Multichannel consecutive data cross-extraction with 1DCNN-attention for diagnosis of power transformer
arXiv (Cornell University)(2023)
摘要
Power transformer plays a critical role in grid infrastructure, and its diagnosis is paramount for maintaining stable operation. However, the current methods for transformer diagnosis focus on discrete dissolved gas analysis, neglecting deep feature extraction of multichannel consecutive data. The unutilized sequential data contains the significant temporal information reflecting the transformer condition. In light of this, the structure of multichannel consecutive data cross-extraction (MCDC) is proposed in this article in order to comprehensively exploit the intrinsic characteristic and evaluate the states of transformer. Moreover, for the better accommodation in scenario of transformer diagnosis, one dimensional convolution neural network attention (1DCNN-attention) mechanism is introduced and offers a more efficient solution given the simplified spatial complexity. Finally, the effectiveness of MCDC and the superior generalization ability, compared with other algorithms, are validated in experiments conducted on a dataset collected from real operation cases of power transformer. Additionally, the better stability of 1DCNN-attention has also been certified.
更多查看译文
关键词
power transformer,cross-extraction,dcnn-attention
AI 理解论文
溯源树
样例
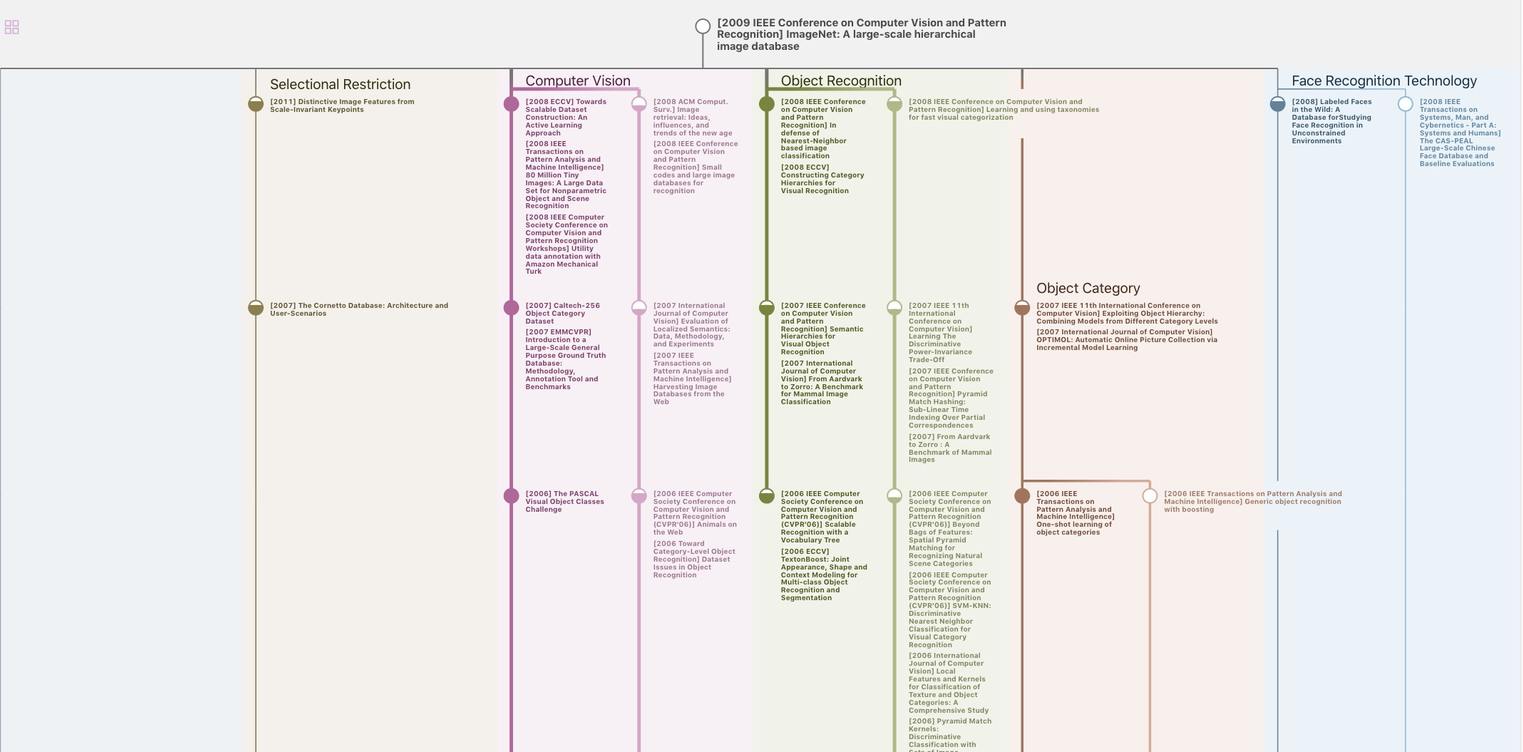
生成溯源树,研究论文发展脉络
Chat Paper
正在生成论文摘要