Real-time water demand pattern estimation using an optimized extended Kalman filter
EXPERT SYSTEMS WITH APPLICATIONS(2024)
摘要
This study presents a hybrid approach for the estimation of real-time water demand multipliers using the Kalman filter (KF) and extended Kalman filter (EKF). Multiple Linear Regression (MLR) and Nonlinear Regression (NLR) models were applied to predict water demand multipliers at each time step with historical flow data. The estimation performance of EKF is highly affected by the state noise covariance matrix (Q) and the measurement noise covariance matrix (R). An inappropriate value of Q and R significantly degrades the EKF's performance and makes the filter diverge. So, the particle swarm optimization algorithm (PSO) was used to tune the noise covariance matrices Q and R at each time step of EKF. Then the optimal values of noise covariance matrices are inserted in the real-time water demand multiplier estimation process. To find the optimal values of Q and R, the mean absolute percentage error (MAPE) between measured and simulated pressure was minimized. The proposed method was evaluated in Net1 and Net3 benchmark networks. The root means square error (RMSE) of EKF-PSO estimated water demand multiplier for Net1 and Net3 were 0.063 and 0.198, respectively. The simulation results indicated that the EKF-PSO algorithm was more accurate than the conventional EKF algorithm. Moreover, the KF-PSO performed poorly when dealing with nonlinear hydraulic systems.
更多查看译文
关键词
Demand multiplier,PSO,Online Calibration,Water Distribution Networks,EPANET
AI 理解论文
溯源树
样例
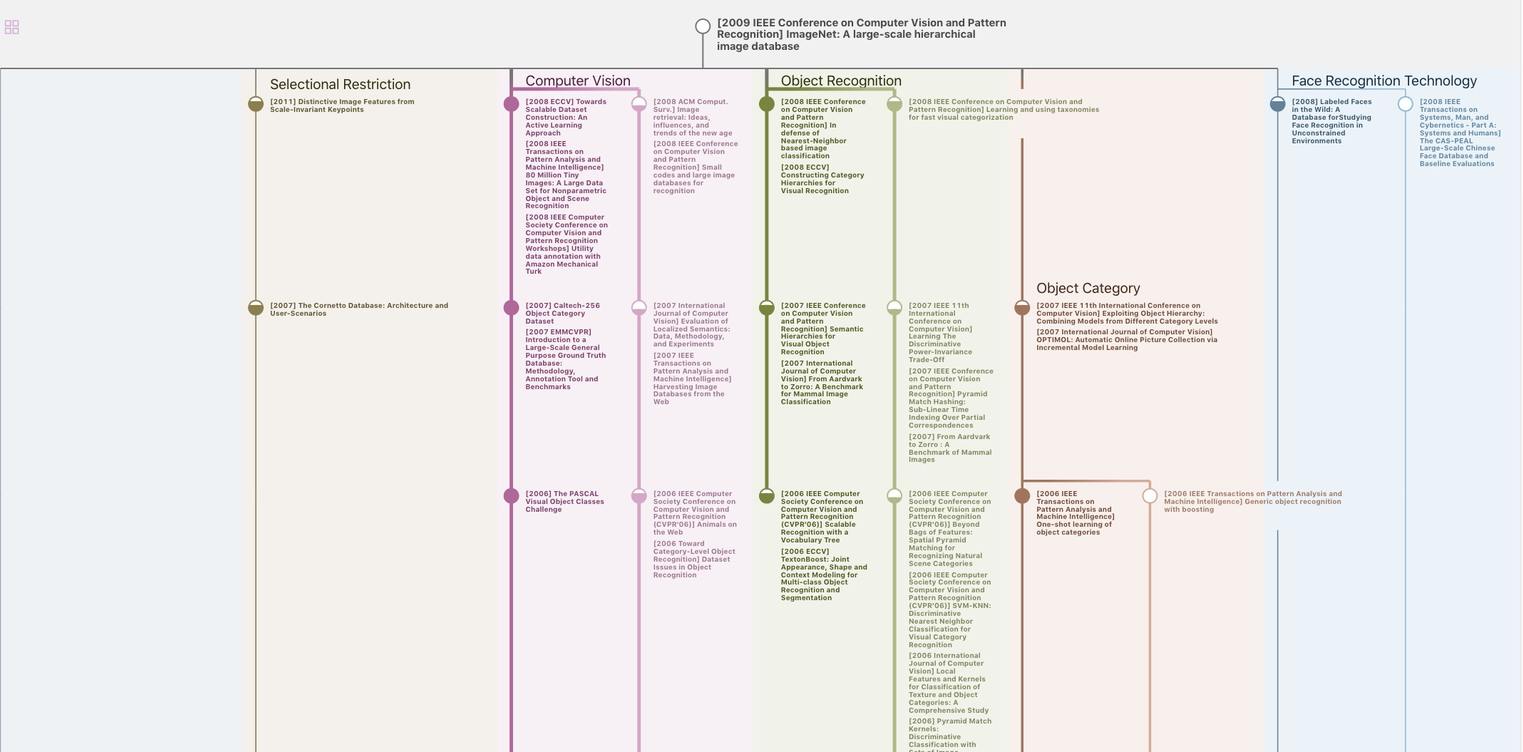
生成溯源树,研究论文发展脉络
Chat Paper
正在生成论文摘要