Domain-Specific Entity Recognition as Token-Pair Relation Classification
IEEE Access(2023)
摘要
Named Entity Recognition (NER) is a fundamental but crucial task in natural language understanding, aiming at identifying entity mentions from free text. Current methods mainly use sequence-labeling and span-based models, where the former ignores the importance of token interaction, and the latter pays little attention to the global inter-dependency among entity tokens. In this work, we propose a novel NER model that consists of two branches: a Token-Pair Interaction Module (TPIM) and a U-shaped Network. The TPIM models head-tail relations between token pairs while capturing intrinsic token connectivity within entity boundaries. The U-shaped Network is employed to capture the contextual dependency in the token-pair relation matrix. Furthermore, we build a typical domain-specific entity dataset CCAEE based on real-world applications in the chemical accident domain. The experimental results on CCAEE and CLUENER datasets demonstrate the effectiveness of our proposed model.
更多查看译文
关键词
Natural language understanding,information extraction,named entity recognition,boundary detection
AI 理解论文
溯源树
样例
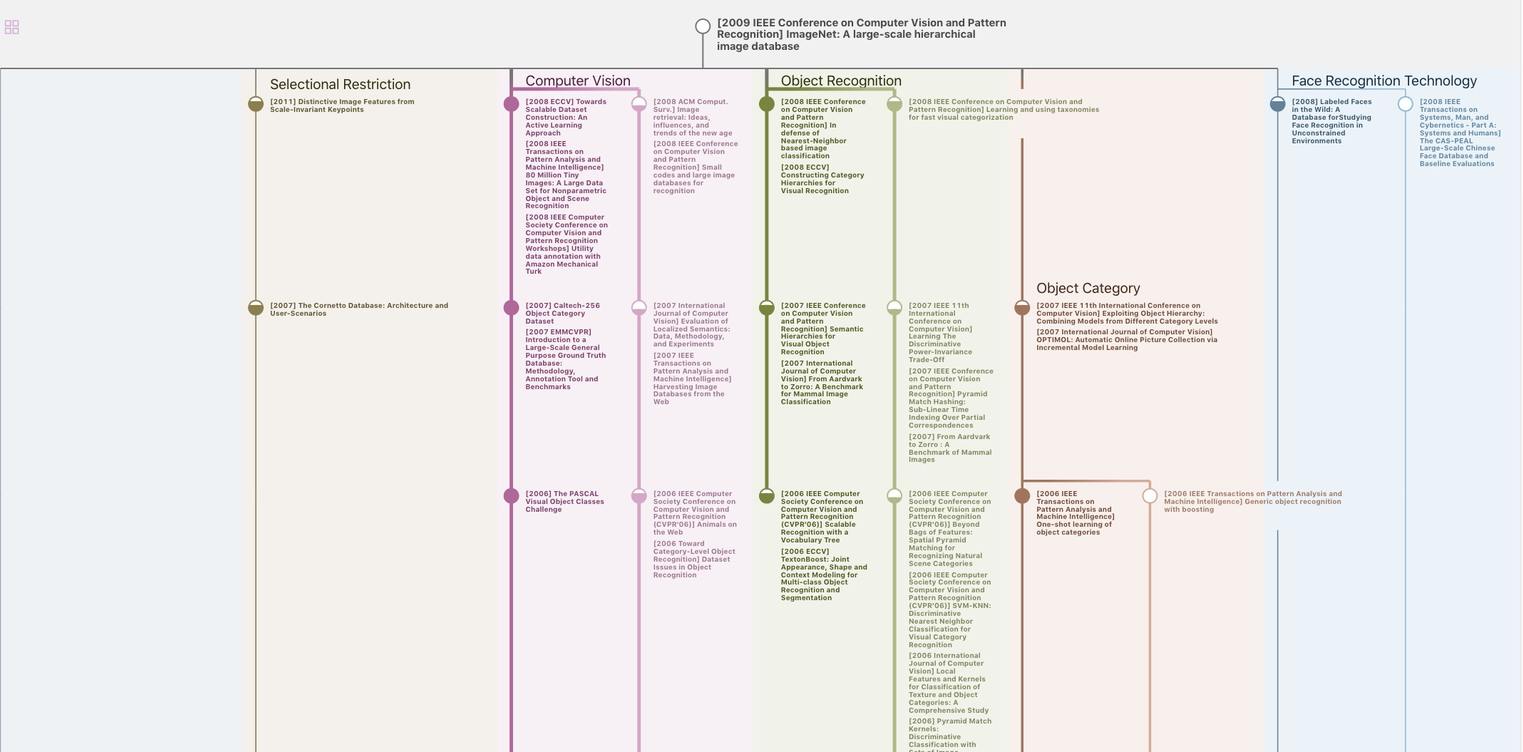
生成溯源树,研究论文发展脉络
Chat Paper
正在生成论文摘要