Accelerating AI performance with the incorporation of TVM and MediaTek NeuroPilot
Connection Science(2023)
摘要
The continuing prominence of machine learning has led to an increased focus on enhancing the inference performance of edge devices to reduce latency and improve efficiency. Two widely adopted strategies for accelerating computational performance are quantisation and the utilisation of AI hardware accelerators. Each type of accelerator or inference engine offers distinct advantages, with accelerators primarily designed to optimise neural network operations. In this paper, we present an innovative method for integrating TVM's quantisation flow with the MediaTek Neuropilot AI accelerator. We outline the process of converting the TVM relay intermediate-representation quantised neural network dialect model to a tensor-oriented quantisation format, with the aim of harnessing the full potential of both TVM and MediaTek NeuroPilot. This integration enables more efficient neural network inference while preserving the accuracy of the results. We assessed the effectiveness of our proposed integration by conducting a series of experiments and comparing the performance of our approach with that of TVM equipped with an autotuning mechanism. The findings indicate that our approach substantially outperforms TVM in both floating-point model inference and quantised model inference, with inference speedups of up to 11x and up to 70x, respectively. These results underscore the potential of our approach in accelerating AI performance across a diverse range of applications and edge devices. Moreover, a key contribution of our work is providing a valuable practical method for other hardware companies interested in integrating TVM with their own accelerators to achieve performance gains.
更多查看译文
关键词
AI,quantisation,heterogeneous computing,compiler
AI 理解论文
溯源树
样例
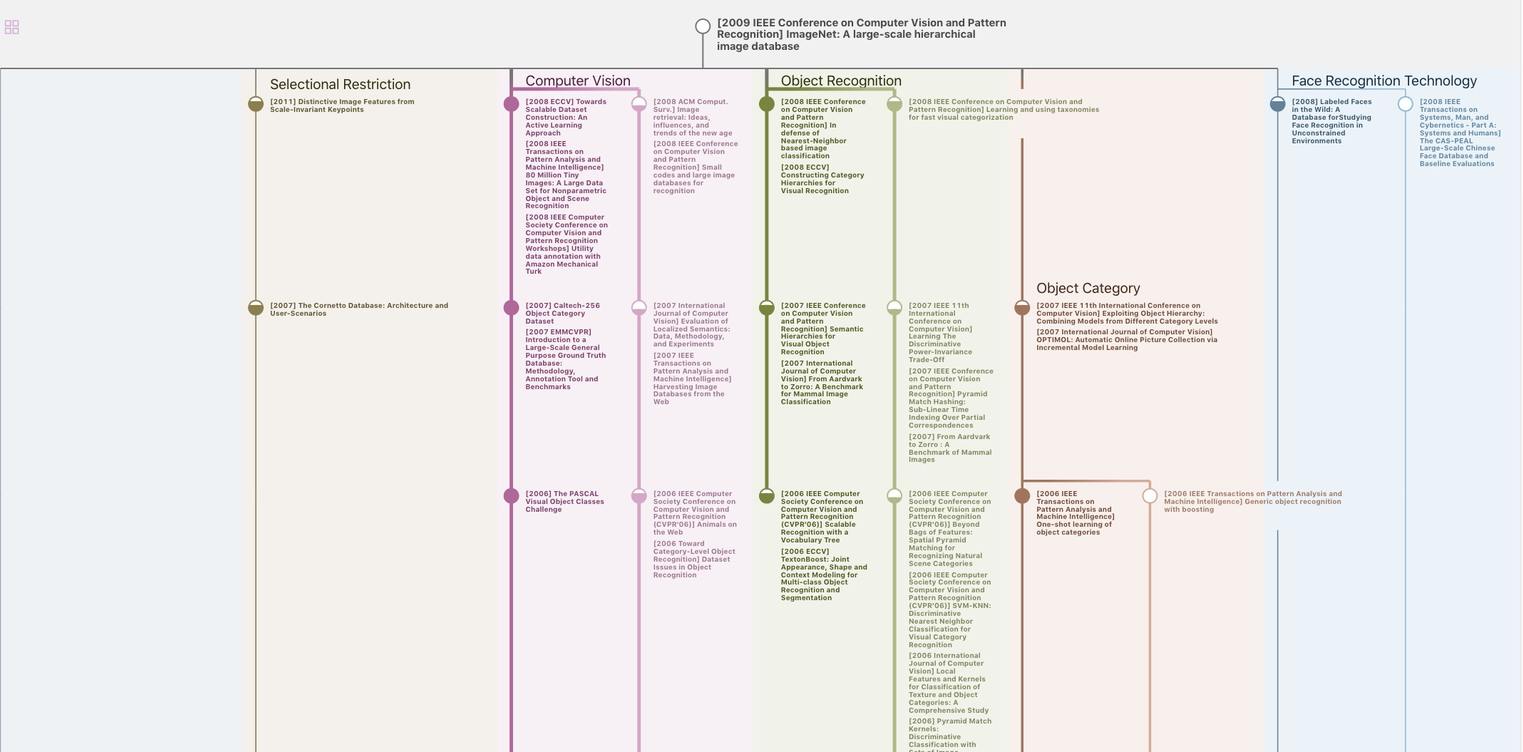
生成溯源树,研究论文发展脉络
Chat Paper
正在生成论文摘要