LoRAShear: Efficient Large Language Model Structured Pruning and Knowledge Recovery
CoRR(2023)
摘要
Large Language Models (LLMs) have transformed the landscape of artificial intelligence, while their enormous size presents significant challenges in terms of computational costs. We introduce LoRAShear, a novel efficient approach to structurally prune LLMs and recover knowledge. Given general LLMs, LoRAShear first creates the dependency graphs to discover minimally removal structures and analyze the knowledge distribution. It then proceeds progressive structured pruning on LoRA adaptors and enables inherent knowledge transfer to better preserve the information in the redundant structures. To recover the lost knowledge during pruning, LoRAShear meticulously studies and proposes a dynamic fine-tuning schemes with dynamic data adaptors to effectively narrow down the performance gap to the full models. Numerical results demonstrate that by only using one GPU within a couple of GPU days, LoRAShear effectively reduced footprint of LLMs by 20% with only 1.0% performance degradation and significantly outperforms state-of-the-arts. The source code will be available at https://github.com/microsoft/lorashear.
更多查看译文
关键词
knowledge recovery,structured,language
AI 理解论文
溯源树
样例
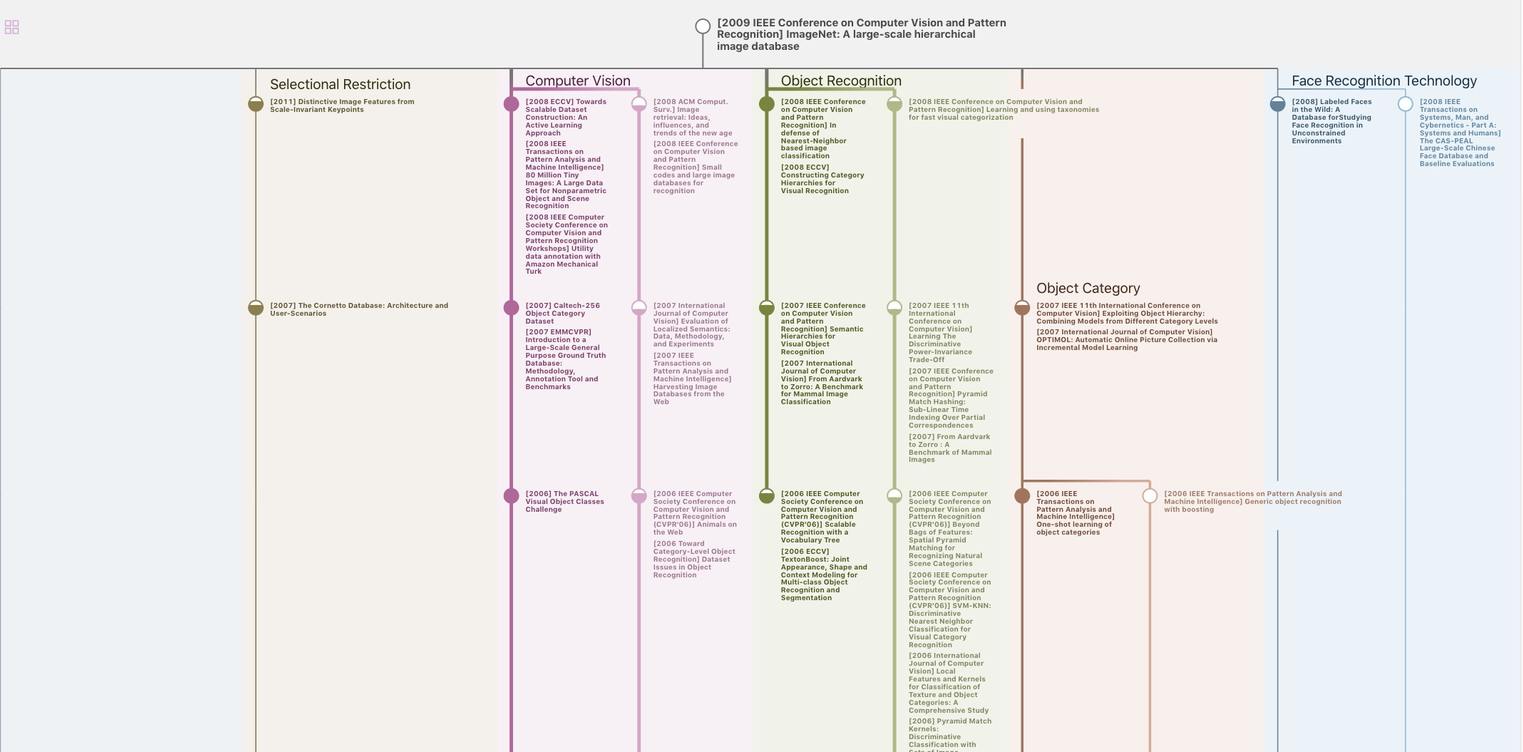
生成溯源树,研究论文发展脉络
Chat Paper
正在生成论文摘要