On learning distribution alignment for video-based visible-infrared person re-identification
Computer Vision and Image Understanding(2023)
摘要
This paper studies the matching problem of cross-modality video data from a discrete distribution alignment view. Central to this discussion is the visible-infrared person re-identification (VI-reID), a crucial feature that bolsters surveillance systems’ efficacy in monitoring individuals across diverse lighting conditions. Going beyond traditional image-to-image matching paradigms, a recent study shows that temporal information can bring richer cues to encode the pedestrian representation, improving the representation power of deep neural networks. However, this integration further complicates cross-modality data matching due to the joint processing of spatial and temporal information. This paper formulates the video data as a discrete distribution and aligns the cross-modality video representation by reducing the matching cost between the two distributions. To this end, a natural idea for aligning the videos is to reduce the divergence of distributions. Moreover, the powerful optimal transport (OT) scheme, which generates the optimal matching flows and establishes the relevance of two distributions, is also employed as a way to measure the distance of distributions. Nevertheless, we observe that endowing the OT in the advanced VI-reID feature extractor leads to a non-symmetric measurement. To mitigate this, the paper introduces a new metric, namely symmetric optimal transport (SOT), reformulating OT into a symmetric form. Thorough analyses and empirical studies affirm the superiority of the proposed SOT, which significantly outperforms the current state-of-the-art methods according to standard benchmarking evaluations.
更多查看译文
关键词
41A05,41A10,65D05,65D17,Visible-infrared person re-identification,Video,Discrete distribution,Optimal transport,Symmetric optimal transport
AI 理解论文
溯源树
样例
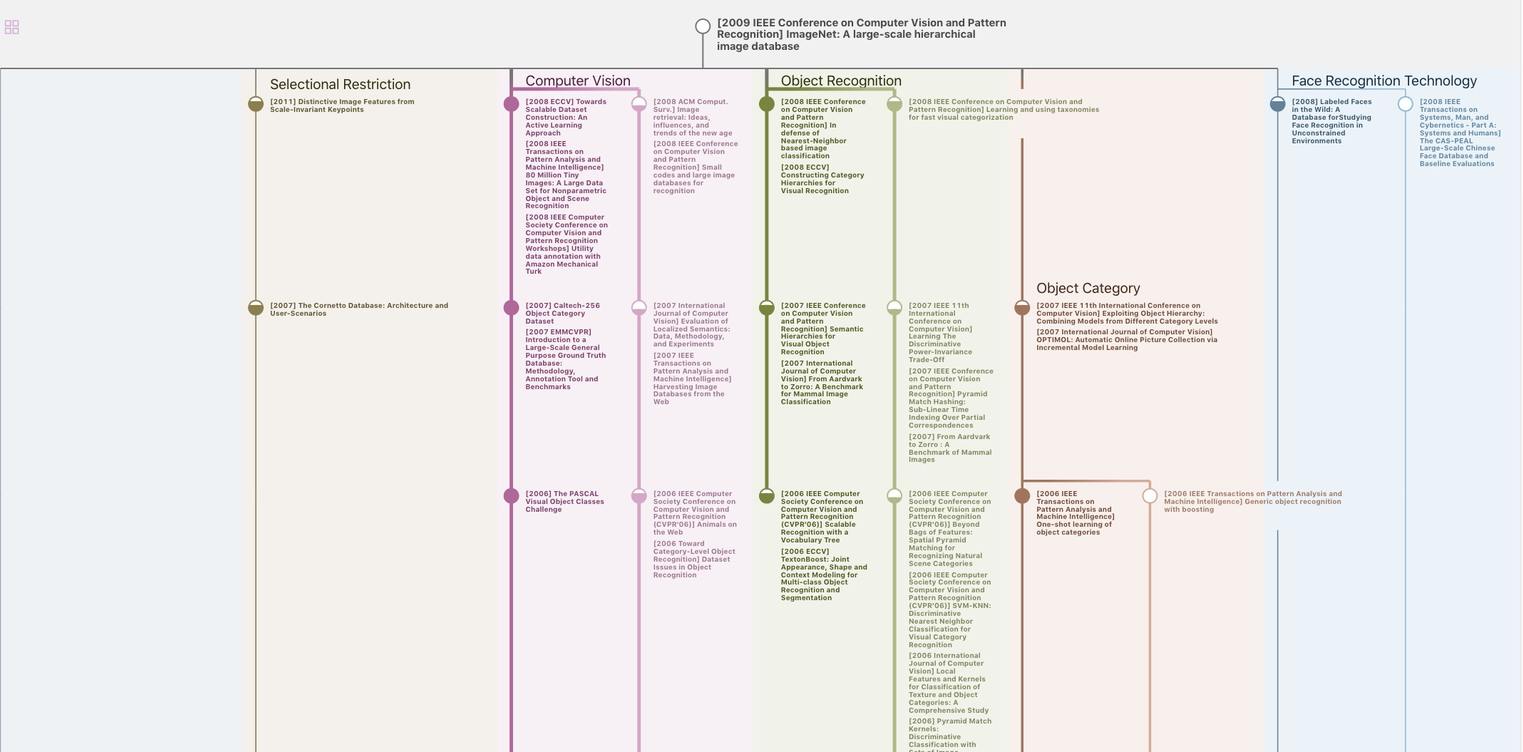
生成溯源树,研究论文发展脉络
Chat Paper
正在生成论文摘要