Sample based Explanations via Generalized Representers
NeurIPS(2023)
摘要
We propose a general class of sample based explanations of machine learning models, which we term generalized representers. To measure the effect of a training sample on a model's test prediction, generalized representers use two components: a global sample importance that quantifies the importance of the training point to the model and is invariant to test samples, and a local sample importance that measures similarity between the training sample and the test point with a kernel. A key contribution of the paper is to show that generalized representers are the only class of sample based explanations satisfying a natural set of axiomatic properties. We discuss approaches to extract global importances given a kernel, and also natural choices of kernels given modern non-linear models. As we show, many popular existing sample based explanations could be cast as generalized representers with particular choices of kernels and approaches to extract global importances. Additionally, we conduct empirical comparisons of different generalized representers on two image and two text classification datasets.
更多查看译文
关键词
explanations,sample
AI 理解论文
溯源树
样例
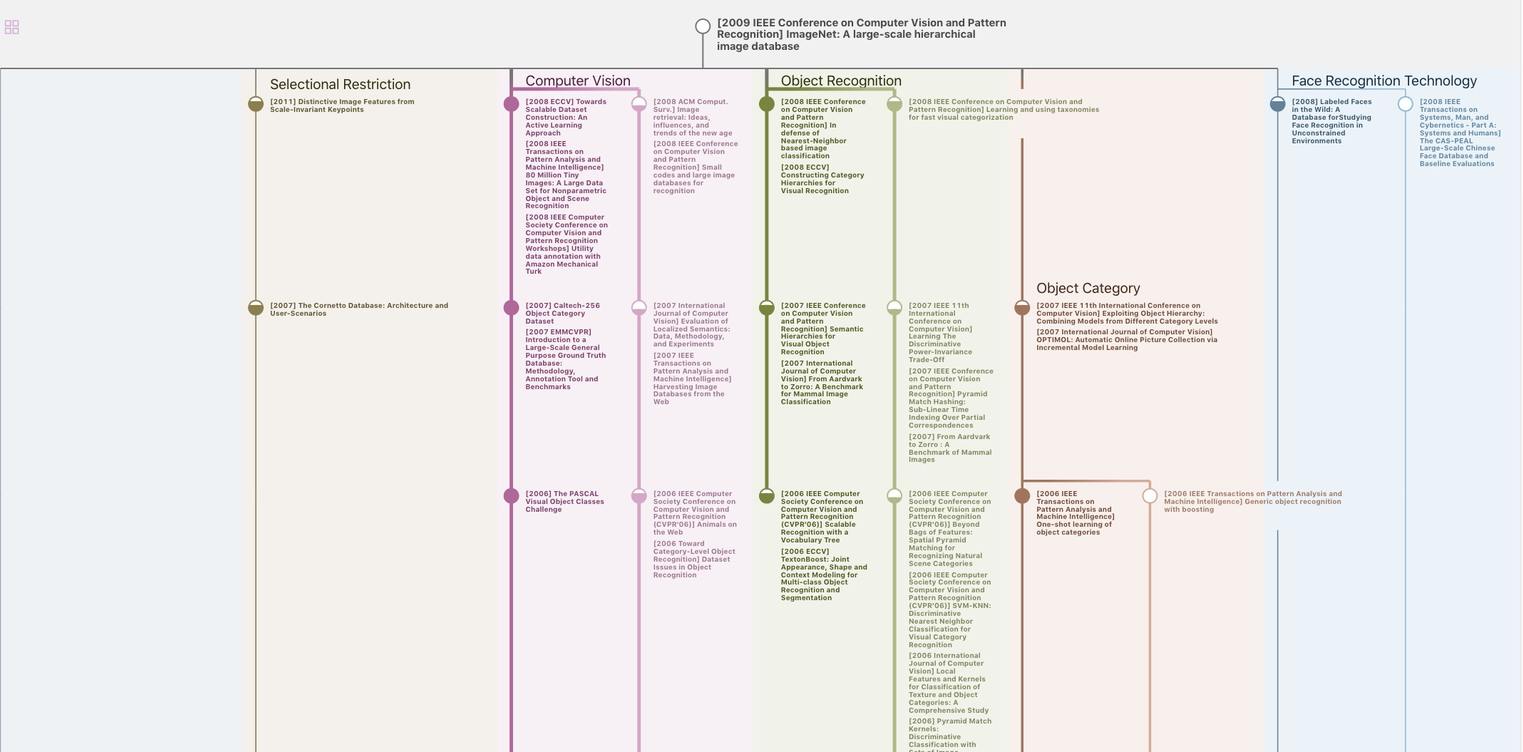
生成溯源树,研究论文发展脉络
Chat Paper
正在生成论文摘要