Efficient Fully Bayesian Approach to Brain Activity Mapping with Complex-Valued fMRI Data
arXiv (Cornell University)(2023)
摘要
Functional magnetic resonance imaging (fMRI) enables indirect detection of brain activity changes via the blood-oxygen-level-dependent (BOLD) signal. Conventional analysis methods mainly rely on the real-valued magnitude of these signals. In contrast, research suggests that analyzing both real and imaginary components of the complex-valued fMRI (cv-fMRI) signal provides a more holistic approach that can increase power to detect neuronal activation. We propose a fully Bayesian model for brain activity mapping with cv-fMRI data. Our model accommodates temporal and spatial dynamics. Additionally, we propose a computationally efficient sampling algorithm, which enhances processing speed through image partitioning. Our approach is shown to be computationally efficient via image partitioning and parallel computation while being competitive with state-of-the-art methods. We support these claims with both simulated numerical studies and an application to real cv-fMRI data obtained from a finger-tapping experiment.
更多查看译文
关键词
brain activity mapping,fmri data,complex-valued
AI 理解论文
溯源树
样例
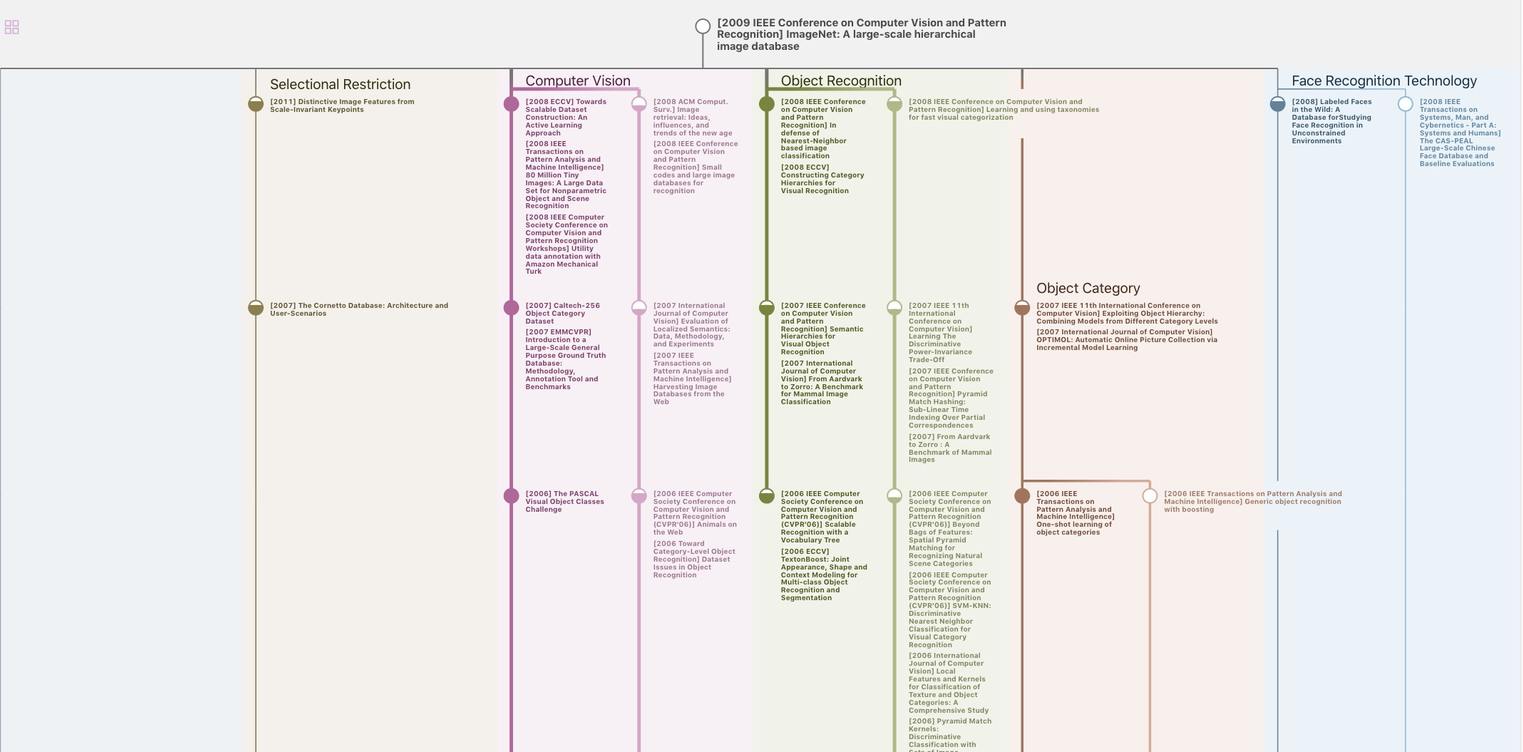
生成溯源树,研究论文发展脉络
Chat Paper
正在生成论文摘要