Support Recovery with Stochastic Gates: Theory and Application for Linear Models
arXiv (Cornell University)(2021)
摘要
Consider the problem of simultaneous estimation and support recovery of the coefficient vector in a linear data model with additive Gaussian noise. We study the problem of estimating the model coefficients based on a recently proposed non-convex regularizer, namely the stochastic gates (STG) [Yamada et al. 2020]. We suggest a new projection-based algorithm for solving the STG regularized minimization problem, and prove convergence and support recovery guarantees of the STG-estimator for a range of random and non-random design matrix setups. Our new algorithm has been shown to outperform the existing STG algorithm and other classical estimators for support recovery in various real and synthetic data analyses.
更多查看译文
关键词
stochastic gates,models,recovery,support
AI 理解论文
溯源树
样例
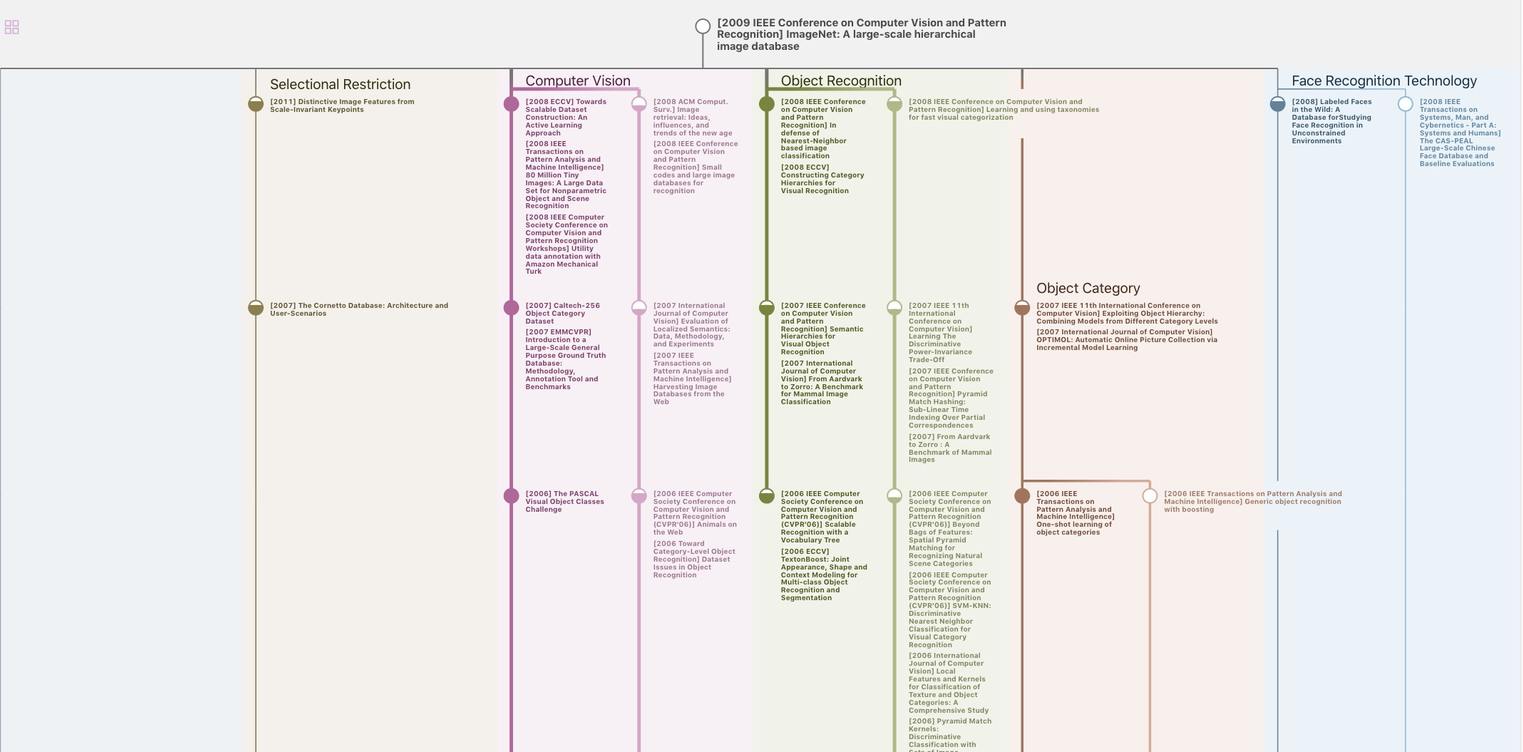
生成溯源树,研究论文发展脉络
Chat Paper
正在生成论文摘要