A Deep Anomaly Detection With Same Probability Distribution and Its Application in Rolling Bearing
JOURNAL OF DYNAMIC SYSTEMS MEASUREMENT AND CONTROL-TRANSACTIONS OF THE ASME(2023)
摘要
An innovative deep-learning-based model, namely, deep anomaly detection with the same probability distribution (DADSPD) is proposed to improve the accuracy of anomaly detection (AD) of rolling bearings driven only by normal data. First, the main framework of feature extraction based on a residual network was established, and a three-layer encoder structure was used to extract multidimensional features. Second, a new loss function based on the same probability distribution is designed, and the function of its probability distribution is to complete the training of the model by calculating the similarity between the outputs. Subsequently, the vibration data were preprocessed using wavelet and envelope analysis, and the processed data are converted into two-dimensional image signals and used as the input of the DADSPD. Finally, the model is verified on three sets of run-to-failure experimental datasets of rolling bearing. The results demonstrate that the proposed DADSPD model reaches more than 99%, which indicates that the DADSPD model has a high fault early warning and AD capability.
更多查看译文
关键词
rolling bearing,anomaly detection,unsupervised learning,autoencoder,multivariate Gaussian distribution
AI 理解论文
溯源树
样例
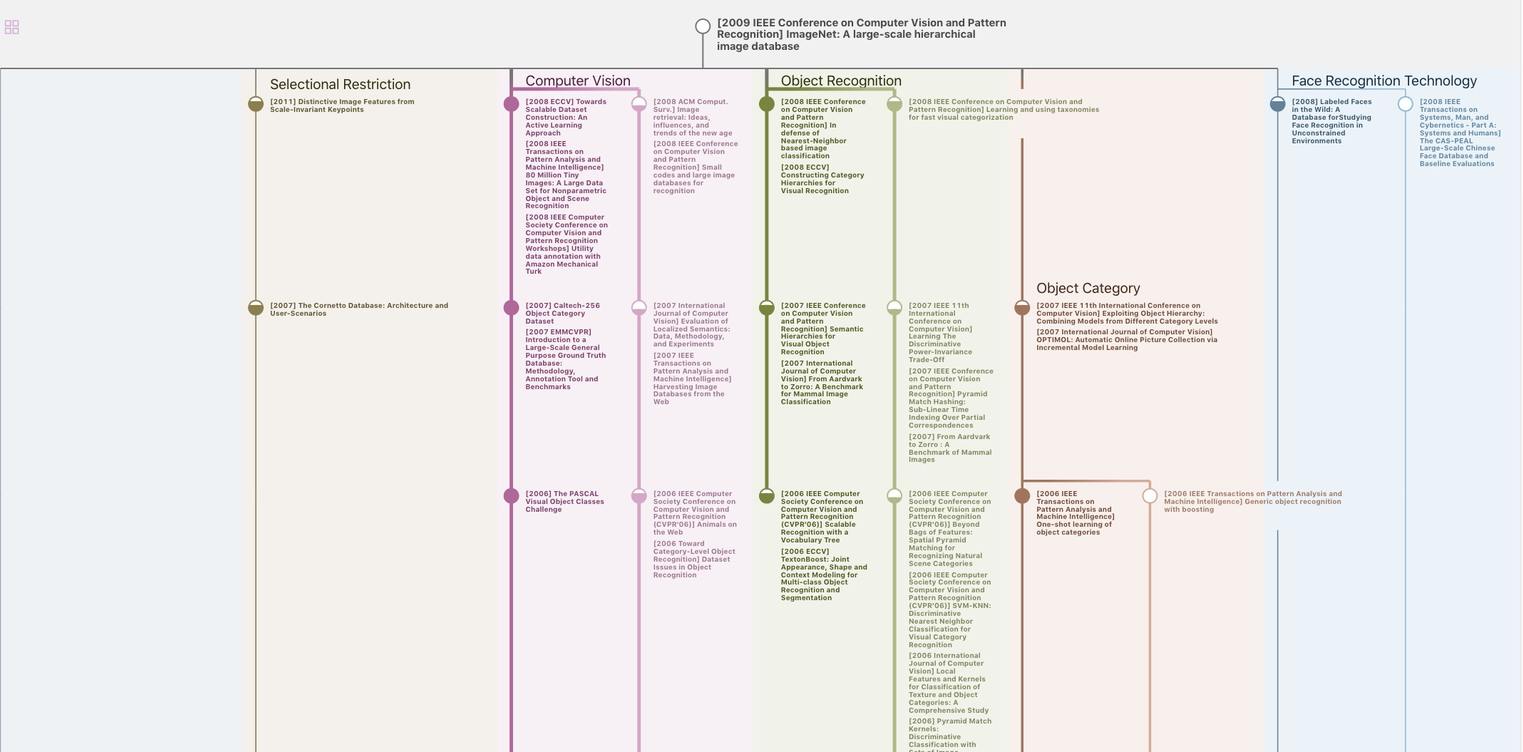
生成溯源树,研究论文发展脉络
Chat Paper
正在生成论文摘要