A Zeroth-Order Variance-Reduced Method for Decentralized Stochastic Non-convex Optimization
arXiv (Cornell University)(2023)
摘要
In this paper, we consider a distributed stochastic non-convex optimization problem, which is about minimizing a sum of $n$ local cost functions over a network with only zeroth-order information. A novel single-loop Decentralized Zeroth-Order Variance Reduction algorithm, called DZOVR, is proposed, which combines two-point gradient estimation, momentum-based variance reduction technique, and gradient tracking. Under mild assumptions, we show that the algorithm is able to achieve $\mathcal{O}(dn^{-1}\epsilon^{-3})$ sampling complexity at each node to reach an $\epsilon$-accurate stationary point and also exhibits network-independent and linear speedup properties. To the best of our knowledge, this is the first stochastic decentralized zeroth-order algorithm that achieves this sampling complexity. Numerical experiments demonstrate that DZOVR outperforms the other state-of-the-art algorithms and has network-independent and linear speedup properties.
更多查看译文
关键词
decentralized stochastic
AI 理解论文
溯源树
样例
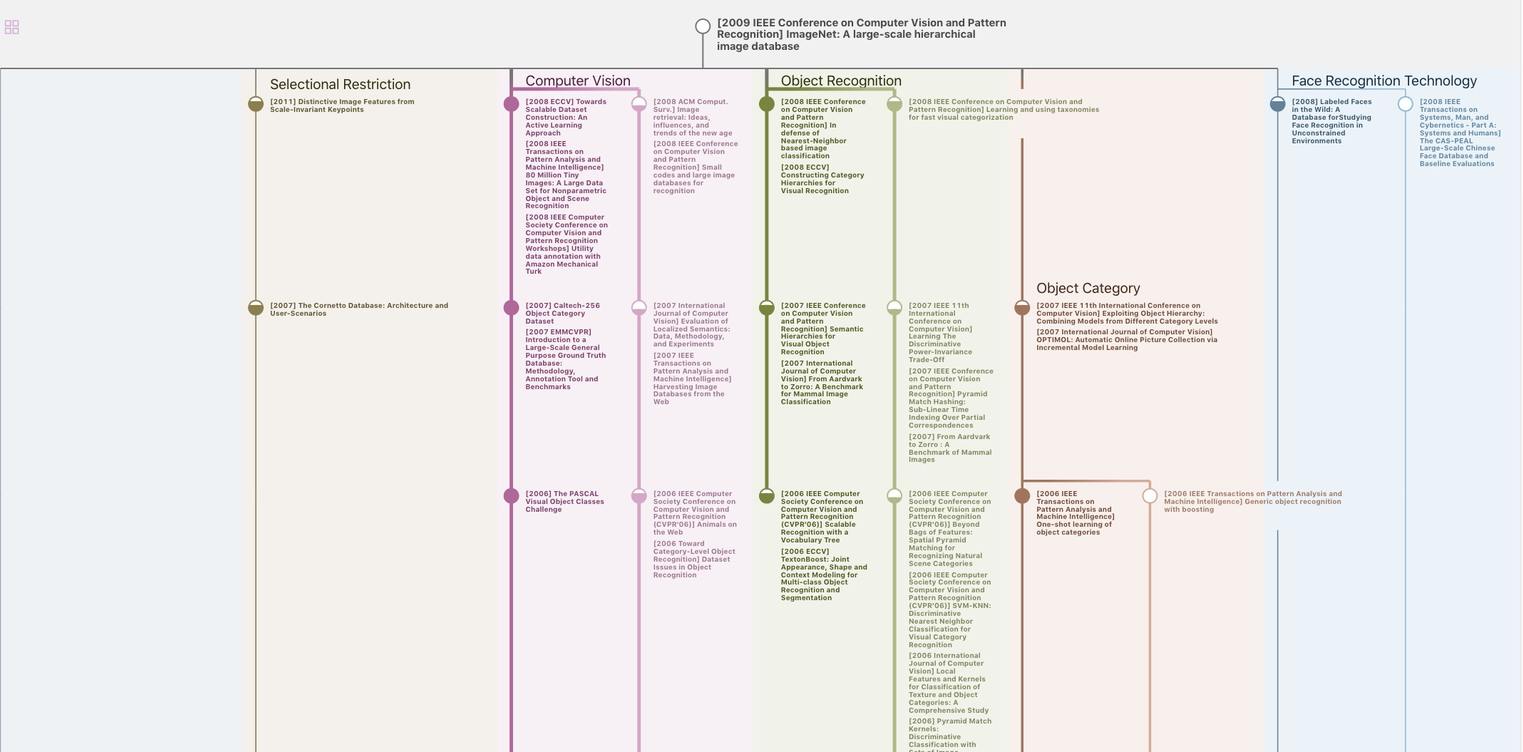
生成溯源树,研究论文发展脉络
Chat Paper
正在生成论文摘要