XGAN: Unsupervised Image-to-Image Translation for Many-to-Many Mappings
Springer eBooks(2020)
摘要
Image translation refers to the task of mapping images from a visual domain to another. Given two unpaired collections of images, we aim to learn a mapping between the corpus-level style of each collection, while preserving semantic content shared across the two domains. We introduce xgan, a dual adversarial auto-encoder, which captures a shared representation of the common domain semantic content in an unsupervised way, while jointly learning the domain-to-domain image translations in both directions. We exploit ideas from the domain adaptationDomain adaptation literature and define a semantic consistency loss which encourages the learned embedding to preserve semantics shared across domains. We report promising qualitative results for the task of face-to-cartoon translation. The cartoon dataset we collected for this purpose, “CartoonSet”, is also publicly available as a new benchmark for semantic style transferStyle transfer at https://google.github.io/cartoonset/index.html .
更多查看译文
关键词
mappings,image-to-image,many-to-many
AI 理解论文
溯源树
样例
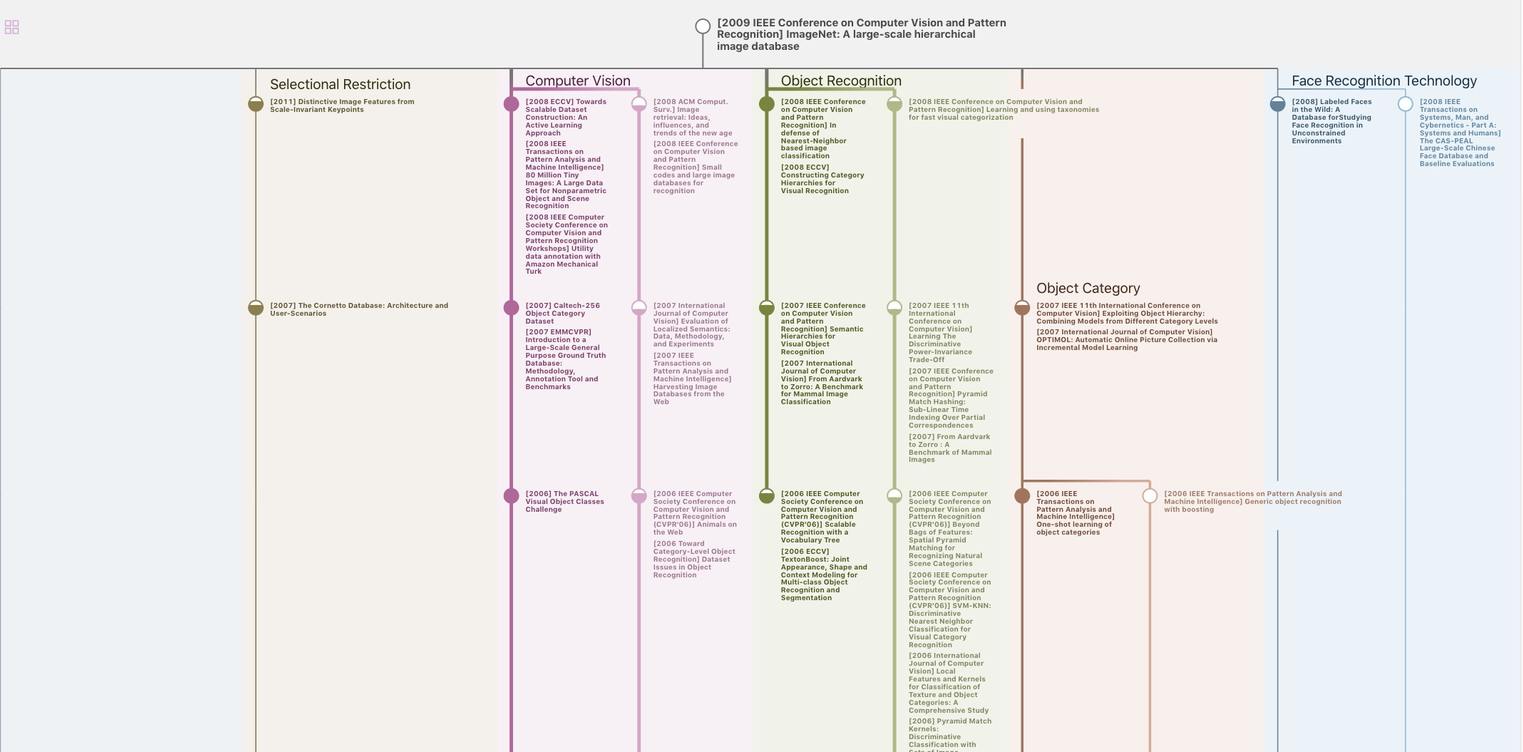
生成溯源树,研究论文发展脉络
Chat Paper
正在生成论文摘要