IoT Edge-based Machine Learning Approach for Detection of Partial Discharge in Power Transformers
2023 IEEE International Conference on Artificial Intelligence, Blockchain, and Internet of Things (AIBThings)(2023)
摘要
The growth of the global economy has fuelled the need for electrical energy. This increased energy demand from 122,745 terawatt-hours in 2000 to 167,781 terawatt-hours in 2020 meant that power systems and power components, e.g., power transformers, must always remain operational. The power transformers are expensive and critical assets in the power supply logistics. Recently, the introduction of solar rooftops has increased the electrical stresses on the power transformers. This paper proposes AI-based models to monitor partial discharge and its likely causes. This study looked at LSTM-GRU and Attention-based Transformer models to detect partial discharges and recommend the likely causes for the partial discharge. The study looked at single-attention layer and dual-attention layer transformers and found very little difference in performance between the two for this use case. The LSTM-GRU option also provided good results, although sensitivity was inferior to the transformer models. This research study is of immediate benefit to a utility as the proposed model can work as a recommendation engine. The proposed model has been used at a power utility successfully.
更多查看译文
关键词
Industrial IoT,IoT Edge,Partial discharge,Attentionbased learning,Power Transformer,Realtime sensor data
AI 理解论文
溯源树
样例
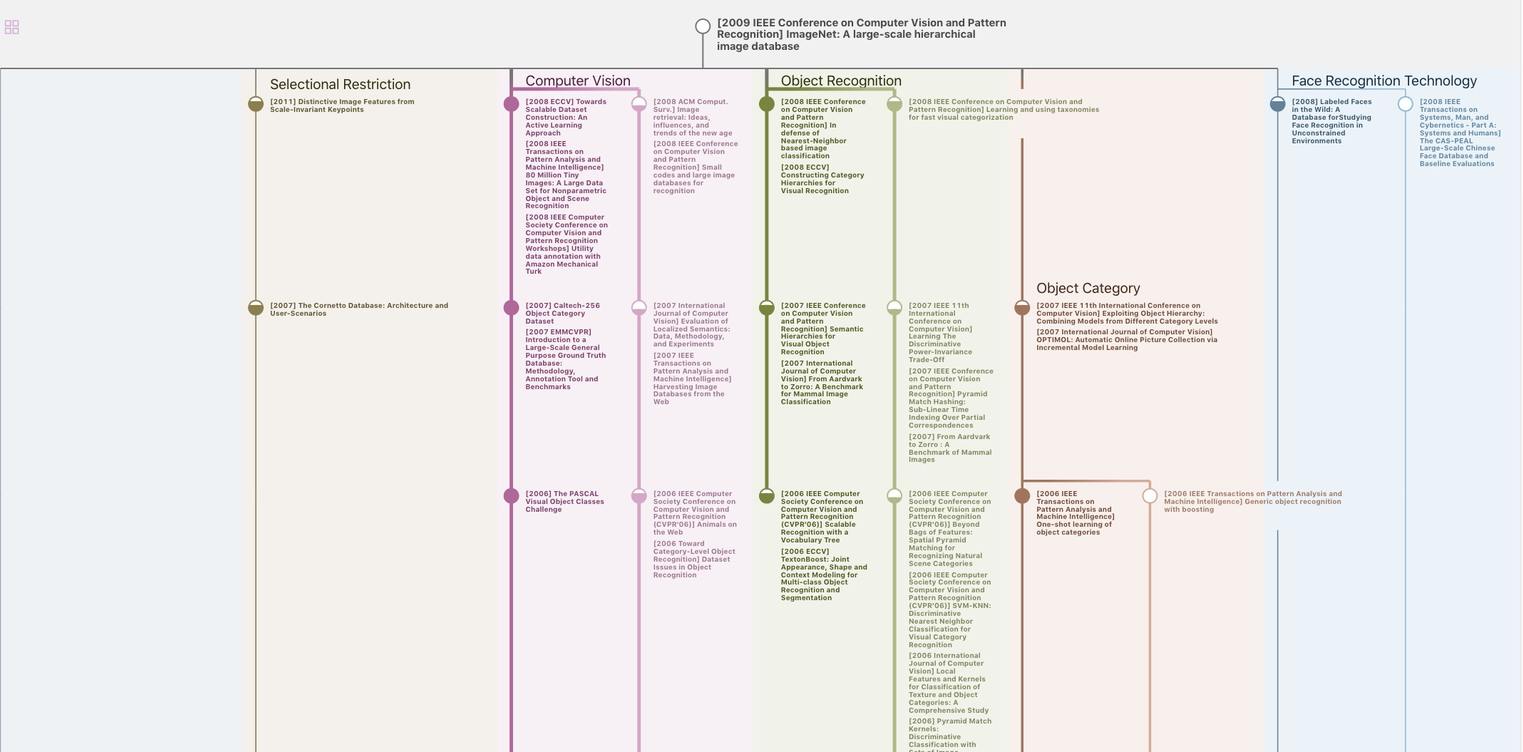
生成溯源树,研究论文发展脉络
Chat Paper
正在生成论文摘要