediction performance, and the Pearson filter reduced accuracy and reliability. Enhancing biological interpretability was achieved by inverting the machine learning model and applying network-level enrichment on the connectome, while directly using regression coefficients as feature weights led to misleading interpretations. Our findings offer crucial insights for applying machine learning to neuroimaging data, emphasizing the value of network enrichment for comprehensible biological interpretation.
### Competing Interest Statement
The authors have declared no competing interest.
更多查看译文
关键词
biological interpretation,enrichment,machine learning results,machine learning,network
AI 理解论文
溯源树
样例
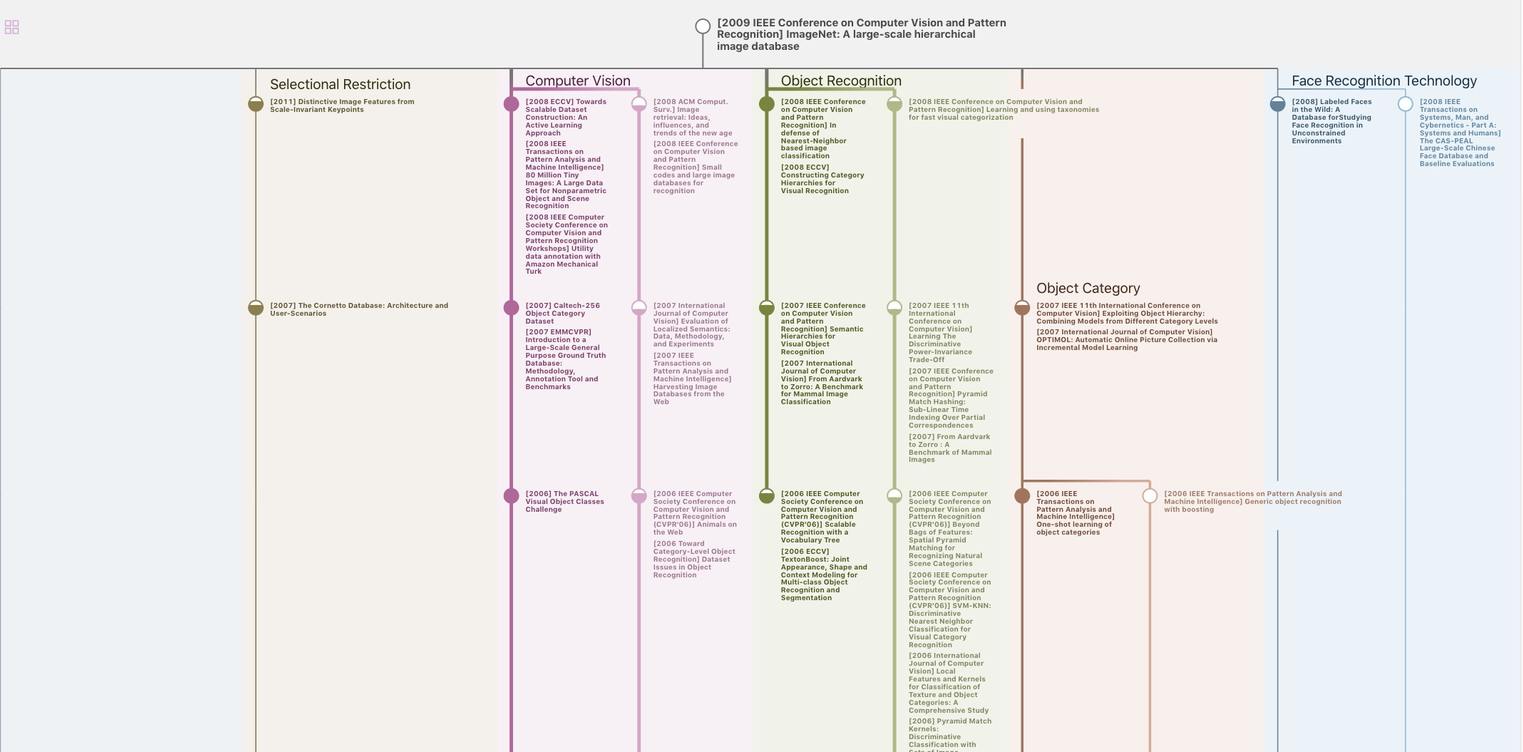
生成溯源树,研究论文发展脉络
Chat Paper
正在生成论文摘要