PMAA: A Progressive Multi-scale Attention Autoencoder Model for High-performance Cloud Removal from Multi-temporal Satellite Imagery
Frontiers in artificial intelligence and applications(2023)
摘要
Satellite imagery analysis plays a pivotal role in remote sensing; however, information loss due to cloud cover significantly impedes its application. Although existing deep cloud removal models have achieved notable outcomes, they scarcely consider contextual information. This study introduces a high-performance cloud removal architecture, termed Progressive Multi-scale Attention Autoencoder (PMAA), which concurrently harnesses global and local information to construct robust contextual dependencies using a novel Multi-scale Attention Module (MAM) and a novel Local Interaction Module (LIM). PMAA establishes long-range dependencies of multi-scale features using MAM and modulates the reconstruction of fine-grained details utilizing LIM, enabling simultaneous representation of fine- and coarse-grained features at the same level. With the help of diverse and multi-scale features, PMAA consistently outperforms the previous state-of-the-art model CTGAN on two benchmark datasets. Moreover, PMAA boasts considerable efficiency advantages, with only 0.5% and 14.6% of the parameters and computational complexity of CTGAN, respectively. These comprehensive results underscore PMAA's potential as a lightweight cloud removal network suitable for deployment on edge devices to accomplish large-scale cloud removal tasks. Our source code and pre-trained models are available at https://github.com/XavierJiezou/PMAA.
更多查看译文
关键词
attention,cloud,imagery,pmaa,multi-scale,high-performance,multi-temporal
AI 理解论文
溯源树
样例
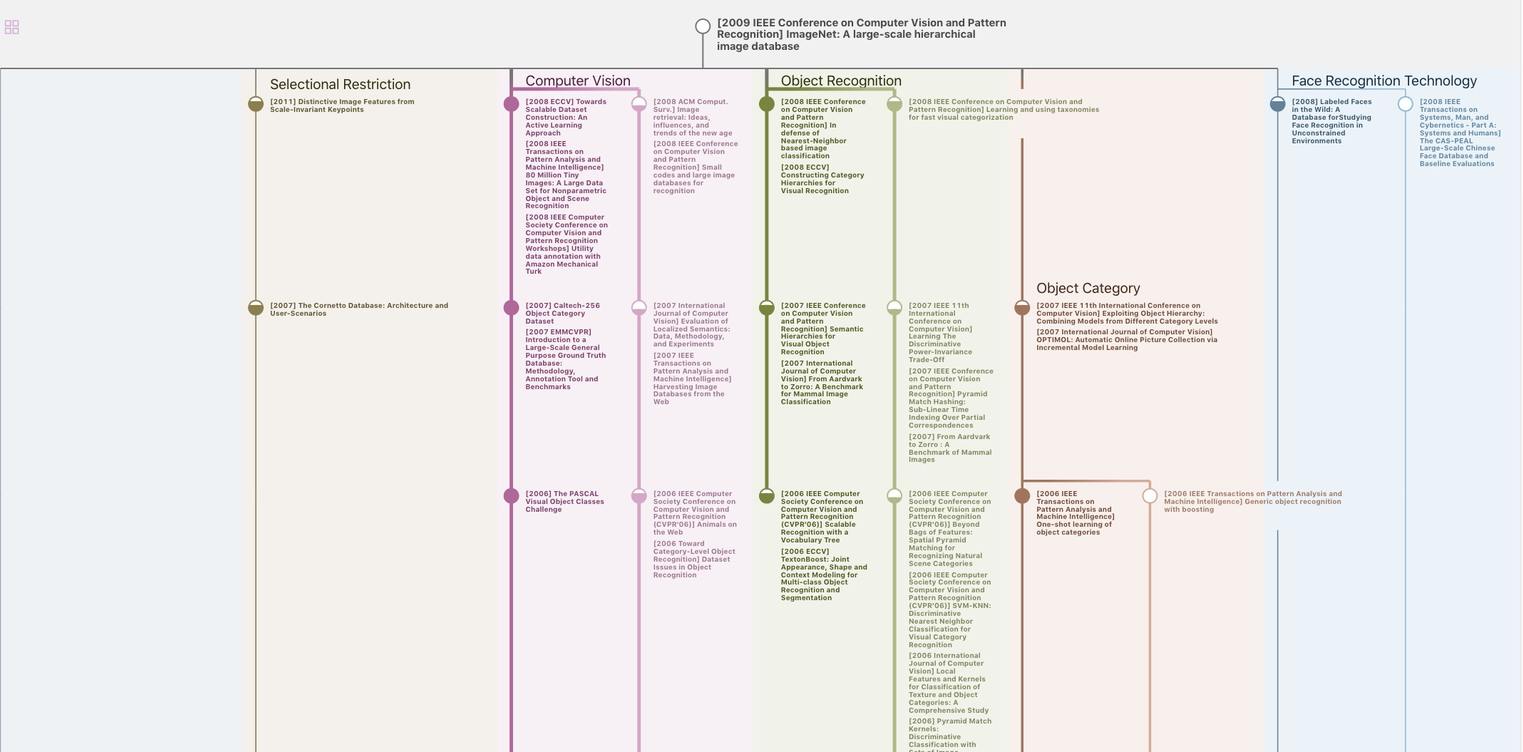
生成溯源树,研究论文发展脉络
Chat Paper
正在生成论文摘要