Where have you been? A Study of Privacy Risk for Point-of-Interest Recommendation
CoRR(2023)
摘要
As location-based services (LBS) have grown in popularity, the collection of human mobility data has become increasingly extensive to build machine learning (ML) models offering enhanced convenience to LBS users. However, the convenience comes with the risk of privacy leakage since this type of data might contain sensitive information related to user identities, such as home/work locations. Prior work focuses on protecting mobility data privacy during transmission or prior to release, lacking the privacy risk evaluation of mobility data-based ML models. To better understand and quantify the privacy leakage in mobility data-based ML models, we design a privacy attack suite containing data extraction and membership inference attacks tailored for point-of-interest (POI) recommendation models, one of the most widely used mobility data-based ML models. These attacks in our attack suite assume different adversary knowledge and aim to extract different types of sensitive information from mobility data, providing a holistic privacy risk assessment for POI recommendation models. Our experimental evaluation using two real-world mobility datasets demonstrates that current POI recommendation models are vulnerable to our attacks. We also present unique findings to understand what types of mobility data are more susceptible to privacy attacks. Finally, we evaluate defenses against these attacks and highlight future directions and challenges.
更多查看译文
关键词
privacy risk,recommendation,point-of-interest
AI 理解论文
溯源树
样例
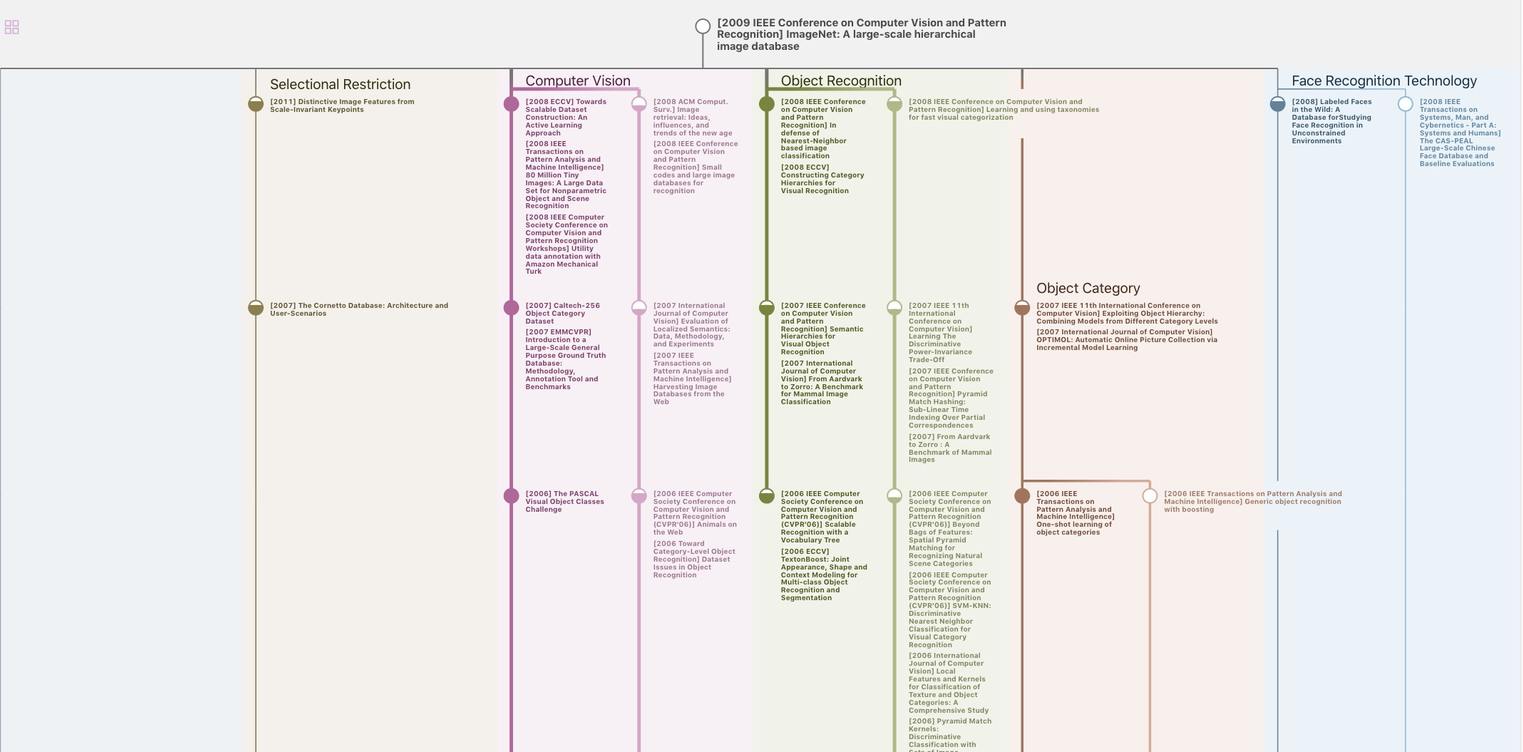
生成溯源树,研究论文发展脉络
Chat Paper
正在生成论文摘要