The Utility of "Even if..." Semifactual Explanation to Optimise Positive Outcomes
CoRR(2023)
摘要
When users receive either a positive or negative outcome from an automated system, Explainable AI (XAI) has almost exclusively focused on how to mutate negative outcomes into positive ones by crossing a decision boundary using counterfactuals (e.g., \textit{"If you earn 2k more, we will accept your loan application"}). Here, we instead focus on \textit{positive} outcomes, and take the novel step of using XAI to optimise them (e.g., \textit{"Even if you wish to half your down-payment, we will still accept your loan application"}). Explanations such as these that employ "even if..." reasoning, and do not cross a decision boundary, are known as semifactuals. To instantiate semifactuals in this context, we introduce the concept of \textit{Gain} (i.e., how much a user stands to benefit from the explanation), and consider the first causal formalisation of semifactuals. Tests on benchmark datasets show our algorithms are better at maximising gain compared to prior work, and that causality is important in the process. Most importantly however, a user study supports our main hypothesis by showing people find semifactual explanations more useful than counterfactuals when they receive the positive outcome of a loan acceptance.
更多查看译文
关键词
semifactual explanation,optimise positive
AI 理解论文
溯源树
样例
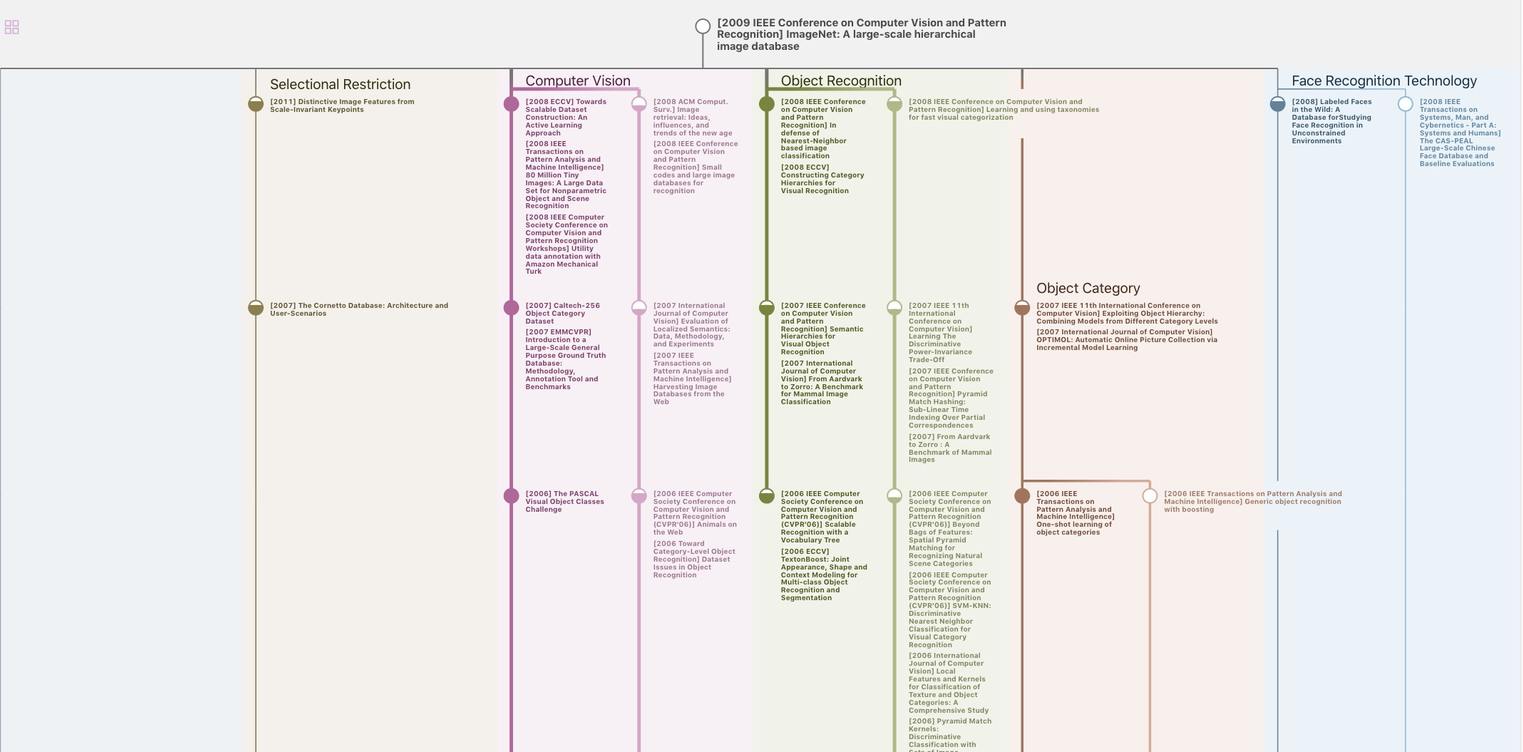
生成溯源树,研究论文发展脉络
Chat Paper
正在生成论文摘要