Data-driven motif discovery in biological neural networks
bioRxiv (Cold Spring Harbor Laboratory)(2023)
Abstract
Data from a variety of domains are represented as graphs, including social networks, transportation networks, computer networks, and biological networks. A key question spans these domains: are there meaningful repeated subgraphs, or motifs, within the structure of these larger networks? This is a particularly relevant problem when searching for repeated neural circuits in networks of biological neurons, as the field now regularly produces large brain connectivity maps of neurons and synapses, or connectomes. Given acquisition costs, however, these neuron-synapse connectivity maps are mostly one-of-a-kind. With current graph analysis techniques, it is very challenging to discover new “interesting” subgraphs a priori given small sample sizes of host graphs. Another challenge is that for even relatively modest graph sizes, an exhaustive search of all possible subgraphs is computationally intractable. For these reasons, motif discovery in biological graphs remains an unsolved challenge in the field. In this work, we present a motif discovery approach that can derive a list of undirected or directed motifs, with occurrence counts which are statistically significant compared to randomized graphs, from a single graph example. We first address common pitfalls in the current most common approaches when testing for motif statistical significance, and outline a strategy to ameliorate this problem with improved graph randomization techniques. We then propose a progressive-refinement approach for motif discovery, which addresses issues of computational cost. We demonstrate that our sampling correction technique allows for significance testing of target motifs while highlighting misleading conclusions from standard random graph models. Finally, we share our reference implementation, which is available as an open-source Python package, and demonstrate real-world preliminary results on the C. elegans connectome and the ellipsoid body of the Drosophila melanogaster fruit fly connectome.
### Competing Interest Statement
The authors have declared no competing interest.
MoreTranslated text
Key words
motif discovery,neural networks,data-driven
AI Read Science
Must-Reading Tree
Example
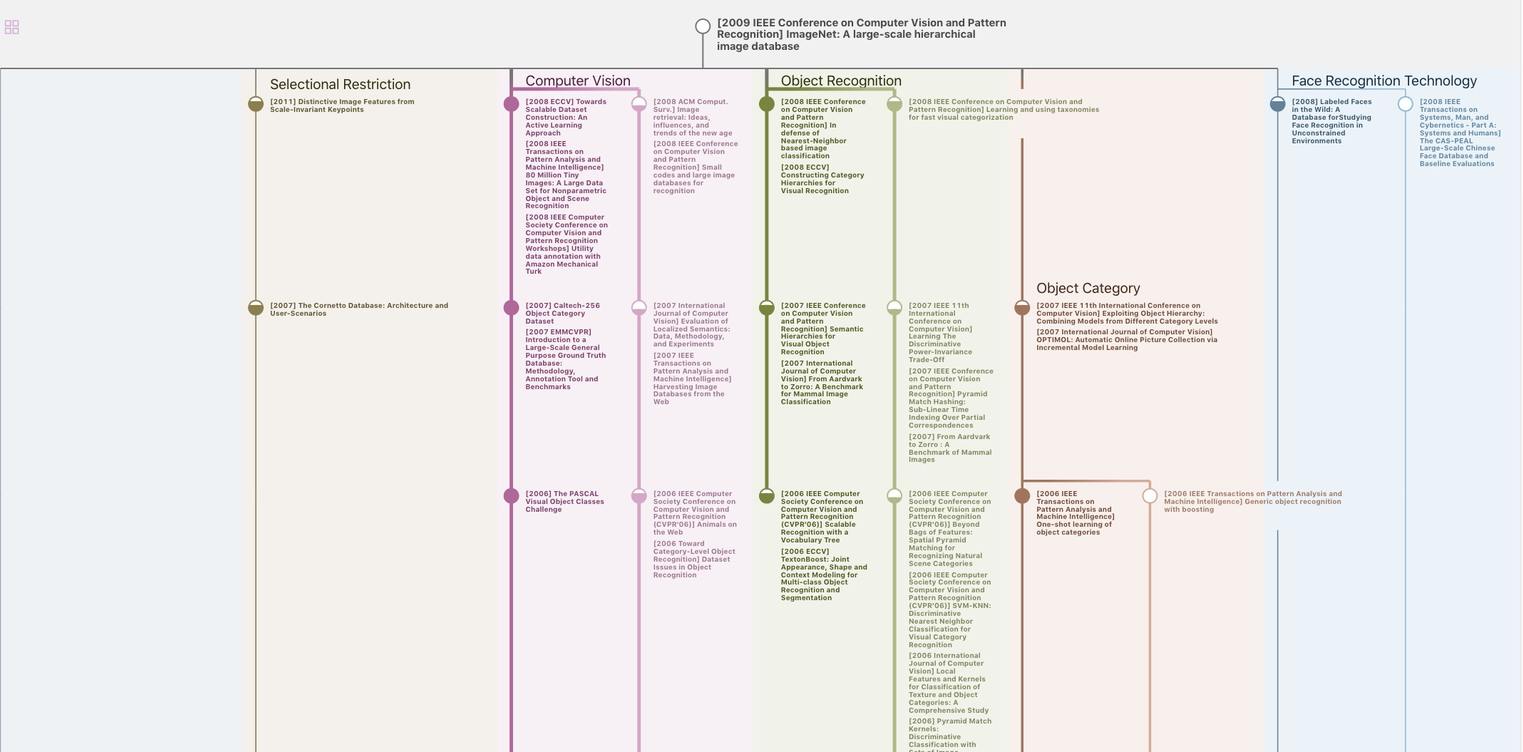
Generate MRT to find the research sequence of this paper
Chat Paper
Summary is being generated by the instructions you defined