A sequence context-based germline filter for structural variant calling from tumor samples without paired normal
bioRxiv (Cold Spring Harbor Laboratory)(2023)
摘要
There is currently no method to distinguish between germline and somatic structural variants (SVs) in tumor samples that lack a matched normal sample. In this study, we analyzed several features of germline and somatic SVs from a cohort of 974 patients from The Cancer Genome Atlas (TCGA). We identified a total of 21 features that differed significantly between germline and somatic SVs. Several of the germline SV features were associated with each other, as were several of the somatic SV features. We also found that these associations differed between the germline and somatic classes, for example, we found that somatic inversions were more likely to be longer events than their germline counterparts. Using these features we trained a support vector machine (SVM) classifier on 555,849 TCGA SVs to computationally distinguish germline from somatic SVs in the absence of a matched normal. This classifier had an ROC curve AUC of 0.984 when tested on an independent test set of 277,925 TCGA SVs. In this dataset, we achieved a positive predictive value (PPV) of 0.81 for an SV called somatic by the classifier being truly somatic. We further tested the classifier on a separate set of 7,623 SVs from pediatric high-grade gliomas (pHGG). In this non-TCGA cohort, our classifier achieved a PPV of 0.828, showing robust performance across datasets.
### Competing Interest Statement
The authors have declared no competing interest.
更多查看译文
关键词
germline filter,tumor samples,structural variant,context-based
AI 理解论文
溯源树
样例
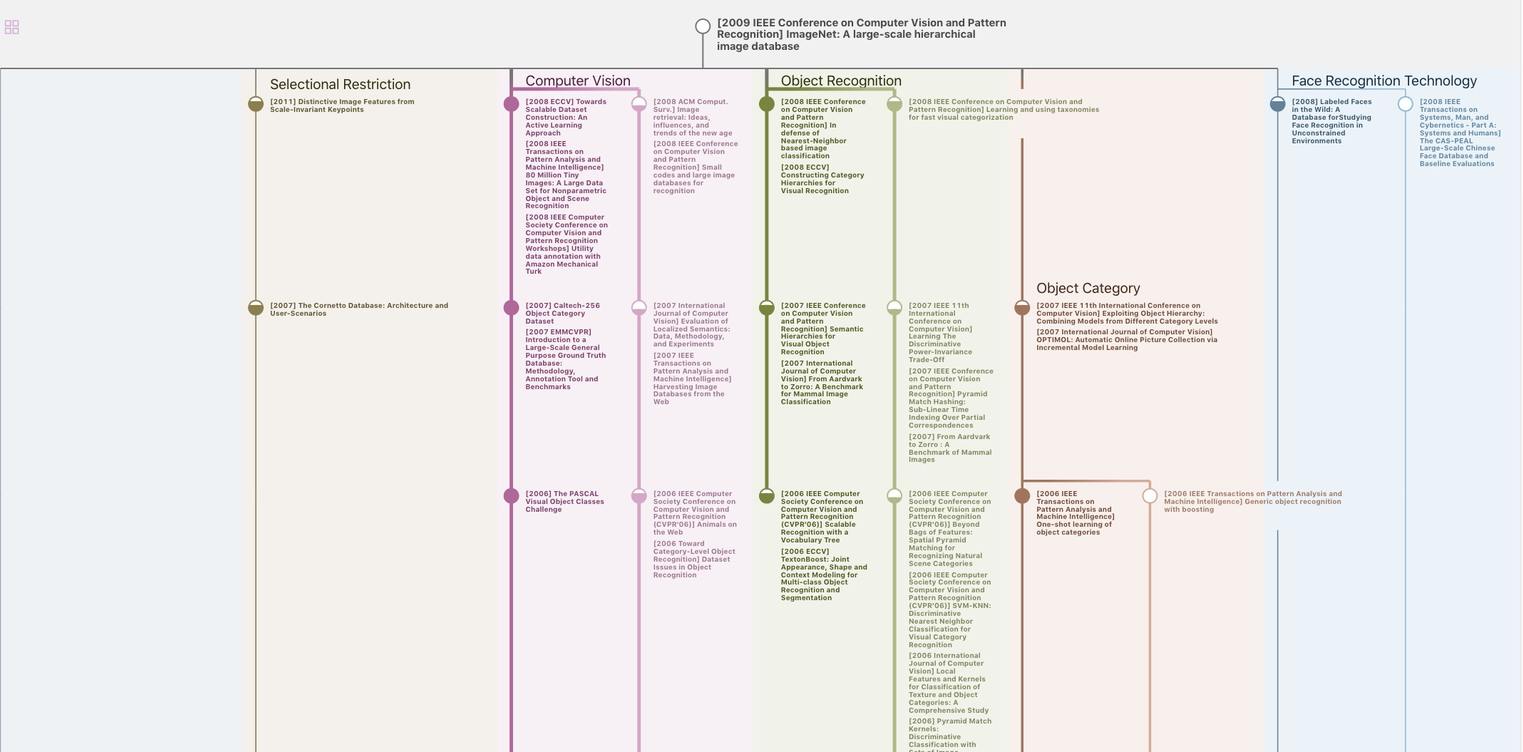
生成溯源树,研究论文发展脉络
Chat Paper
正在生成论文摘要