SemiBuildingChange: A Semi-Supervised High-Resolution Remote Sensing Image Building Change Detection Method With a Pseudo Bitemporal Data Generator
IEEE Transactions on Geoscience and Remote Sensing(2023)
摘要
Remote sensing (RS) image change detection (CD) aims to obtain change information of the target area between multitemporal RS images. With the modernization of cities, building CD (BCD) plays a pivotal role in land resource planning, smart city construction, and natural disaster assessment, and it is a typical application field of CD task. Recently, deep learning (DL)-based methods have shown their superiority in RS image CD. However, the performance of the existing supervised CD methods relies heavily on a large amount of high-quality annotated bitemporal RS image as training data, which is usually hard to obtain in practice. To address this issue, a semi-supervised BCD method using a pseudo bitemporal data (PITA) generator with consistency regularization was proposed. This method only needs a very small amount of single-temporal RS images with building extraction labels as labeled data. First, with the help of the PITA generator, the model can generate a large number of pseudo bitemporal images with CD labels from a small number of single-temporal images and corresponding building extraction labels automatically, which greatly augments the labeled dataset for CD model training. Then, we proposed an error-prone data enhancement fine-tuning strategy to improve the learning effect of the proposed model to these synthesized training data. Finally, we enhance the robustness of the model by forcing the model to make consistent predictions on the images before and after perturbations. Extensive experimental results demonstrate that our method can effectively improve the BCD performance of the model even if labeled data are scare and outperform the state-of-the-art methods.
更多查看译文
关键词
Building change detection (BCD), data augmentation, pseudo bitemporal generator, remote sensing (RS), semi-supervised learning (SSL)
AI 理解论文
溯源树
样例
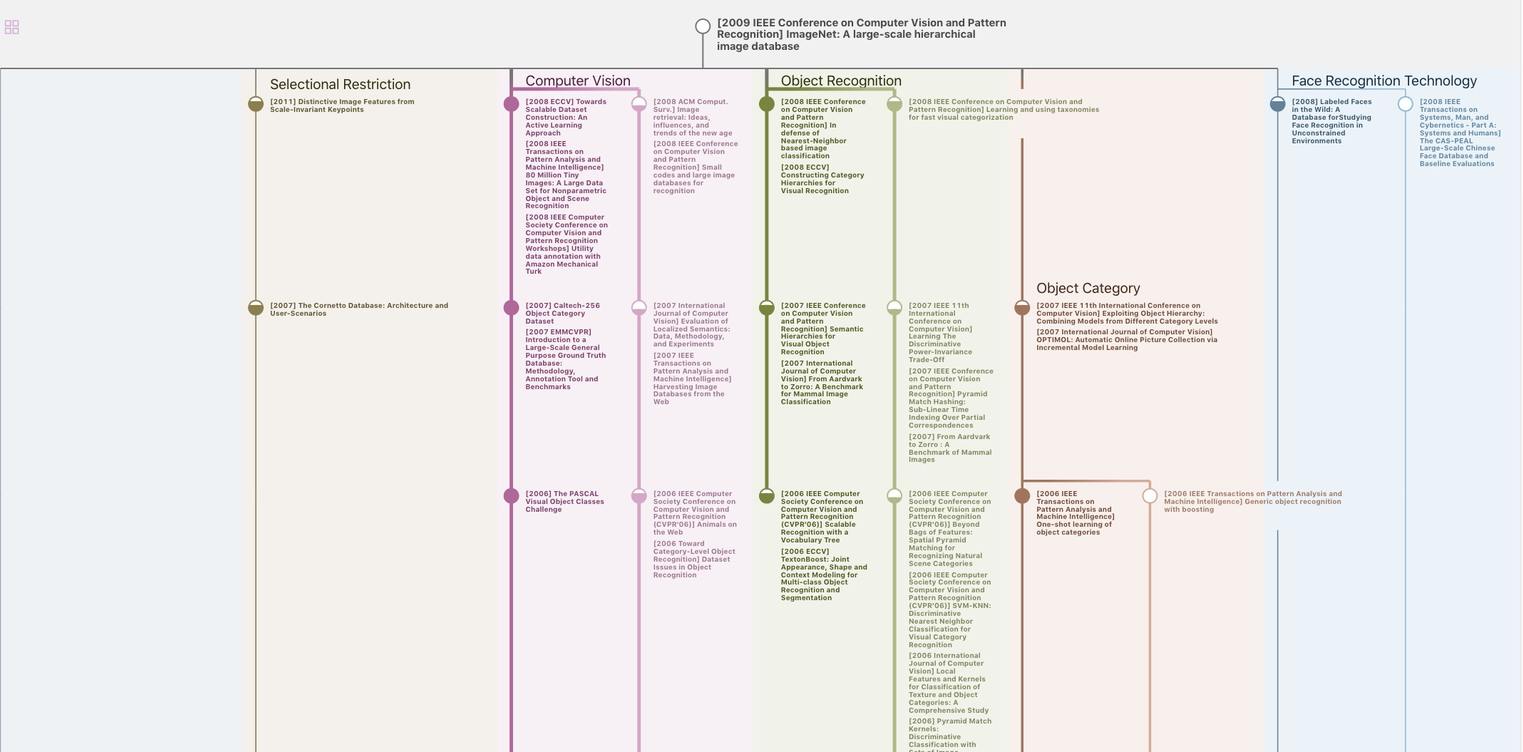
生成溯源树,研究论文发展脉络
Chat Paper
正在生成论文摘要