Abnormal Behavior Recognition Based on Skeleton Key Points
2023 IEEE 7th Information Technology and Mechatronics Engineering Conference (ITOEC)(2023)
摘要
Being aimed at the problem that most of the graph convolutions do not make full use of the context information of the skeleton data, resulting in the low accuracy of similar abnormal line recognition, this paper proposes an improved adaptive graph convolution algorithm for abnormal behavior recognition, which introduces multi-head attention on the basis of adaptive graph convolution. In addition, in order to extract multi-scale features in the network more effectively, multi-scale features in time series are extracted with the help of hole convolution with different expansion coefficients, and multi-scale features are adaptively fused through the attention mechanism. Experimental results show that the multi-head attention adaptive graph convolutional network achieves 76.0% accuracy in abnormal behavior recognition.
更多查看译文
AI 理解论文
溯源树
样例
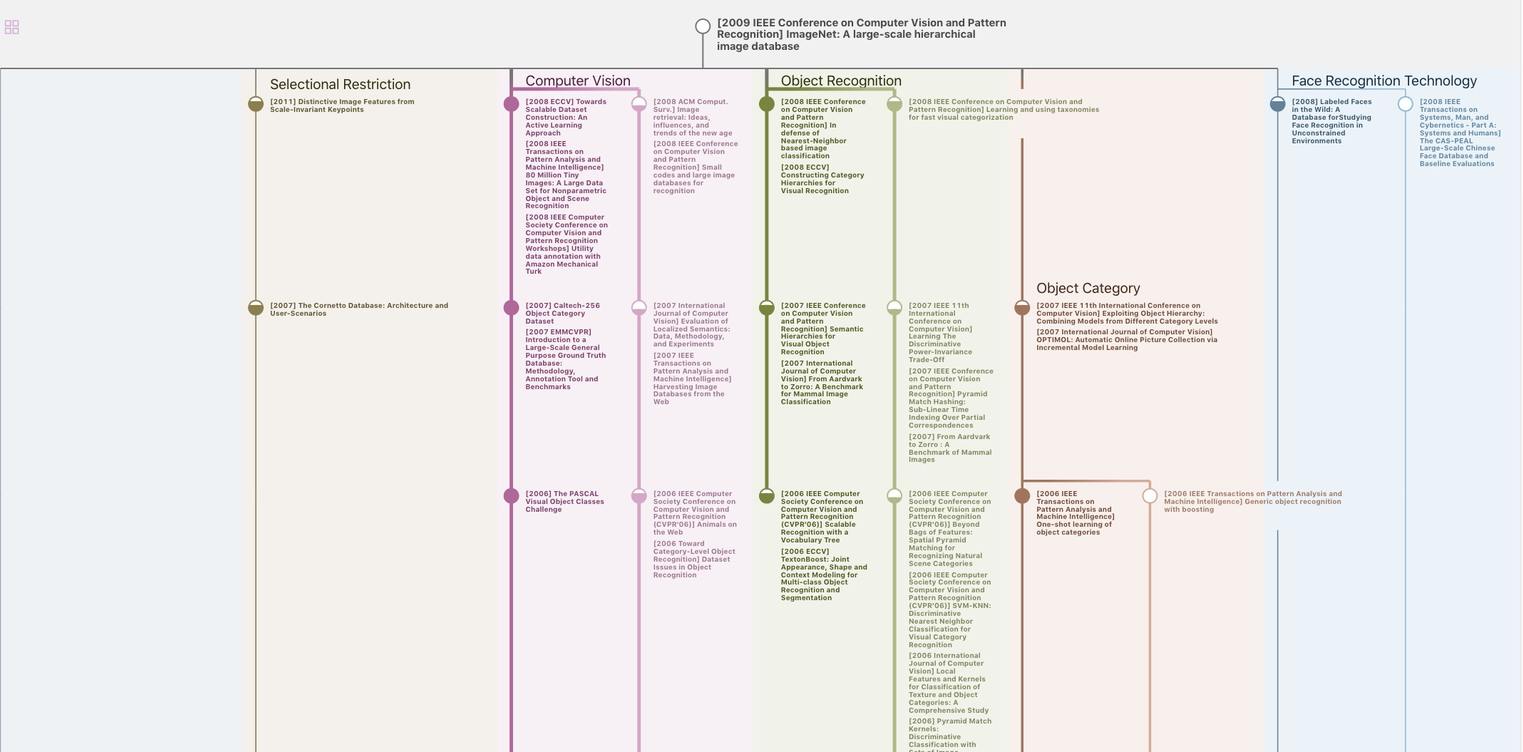
生成溯源树,研究论文发展脉络
Chat Paper
正在生成论文摘要