A Dementia Diagnosis Technique Based on AI and Hardware Acceleration
2023 IEEE International Conference on Artificial Intelligence, Blockchain, and Internet of Things (AIBThings)(2023)
摘要
The effects of Alzheimer’s disease (AD) are devastating, both personally and within the patient’s family, as the disease progresses slowly over many years. It could significantly affect illness consequences and outcomes if detected early and accurately. Non-invasive medical testing often uses blood bio-samples because they are easy to collect and relatively cheap to analyze. This paper proposes a federated learning (FL)-based diagnostic model for AD using blood bio-samples. We have used blood bio-sample data sets downloaded from the ADNI website to test and compare the efficacy of our models. According to the enormous data collected for early AD detection, we used a hardware acceleration scheme to implement our FL model to fasten the training and testing operations. The hardware-accelerator method is implemented using VHDL and Altera 10 GX FPGA. The simulation results show the proposed algorithms reach an accuracy and sensitivity of 89% and 87%, respectively, for early detection while taking less time to train than other state-of-the-art algorithms. The proposed algorithms also consume 52-172 mW, which makes them suitable for constrained devices.
更多查看译文
关键词
Alzheimer’s disease,hardware acceleration,blood bio-samples,federated learning,early diagnosis
AI 理解论文
溯源树
样例
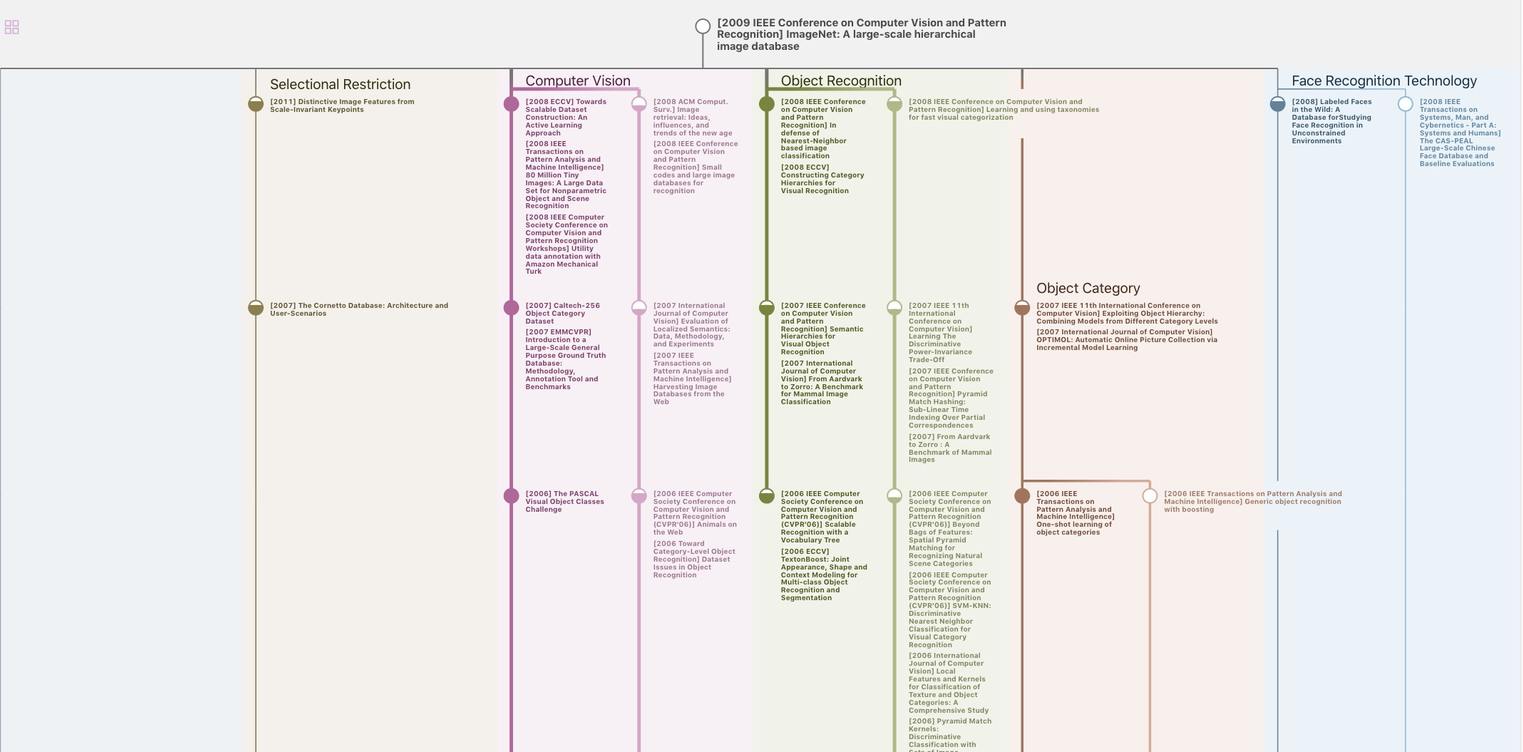
生成溯源树,研究论文发展脉络
Chat Paper
正在生成论文摘要